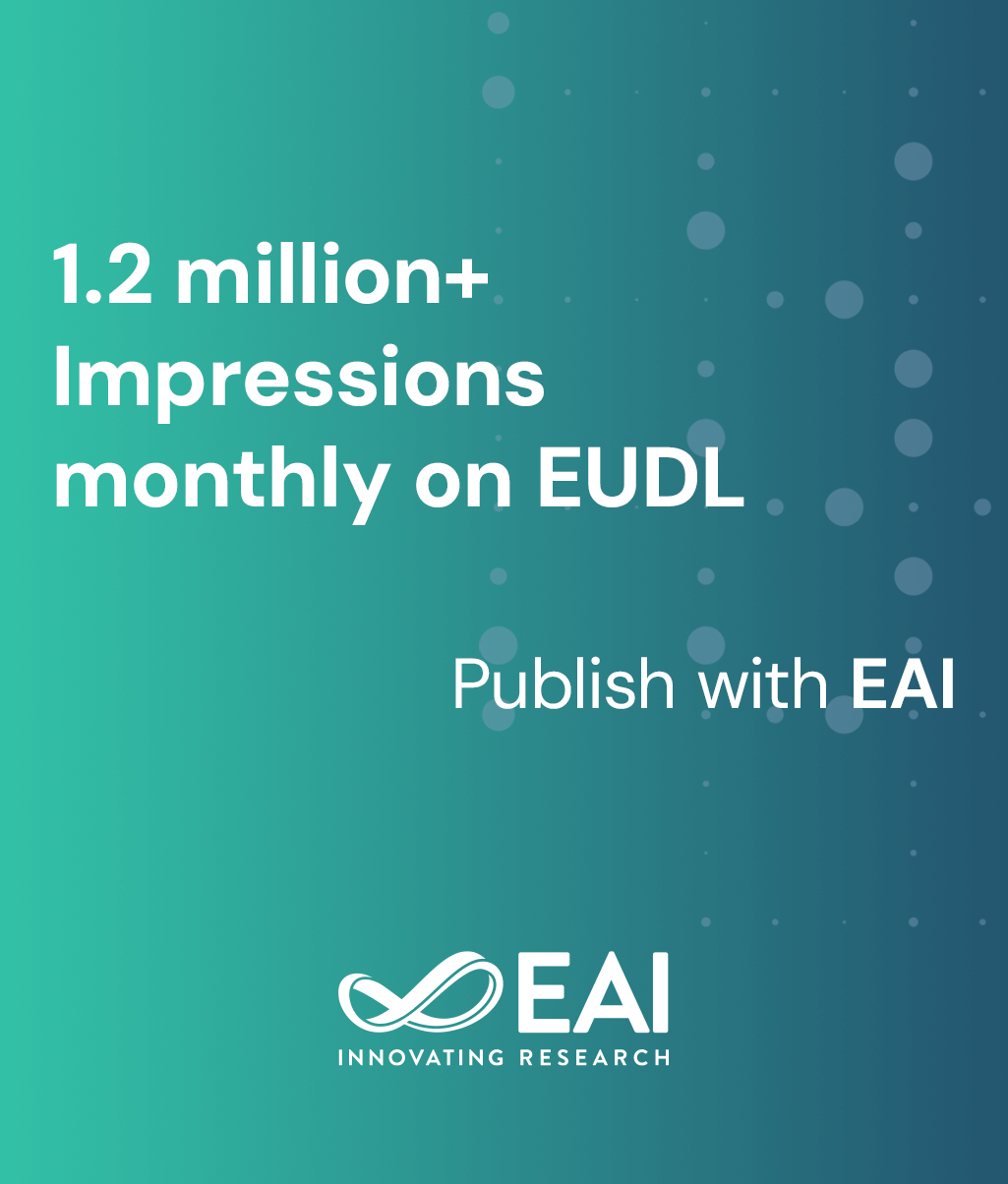
Research Article
Identifying forensically uninteresting files in a large corpus
@ARTICLE{10.4108/eai.8-12-2016.151725, author={N. C. Rowe}, title={Identifying forensically uninteresting files in a large corpus}, journal={EAI Endorsed Transactions on Security and Safety}, volume={3}, number={7}, publisher={EAI}, journal_a={SESA}, year={2016}, month={12}, keywords={digital forensics, metadata, files, corpus, data reduction, hashes, triage, whitelists, classification, malware, camouflage.}, doi={10.4108/eai.8-12-2016.151725} }
- N. C. Rowe
Year: 2016
Identifying forensically uninteresting files in a large corpus
SESA
EAI
DOI: 10.4108/eai.8-12-2016.151725
Abstract
For digital forensics, eliminating the uninteresting is often more critical than finding the interesting. We discuss methods exploiting the metadata of a large corpus. Tests were done with an international corpus of 262.7 million files obtained from 4018 drives. For malware investigations, we show that using a Bayesian ranking formula on metadata can increase malware recall by 5.1 while increasing precision by 1.7 times over inspecting executables alone. For more general investigations, we show that requiring two of nine criteria for uninteresting files, with exceptions for some special interesting files, can exclude 77.4% of our corpus. For a test set that was manually inspected, interesting files identified as uninteresting were 0.18% and uninteresting files identified as interesting were 29.31%. The generality of the methods was confirmed by separately testing two halves of our corpus. This work provides both new uninteresting hash values and programs for finding more.
Copyright © 2016 N. C. Rowe, licensed to EAI. This is an open access article distributed under the terms of the Creative Commons Attribution licence (http://creativecommons.org/licenses/by/3.0/), which permits unlimited use, distribution and reproduction in any medium so long as the original work is properly cited.