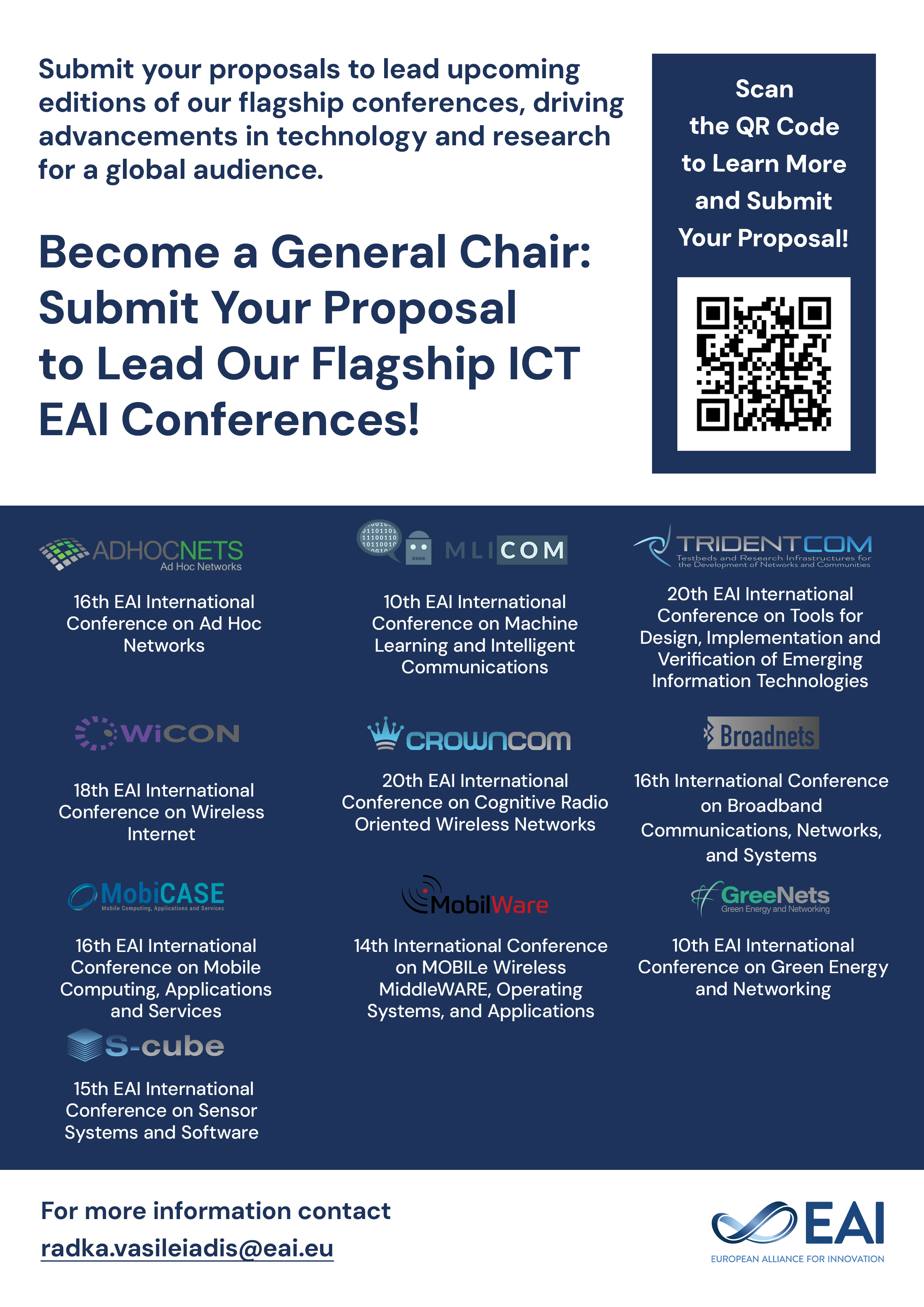
Research Article
Computer Aided Screening Of Cervical Cancer From Pap Smear Microscopic Images
@INPROCEEDINGS{10.4108/eai.7-12-2021.2314705, author={Brindha D and Gauthaam M}, title={Computer Aided Screening Of Cervical Cancer From Pap Smear Microscopic Images}, proceedings={Proceedings of the First International Conference on Combinatorial and Optimization, ICCAP 2021, December 7-8 2021, Chennai, India}, publisher={EAI}, proceedings_a={ICCAP}, year={2021}, month={12}, keywords={hsil lsil segmentation machine learning}, doi={10.4108/eai.7-12-2021.2314705} }
- Brindha D
Gauthaam M
Year: 2021
Computer Aided Screening Of Cervical Cancer From Pap Smear Microscopic Images
ICCAP
EAI
DOI: 10.4108/eai.7-12-2021.2314705
Abstract
Cervical Cancer is globally ranked as the second among the type of cancers found in females. If diagnosed at early stage cervical cancer can be preventable type of cancer. Precancerous cervical cell changes generally do not cause symptoms, and hence it has prompted for an extensive research. An automated system for screening cervical cancer from pap-smear images have been developed to classify the pap smear microscopic image into normal, LSIL(Low Grade Intraepithelial lesion) and HSIL (High Grade Intraepithelial lesion).The proposed methodology concentrates on segmenting the cervical cells along with the removal of neutrophils which found to be greater hindrance for efficient classification of tissues. The proposed segmentation algorithm is highly generic that it could handle overlapped pap-smear cells to a better extent in comparison with other techniques. The morphology and texture based features are extracted from the segmented region of interest and optimized for accurate classification of cervical cells. Performance of classifiers and the significance of each feature have been analyzed from the classification results using machine learning techniques. Among the classifiers, Random Forest classifier provided an overall accuracy of 90% for cervical cancer screening using pap-smear microscopic images.