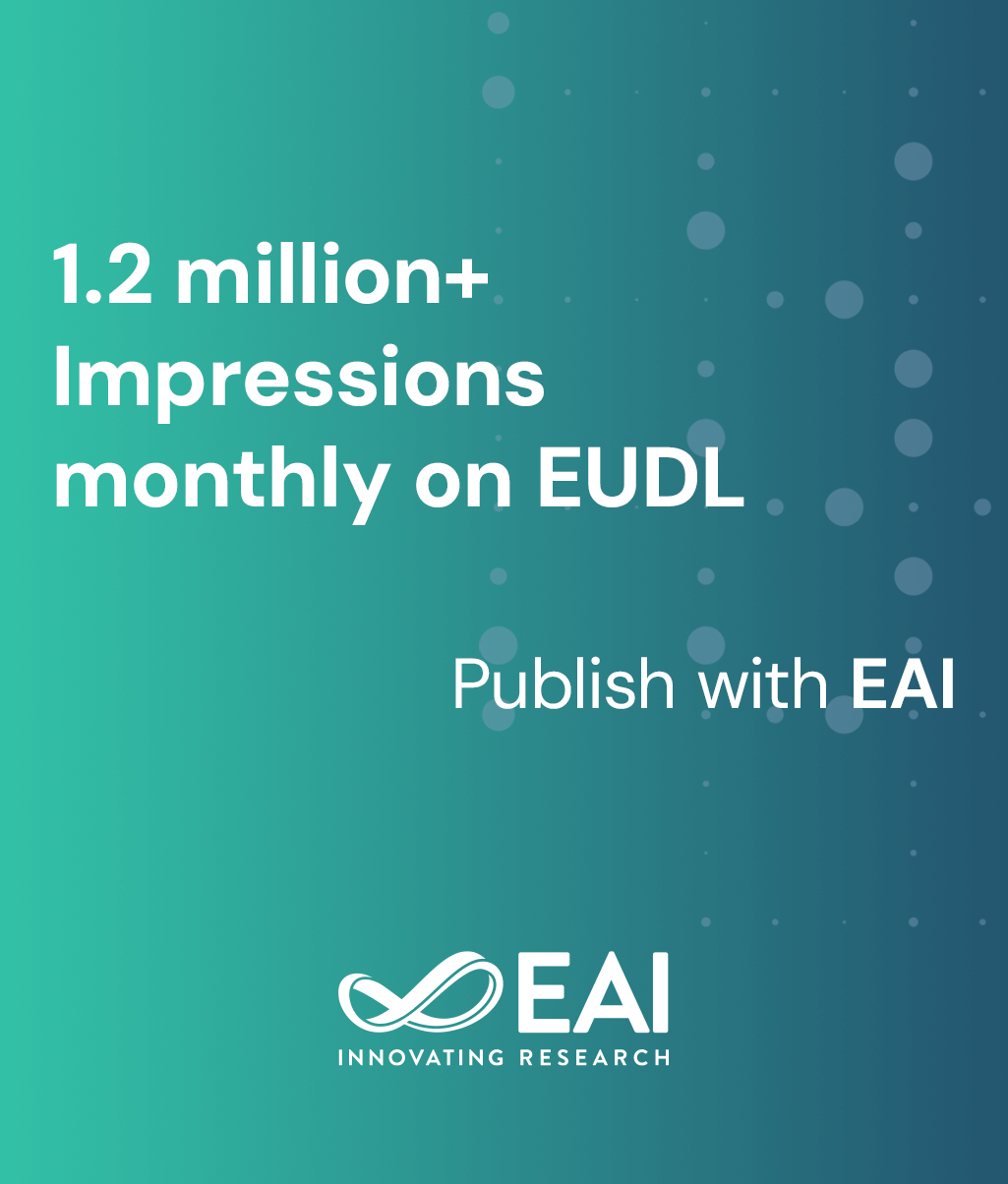
Research Article
Diagnosing False Data Injection Attacks in the Smart Grid: a Practical Framework for Home-area Networks
@INPROCEEDINGS{10.4108/eai.7-8-2017.152988, author={Adrian Padin and Yeabsera Kebede and Maxwell Morgan and Davis Vorva and Atman Fozdar and Richard Kalvaitis and Nikolas Remley and Samir Tout and Michael G. Kallitsis}, title={Diagnosing False Data Injection Attacks in the Smart Grid: a Practical Framework for Home-area Networks}, proceedings={The 1st EAI International Conference on Smart Grid Assisted Internet of Things}, publisher={EAI}, proceedings_a={SGIOT}, year={2017}, month={8}, keywords={Smart grid anomaly detection false data injection attacks statistics algorithms software monitoring real-world measurements.}, doi={10.4108/eai.7-8-2017.152988} }
- Adrian Padin
Yeabsera Kebede
Maxwell Morgan
Davis Vorva
Atman Fozdar
Richard Kalvaitis
Nikolas Remley
Samir Tout
Michael G. Kallitsis
Year: 2017
Diagnosing False Data Injection Attacks in the Smart Grid: a Practical Framework for Home-area Networks
SGIOT
EAI
DOI: 10.4108/eai.7-8-2017.152988
Abstract
Advances in the metering infrastructure of the electric grid allow two-way communication capabilities between the utility center and a vast array of smart meters installed in the grid's distribution and transmission components. Nefarious users that manage to compromise insecure smart meters can alter the payload transmitted from these meters, and abruptly increase or reduce electricity demand in a coordinated manner. This malicious practice, known as false data injection attack, can destabilize the grid. This paper describes a practical framework for diagnosing false data injection attacks in the smart grid. We propose a behavioral-based monitoring system that can be installed at home-area networks for detecting the aforementioned anomalies. We demonstrate a real-world prototype of our system engineered with inexpensive devices such as Raspberry Pi's and Z-Wave wireless sensors, and evaluate its performance with real data.