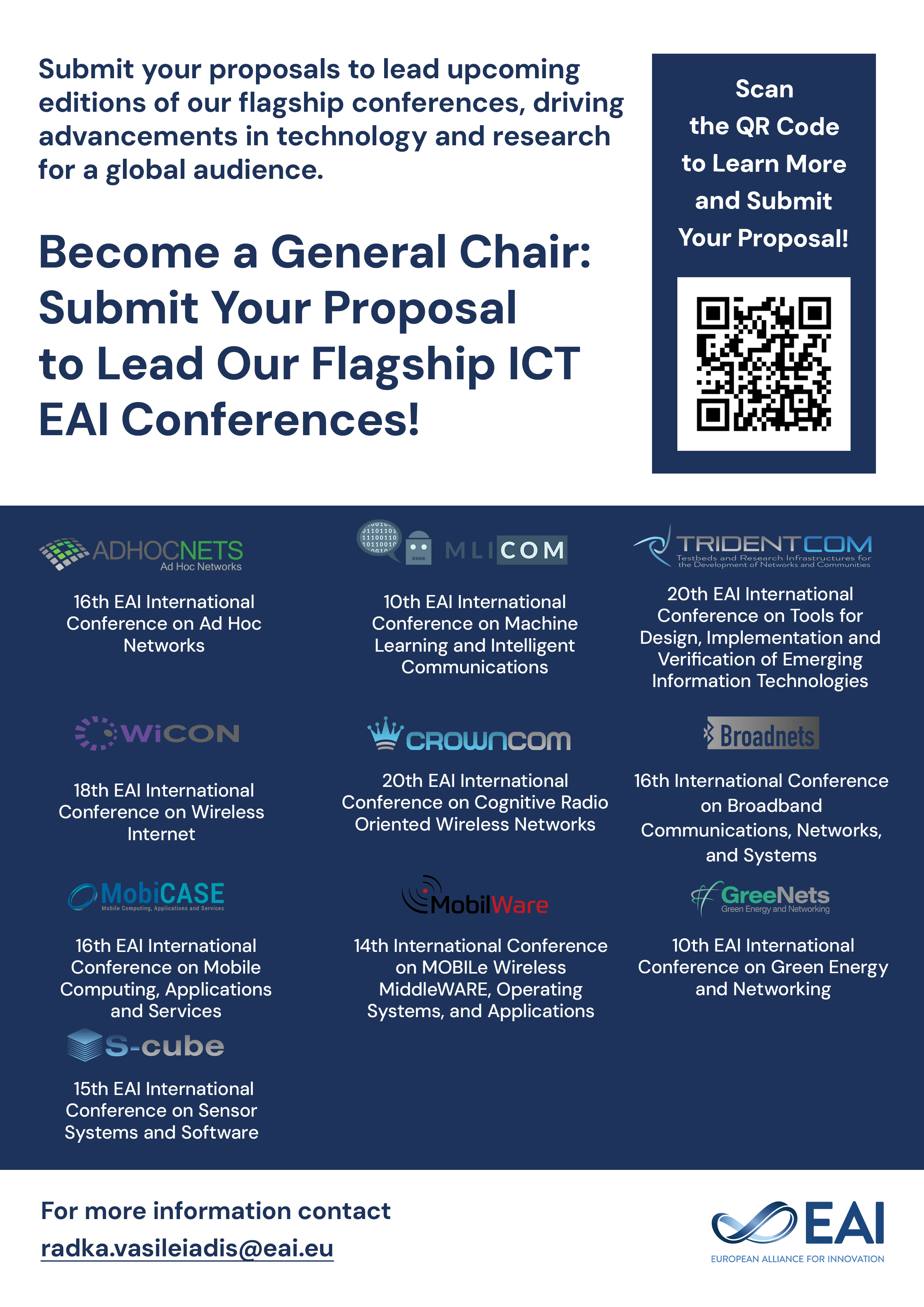
Research Article
Research on the Application of Deep Learning-based Differential Privacy Protection Models in Financial Big Data
@INPROCEEDINGS{10.4108/eai.7-7-2023.2338057, author={Yanjun Ma and Rui Lu and Yu Zhang}, title={Research on the Application of Deep Learning-based Differential Privacy Protection Models in Financial Big Data}, proceedings={Proceedings of the 2nd International Conference on Financial Innovation, FinTech and Information Technology, FFIT 2023, July 7--9, 2023, Chongqing, China}, publisher={EAI}, proceedings_a={FFIT}, year={2023}, month={10}, keywords={deep learning differential privacy privacy protection financial big data}, doi={10.4108/eai.7-7-2023.2338057} }
- Yanjun Ma
Rui Lu
Yu Zhang
Year: 2023
Research on the Application of Deep Learning-based Differential Privacy Protection Models in Financial Big Data
FFIT
EAI
DOI: 10.4108/eai.7-7-2023.2338057
Abstract
With the digital transformation of the financial industry and the widespread application of big data, privacy protection has become a critical challenge. The differential privacy protection model based on deep learning preserves the privacy of financial big data while maintaining data availability and accuracy. This paper investigates and analyses the application of differential privacy protection models based on deep learning in the field of financial big data. Firstly, this paper introduces the basic concepts and principles of differential privacy, as well as the significance of deep learning in the financial domain. Next, the working principles and main methods of the differential privacy protection model based on deep learning are elaborated, including privacy budget allocation, noise injection, and model optimization techniques. Finally, this paper explores the application of the differential privacy protection model based on deep learning in financial big data analysis. It is noted that these models can achieve efficient and accurate data analysis and prediction while protecting user privacy. Specific applications include individual credit assessment, fraud detection, anti-fraud measures, among others. The paper also discusses the advantages and potential challenges of the models and proposes future research directions.