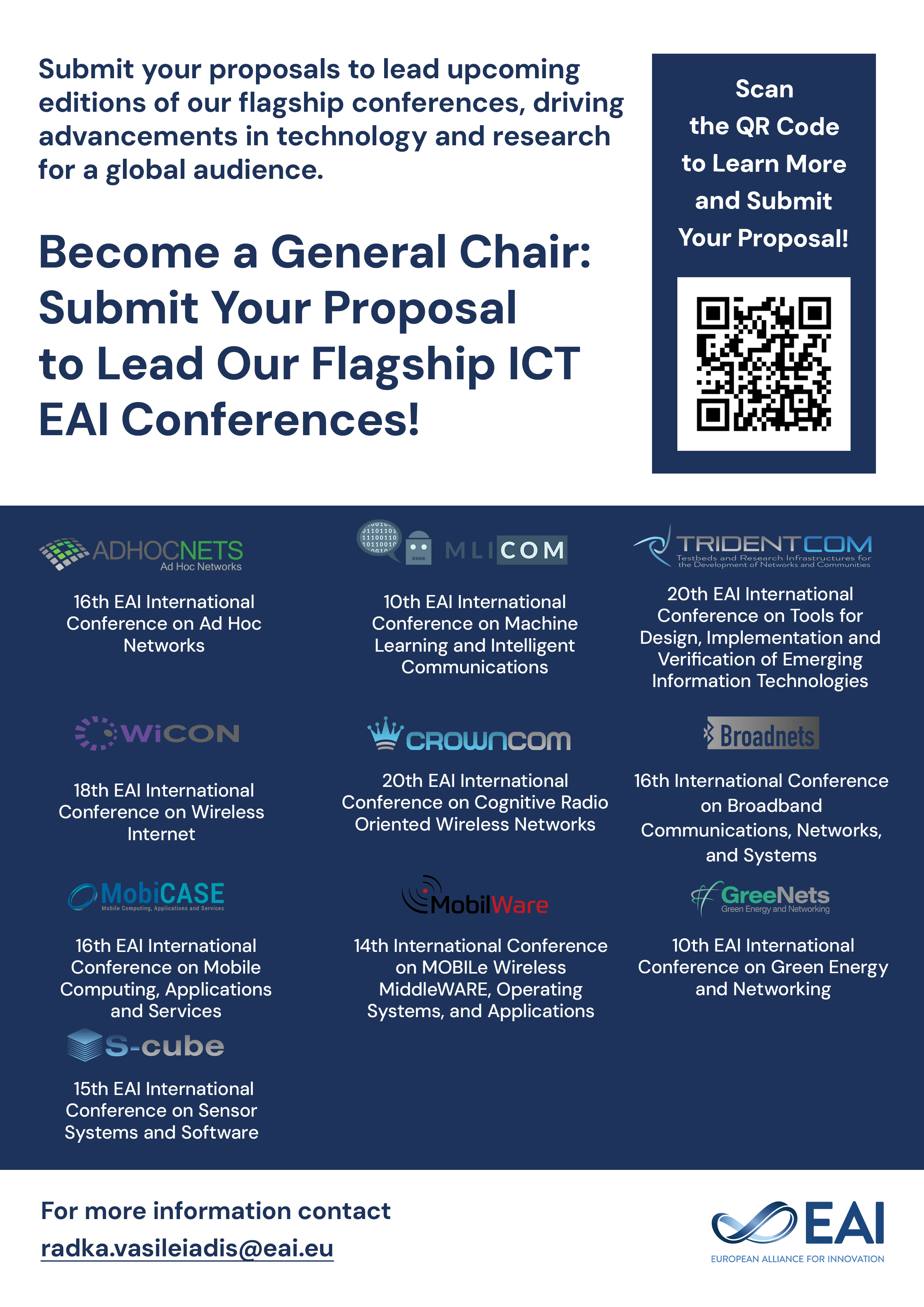
Research Article
Stock Prediction Based on LSTM
@INPROCEEDINGS{10.4108/eai.8-12-2023.2344733, author={Min Li and Weize Liao and Jianrong Huang and Jiebo Jiang}, title={Stock Prediction Based on LSTM}, proceedings={Proceedings of the 5th Management Science Informatization and Economic Innovation Development Conference, MSIEID 2023, December 8--10, 2023, Guangzhou, China}, publisher={EAI}, proceedings_a={MSIEID}, year={2024}, month={4}, keywords={stock; lstm; prediction; multivariate}, doi={10.4108/eai.8-12-2023.2344733} }
- Min Li
Weize Liao
Jianrong Huang
Jiebo Jiang
Year: 2024
Stock Prediction Based on LSTM
MSIEID
EAI
DOI: 10.4108/eai.8-12-2023.2344733
Abstract
Stock market forecasting based on Long Short-Term Memory networks (LSTMs) is a prevalent technique within the realms of Natural Language Processing and time-series analysis. Predicting stock market trends is widely acknowledged to be a highly intricate task, attributed to the multifaceted influences ranging from economic factors, policy changes, to market sentiment. The LSTM's superior capability in handling time-series data has thus made it a popular choice for such forecasting endeavors. This paper selects two stocks, Vanadium Titanium Shares (SZ.000629) and Crystal Technology (SH.603005), and applies both single variable LSTM(SV-LSTM) and multivariate LSTM(MV-LSTM) models to forecast the opening price, the lowest price, and the previous closing price. The results indicate that the MV-LSTM yields more accurate predictions and exhibits relatively smaller errors than its SV-LSTM counterpart.