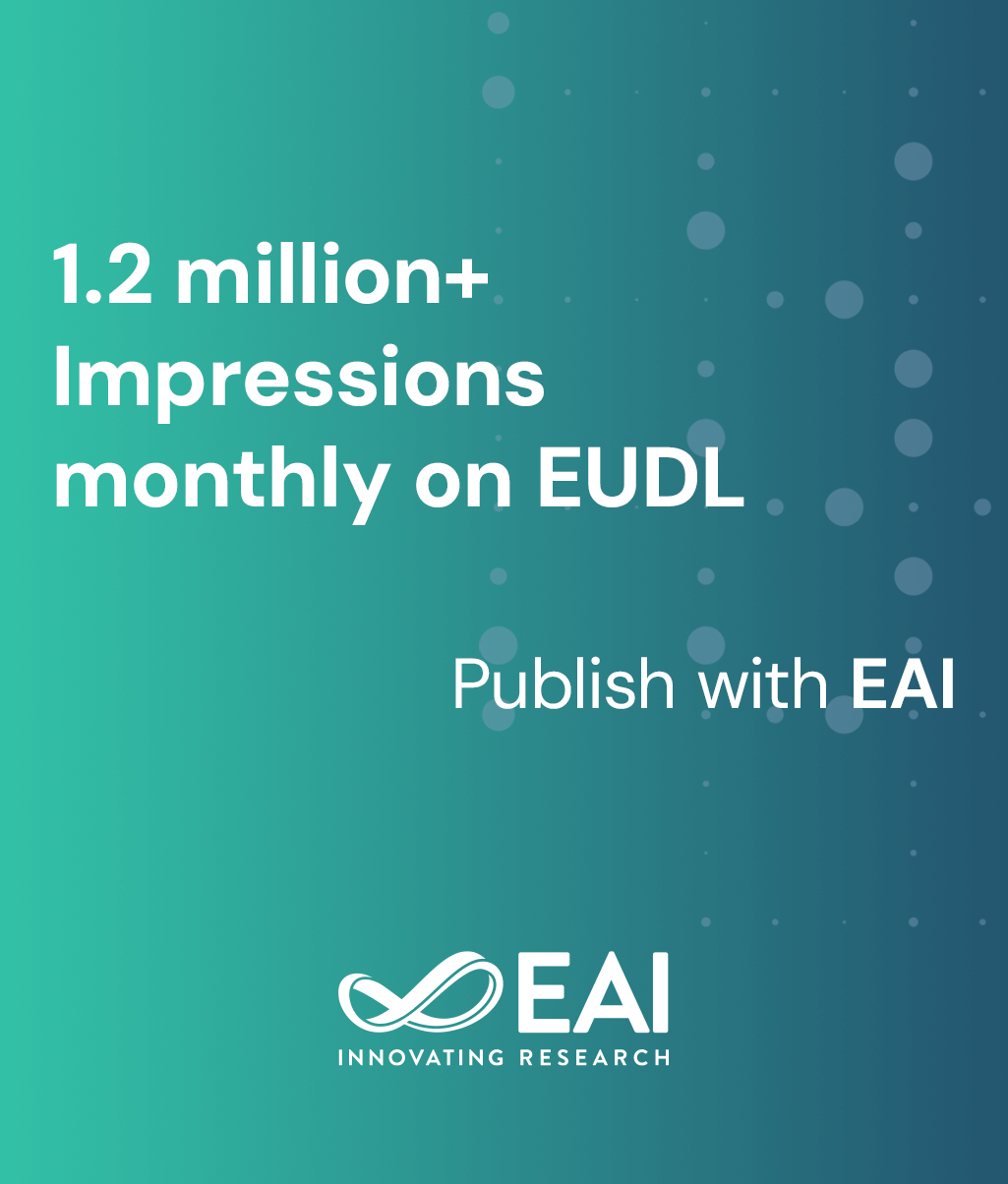
Research Article
Analysis and Discrimination of Insurance Fraud based on Data Mining
@INPROCEEDINGS{10.4108/eai.7-7-2023.2338047, author={Tianqi Yang and Yue Wu}, title={Analysis and Discrimination of Insurance Fraud based on Data Mining}, proceedings={Proceedings of the 2nd International Conference on Financial Innovation, FinTech and Information Technology, FFIT 2023, July 7--9, 2023, Chongqing, China}, publisher={EAI}, proceedings_a={FFIT}, year={2023}, month={10}, keywords={insurance fraud data mining k-means svm}, doi={10.4108/eai.7-7-2023.2338047} }
- Tianqi Yang
Yue Wu
Year: 2023
Analysis and Discrimination of Insurance Fraud based on Data Mining
FFIT
EAI
DOI: 10.4108/eai.7-7-2023.2338047
Abstract
Insurance is an important component of the financial system, playing an important role in social stability and ensuring people's livelihoods. With the vigorous develop-ment of the insurance industry this year, automobile insurance fraud has been a fre-quent occurrence. This article achieves effective discrimination of insurance fraud by constructing a model of insurance fraud. Firstly, preprocess the data from the insur-ance claims dataset and encode the text variables using average encoding; Then, this article conducts data correlation analysis on all attributes of the sample using Pearson correlation coefficient; Based on the results of the previous analysis, the K-means clustering method is used to achieve dimensionality reduction and enhancement of sample attributes; Finally, by training an SVM classifier with Gaussian kernel func-tion, effective discrimination of insurance fraud is achieved. Through experimental verification, the model method is effective and can achieve accurate discrimination with an accuracy of 96%.