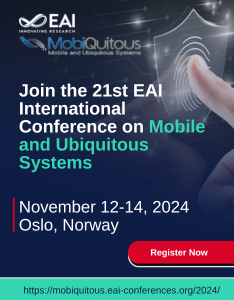
Research Article
Beating-Time Gestures Imitation Learning for Humanoid Robots
@ARTICLE{10.4108/eai.8-11-2017.153335, author={Denis Amelynck and Pieter-Jan Maes and Jean-Pierre Martens and Marc Leman}, title={Beating-Time Gestures Imitation Learning for Humanoid Robots}, journal={EAI Endorsed Transactions on Creative Technologies}, volume={4}, number={13}, publisher={EAI}, journal_a={CT}, year={2017}, month={10}, keywords={programming by demonstration, cubic spline regression, dynamical time warping, beating-time gestures}, doi={10.4108/eai.8-11-2017.153335} }
- Denis Amelynck
Pieter-Jan Maes
Jean-Pierre Martens
Marc Leman
Year: 2017
Beating-Time Gestures Imitation Learning for Humanoid Robots
CT
EAI
DOI: 10.4108/eai.8-11-2017.153335
Abstract
Beating-time gestures are movement patterns of the hand swaying along with music, thereby indicating accented musical pulses. The spatiotemporal configuration of these patterns makes it diÿcult to analyse and model them. In this paper we present an innovative modelling approach that is based upon imitation learning or Programming by Demonstration (PbD). Our approach - based on Dirichlet Process Mixture Models, Hidden Markov Models, Dynamic Time Warping, and non-uniform cubic spline regression - is particularly innovative as it handles spatial and temporal variability by the generation of a generalised trajectory from a set of periodically repeated movements. Although not within the scope of our study, our procedures may be implemented for the sake of controlling movement behaviour of robots and avatar animations in response to music.
Copyright © 2017 Denis Amelynck et al., licensed to EAI. This is an open access article distributed under the terms of the Creative Commons Attribution license (http://creativecommons.org/licenses/by/3.0/), which permits unlimited use, distribution and reproduction in any medium so long as the original work is properly cited.