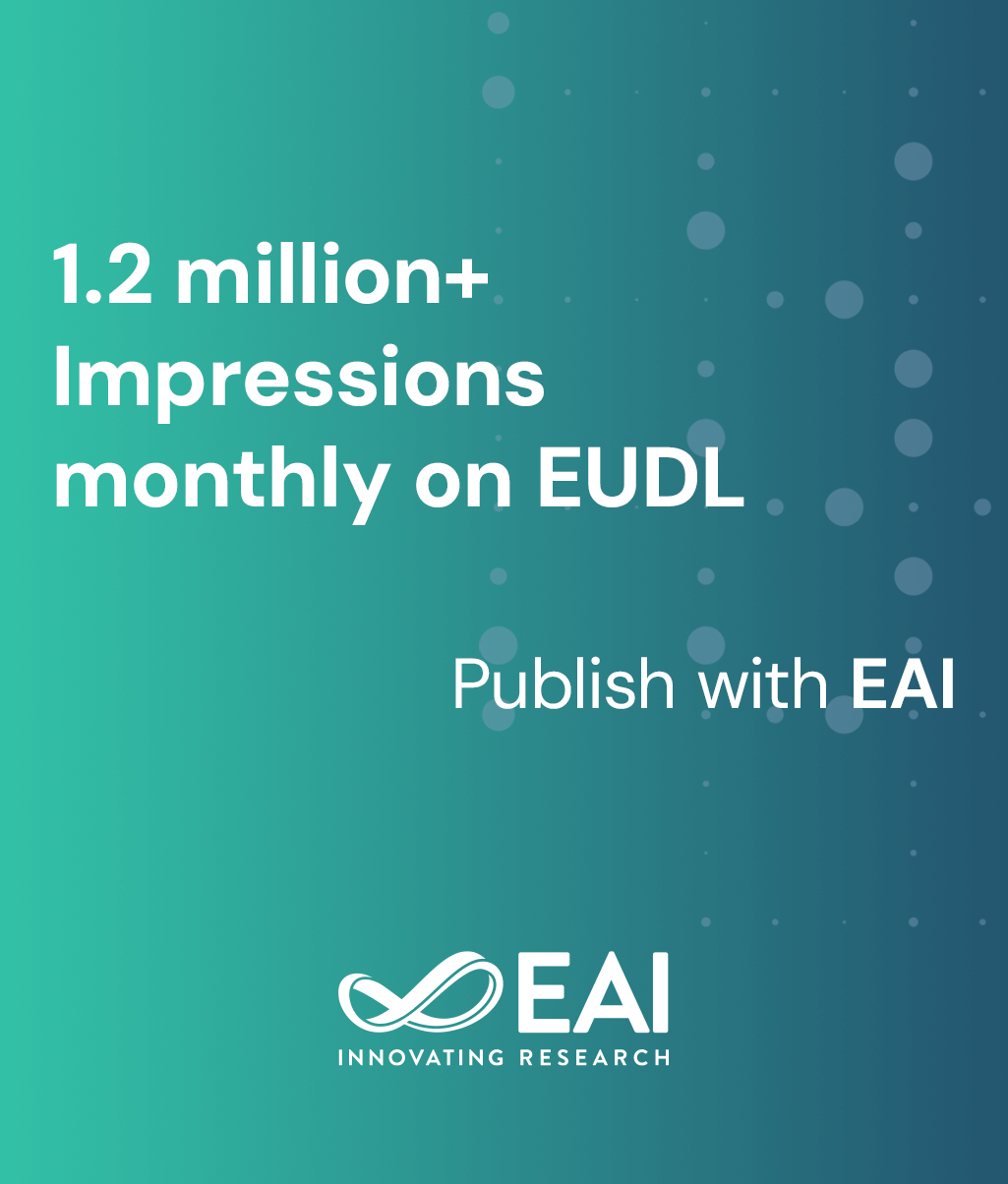
Research Article
Botnet Detection Based On Network Traffic Flow Statistical Features and Model Based Clustering
@INPROCEEDINGS{10.4108/eai.7-12-2021.2314962, author={Kirubavathi G and Nalini S}, title={Botnet Detection Based On Network Traffic Flow Statistical Features and Model Based Clustering}, proceedings={Proceedings of the First International Conference on Combinatorial and Optimization, ICCAP 2021, December 7-8 2021, Chennai, India}, publisher={EAI}, proceedings_a={ICCAP}, year={2021}, month={12}, keywords={botnet detection; network flows; statistical features; model based clustering}, doi={10.4108/eai.7-12-2021.2314962} }
- Kirubavathi G
Nalini S
Year: 2021
Botnet Detection Based On Network Traffic Flow Statistical Features and Model Based Clustering
ICCAP
EAI
DOI: 10.4108/eai.7-12-2021.2314962
Abstract
Botnet is one of the most notorious threats to cybersecurity and cyberspace, providing a distributed platform for multiple illegal activities, such as DDoS, spamming, phishing, click fraud, identity theft, etc. Regardless of numerous methods have been proposed to detect botnets, botnet detection is still a challenging issue, as botmaster’s are continuously improving bots to write them stealthier. Existing botnet detection mechanisms are not cope-up with the modern botnets. In this paper, we propose a novel approach to detect botnet based on network traffic flow behavior analysis using model based clustering called Gaussian Mixture Model (GMM). We have analyzed the botnet traffic flow statistical behaviors in a mananged environment. The proposed model effectively detects the bot irrespective of their structural properties. Our experimental evaluation based on real-world data shows that the proposed model can achieve high detection accuracy with a low false positive rate using traffic flow behaviors. We have compared the proposed model with traditional clustering techniques such as K-Means and X-Means clustering. Our model achieves the improved detection rate compared to the K-Means and X-Means clustering. Also we have compared our proposed model with existing botnet detection methods. Our model achieves the better detection rate with minimum number of features than the prevailing methods.