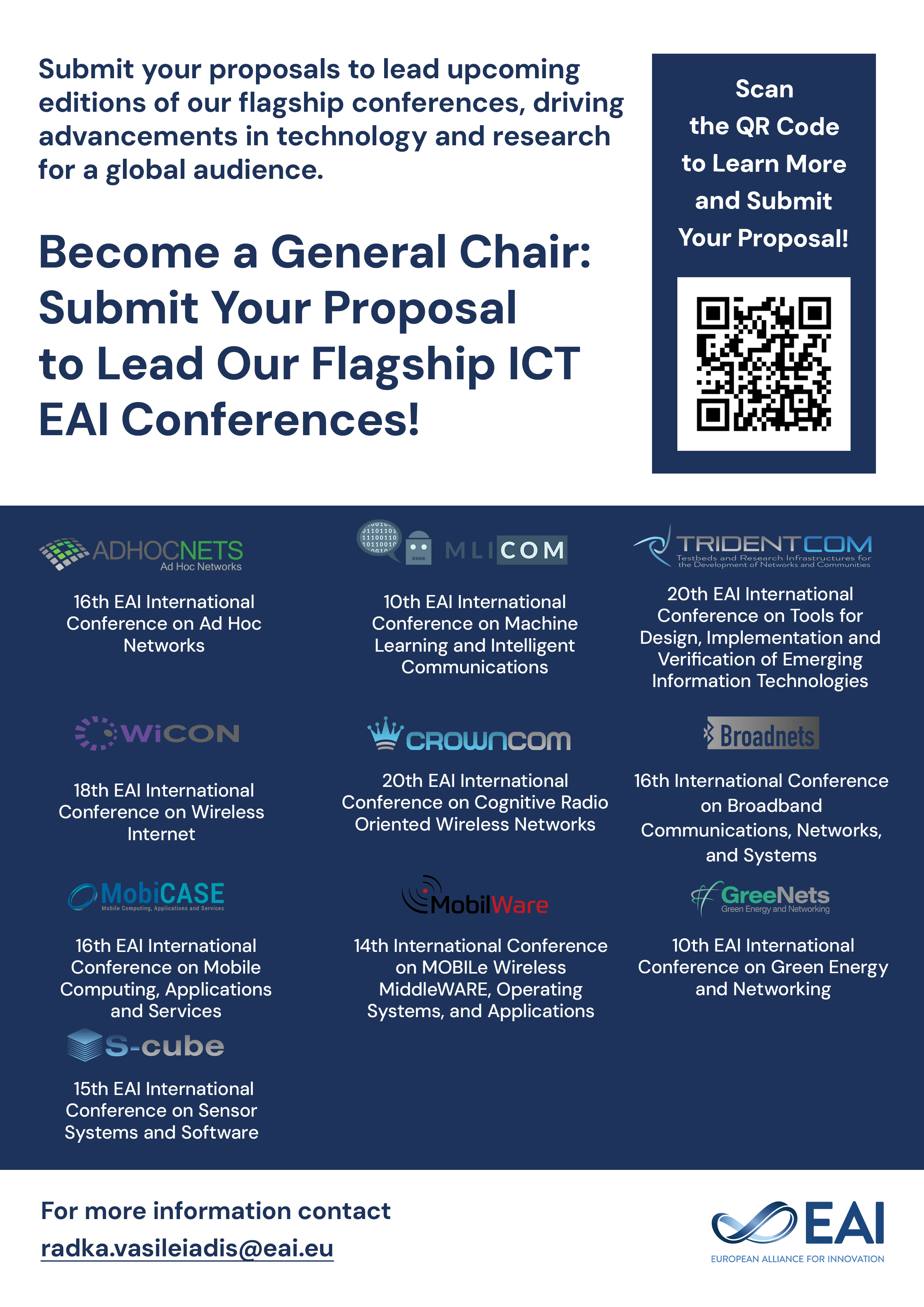
Research Article
Investigation of KNN and Decision Tree Induction Modelin Predicting Customer Buying Pattern
@INPROCEEDINGS{10.4108/eai.7-12-2021.2314593, author={V. Umarani}, title={Investigation of KNN and Decision Tree Induction Modelin Predicting Customer Buying Pattern}, proceedings={Proceedings of the First International Conference on Combinatorial and Optimization, ICCAP 2021, December 7-8 2021, Chennai, India}, publisher={EAI}, proceedings_a={ICCAP}, year={2021}, month={12}, keywords={machine learning classification predicting customer behavior knn dt}, doi={10.4108/eai.7-12-2021.2314593} }
- V. Umarani
Year: 2021
Investigation of KNN and Decision Tree Induction Modelin Predicting Customer Buying Pattern
ICCAP
EAI
DOI: 10.4108/eai.7-12-2021.2314593
Abstract
Machine Learning Techniques is playing a key role in almost all domains. More specifically its role in ecommerce industry is inevitable since these techniques are able to predict and track the buying pattern of its customers. Moreover, majority of businesses put lots of effort in order to survive and gain a competitive edge over their competitors.Today,enormous research has paved way toapply differentMachine Learning models inpredicting the buyers’ behavior and thereby helping the ecommerce websitesto formulate a good marketing strategy. Hence it becomes imperative for any company to better understand the customers for increasing their digital presence and predicting how customers will respond to its marketing strategies. Classification is one of the category of supervised machine learning algorithms that has its hands on various applications domains such as in credit approval, medical diagnosis, target marketing etc. in which the input data is classified in any one of the target variable. Several studies shows the application of predictive models viz., Naïve Bayes Methods, Support Vector Machines(SVM), KNearest Neighbor (KNN), Decision Tree (DT) algorithms in various domains. The main aim of this research work is to investigate KNN and DT models for predicting customer buying pattern based on the gender, age and salary. with an accuracy rate of 95%, while the accuracy rate of KNN was 87%.