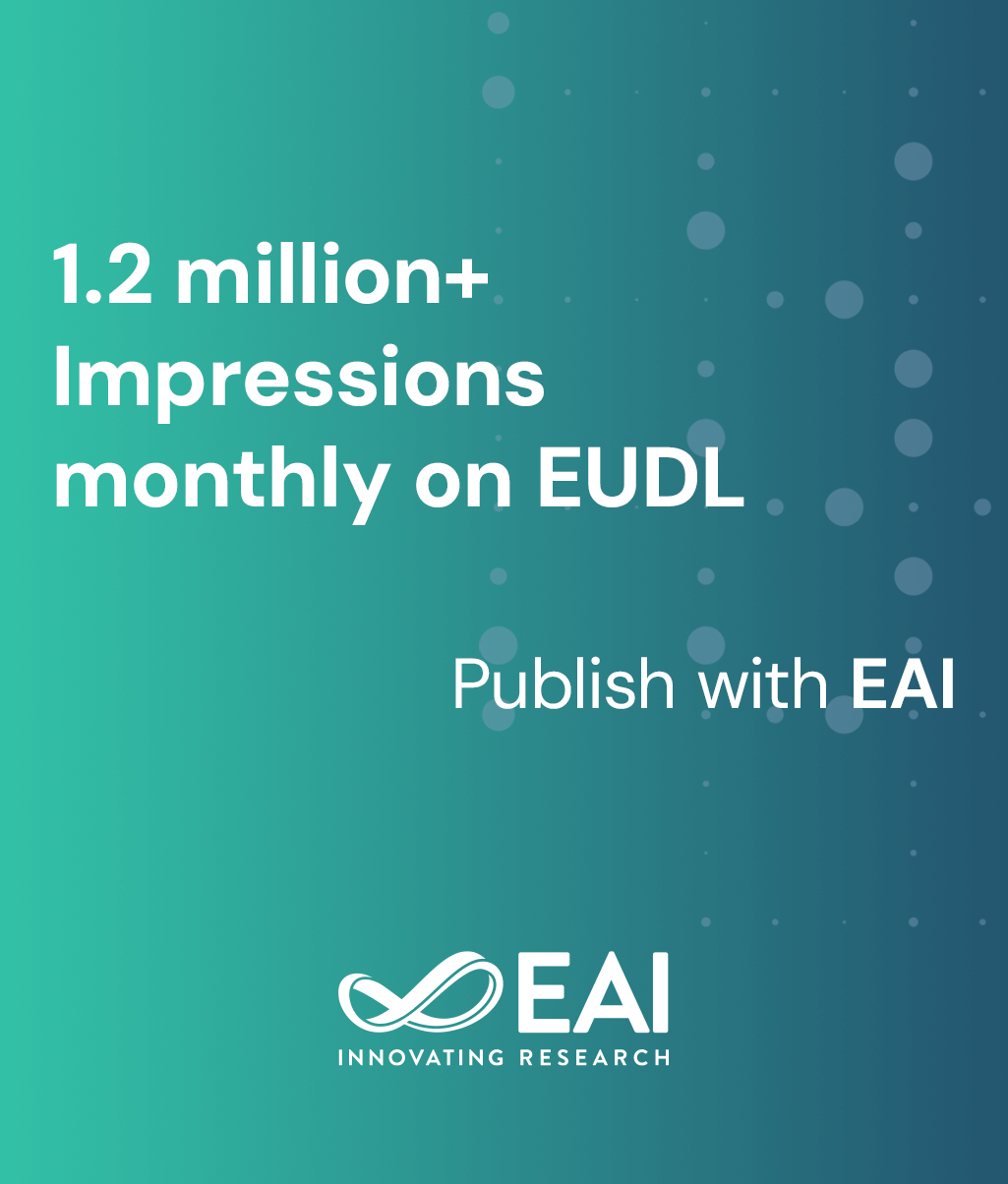
Research Article
Enhancing Predictive Accuracy in Financial Machine Learning Through Gaussian Mixture Decomposition
@INPROCEEDINGS{10.4108/eai.8-12-2023.2344476, author={Xingyou Li and Bo Yu}, title={Enhancing Predictive Accuracy in Financial Machine Learning Through Gaussian Mixture Decomposition}, proceedings={Proceedings of the 5th Management Science Informatization and Economic Innovation Development Conference, MSIEID 2023, December 8--10, 2023, Guangzhou, China}, publisher={EAI}, proceedings_a={MSIEID}, year={2024}, month={4}, keywords={gaussian mixture distribution cnn svm attention}, doi={10.4108/eai.8-12-2023.2344476} }
- Xingyou Li
Bo Yu
Year: 2024
Enhancing Predictive Accuracy in Financial Machine Learning Through Gaussian Mixture Decomposition
MSIEID
EAI
DOI: 10.4108/eai.8-12-2023.2344476
Abstract
When machine learning is applied to financial markets, one of the persistent challenges is improving the accuracy of model predictions due to the presence of substantial noise in financial data. This study aims to enhance data quality through a novel approach: decomposing data into distinct groups using Gaussian mixture distributions and independently training machine learning models on each group. Three different models were empirically validated: Convolutional Neural Networks, Support Vector Machines, and Attention Models. The results indicate that this method significantly enhances the predictive accuracy of the models. In addition, a majority voting approach was employed to select prediction results, demonstrating promising results in terms of accuracy and enhancing the practical utility of the method. The efficacy of this approach lies in separating various influencing factors in the data, making relatively simple data distributions more conducive to training machine learning models. This research provides new insights for modeling stock markets using machine learning, offering a means to mitigate the adverse impact of data noise on predictive performance and improving prediction accuracy.