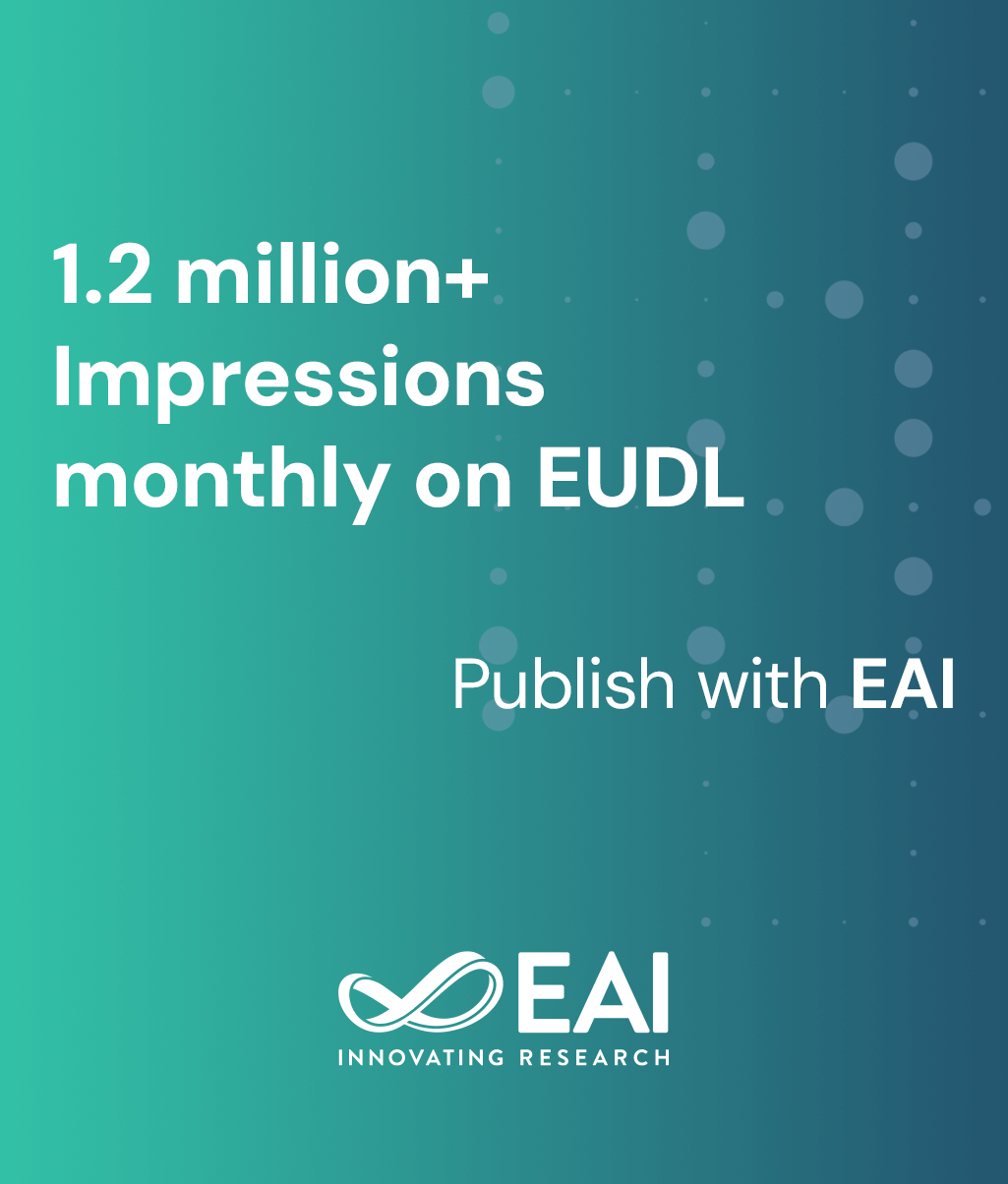
Research Article
Box-Jenkins Modelling and Forecasting of WTI Crude Oil Price
@INPROCEEDINGS{10.4108/eai.7-9-2021.2314841, author={Maitham A. Rodhan and Adnan H. Jaaz}, title={Box-Jenkins Modelling and Forecasting of WTI Crude Oil Price}, proceedings={Proceedings of 2nd International Multi-Disciplinary Conference Theme: Integrated Sciences and Technologies, IMDC-IST 2021, 7-9 September 2021, Sakarya, Turkey}, publisher={EAI}, proceedings_a={IMDC-IST}, year={2022}, month={1}, keywords={wti crude oil arima model stationarity oil price forecast jel codes: c22 c51 e37 p28 g12}, doi={10.4108/eai.7-9-2021.2314841} }
- Maitham A. Rodhan
Adnan H. Jaaz
Year: 2022
Box-Jenkins Modelling and Forecasting of WTI Crude Oil Price
IMDC-IST
EAI
DOI: 10.4108/eai.7-9-2021.2314841
Abstract
Oil is one of the most important commodities that its price and volatility have a huge impact on everyone´s life. Oil heavily affects economic growth. Unexpected movements in oil price have effects on economic stability for both supplier and producer countries. So, estimating crude oil prices are very vital. There are many methods used to model and forecast time-series data such as trend, regression, moving average, and so on. In this paper, the ARIMA method is considered to predict the upcoming year. ARIMA techniques are used to analyse time-series data and have been mainly used for loading forecast due to its accuracy, mathematical soundness, and flexibility due to the inclusion of AR and MA terms over a regression analysis. Data of WTI price obtained from US energy information administration (EIA) from March 1990 to March 2021. The data is first differenced for stationarity and autocorrelation and the residuals techniques are used to select different ARIMA models for analysis. The performance of different models was compared, and the results show that ARIMA (1,1,4) model was the best forecasting. A detailed explanation of the above is presented and summarized in the tables and figures below.