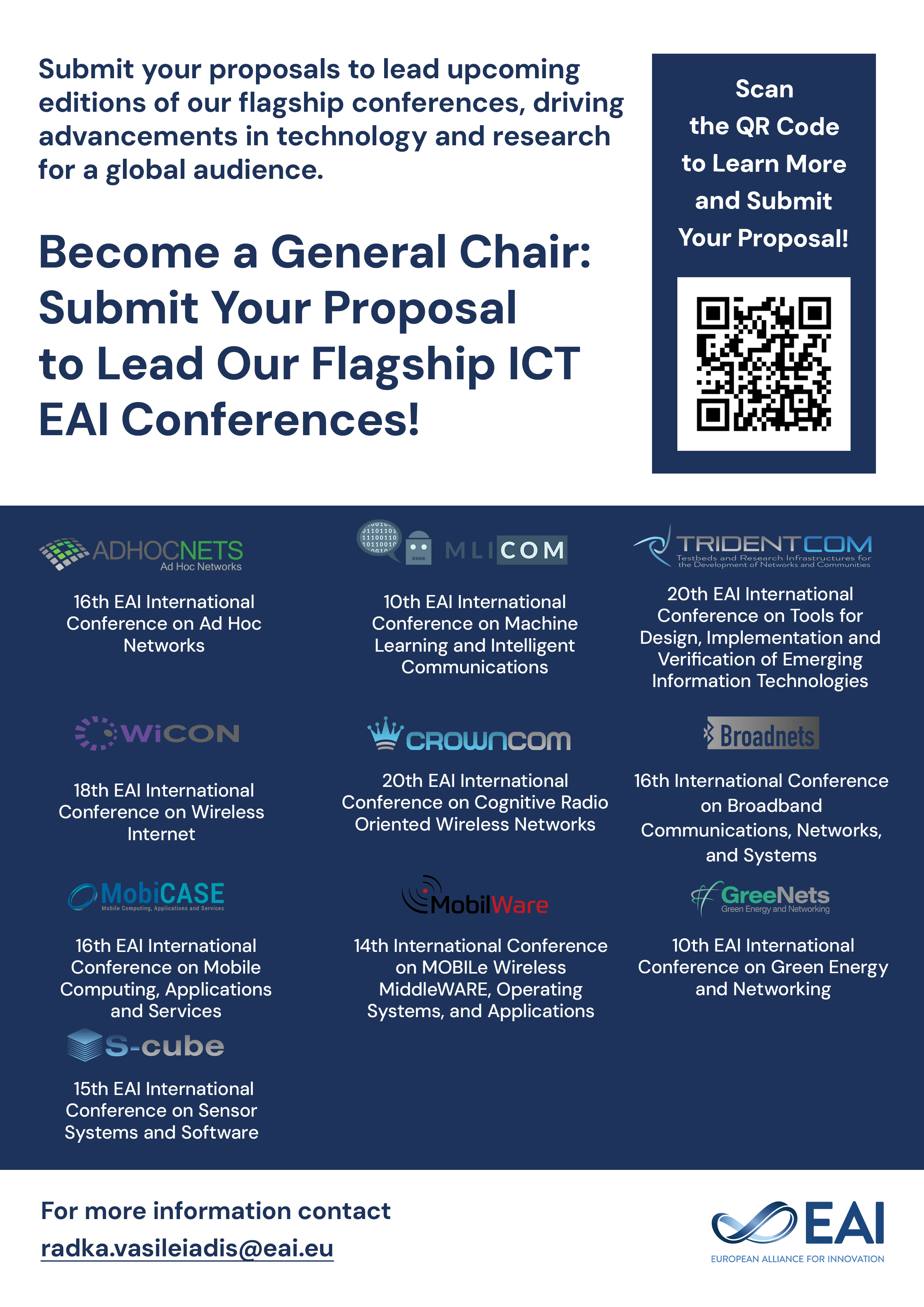
Research Article
Supervised machine leaning based anomaly detection and diagnosis in grid connected photovoltaic systems
@INPROCEEDINGS{10.4108/eai.7-12-2021.2314754, author={Swetha R Kumar and Phavithraa Devi B and Gayathri R G}, title={Supervised machine leaning based anomaly detection and diagnosis in grid connected photovoltaic systems}, proceedings={Proceedings of the First International Conference on Combinatorial and Optimization, ICCAP 2021, December 7-8 2021, Chennai, India}, publisher={EAI}, proceedings_a={ICCAP}, year={2021}, month={12}, keywords={fault detection pv array system naive bayes one vs rest classifier multi-layer perceptron supervised learning}, doi={10.4108/eai.7-12-2021.2314754} }
- Swetha R Kumar
Phavithraa Devi B
Gayathri R G
Year: 2021
Supervised machine leaning based anomaly detection and diagnosis in grid connected photovoltaic systems
ICCAP
EAI
DOI: 10.4108/eai.7-12-2021.2314754
Abstract
Over the past few decades, due to the slackening in the available non-renewable resources and the paramount of pollutants caused by the consumption of these non- renewable resources, renewable energy has gained huge momentousness in power generation. This enlarged the installation of Solar Power plants. But these Solar based Photovoltaic systems are liable to a huge variety of anomalies which generally deteriorates its performance. If those anomalies were not identified on the dot, this may lead to the reduction in efficiency which makes the PV systems unsubstantial. Hence the diagnosis of the anomaly and the identification of its type is of whopping importance to maintain the ideal function of the PV array system.This paper aims to predict and classify the types of anomalies in Photovoltaic Array systems using supervised machine learning algorithms. The performance of the classifier based on Naive Bayes, One Vs Rest and Multi-layer Perceptron Neural Network model on the provided dataset is tested. The results thus obtained from training and testing the models, are also tabulated and the observations are noted. Simulated results disclosed that the Multi- Layer perceptron-based classifier has the utmost accuracy with minimum mean squared error.