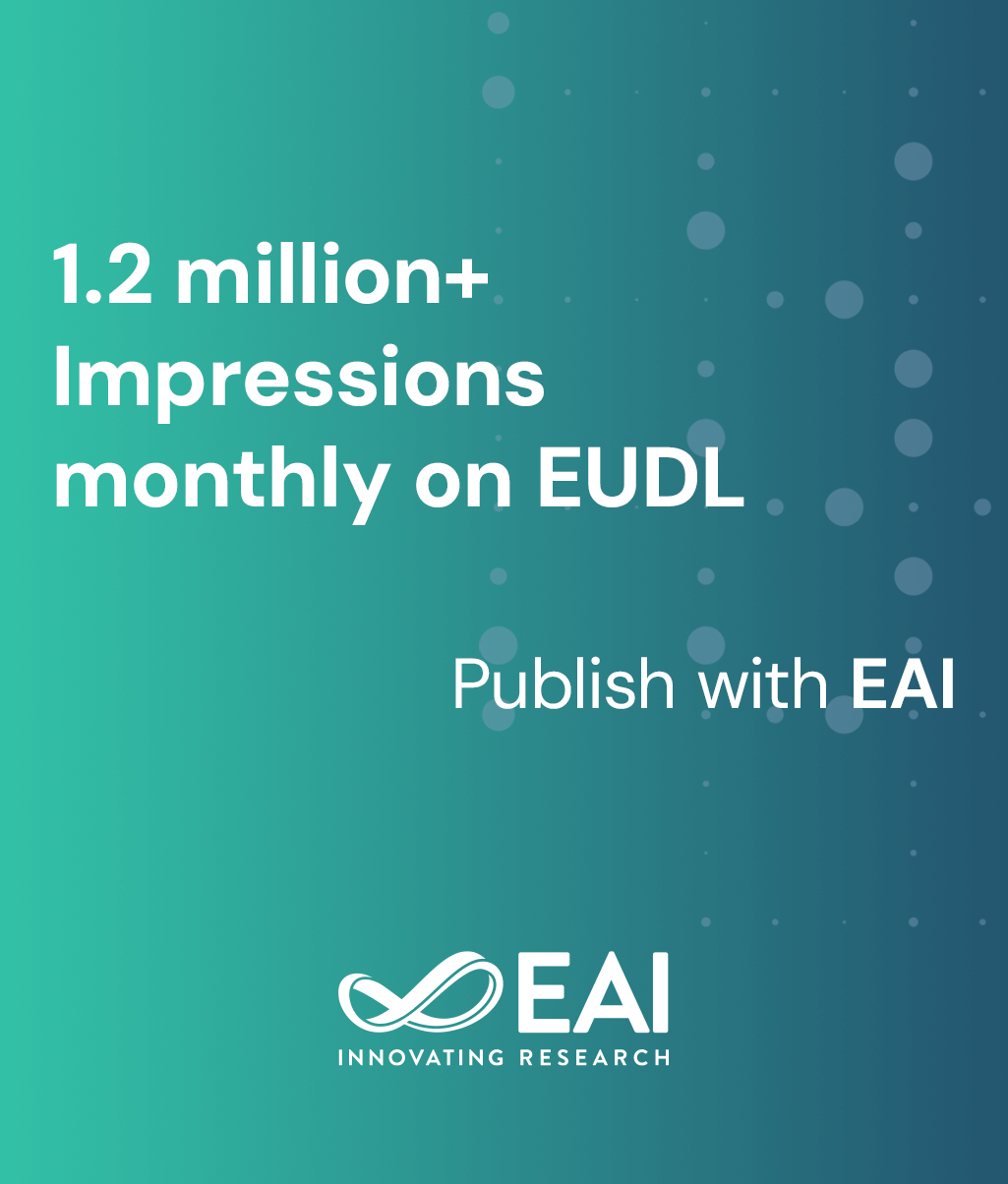
Research Article
Detection of Epileptic Seizure using Fast Adaptive Neuro Fuzzy Inference System
@INPROCEEDINGS{10.4108/eai.7-12-2021.2314517, author={Karthika Renuka D and Ashok Kumar L}, title={Detection of Epileptic Seizure using Fast Adaptive Neuro Fuzzy Inference System}, proceedings={Proceedings of the First International Conference on Combinatorial and Optimization, ICCAP 2021, December 7-8 2021, Chennai, India}, publisher={EAI}, proceedings_a={ICCAP}, year={2021}, month={12}, keywords={electroencephalogram epilepsy spatial constraints extreme machine learning}, doi={10.4108/eai.7-12-2021.2314517} }
- Karthika Renuka D
Ashok Kumar L
Year: 2021
Detection of Epileptic Seizure using Fast Adaptive Neuro Fuzzy Inference System
ICCAP
EAI
DOI: 10.4108/eai.7-12-2021.2314517
Abstract
A diverse range of epilepsies suggest that its arousal may be caused by a variety of factors, but the whole process culminates in a common occurrence known as the seizure. The need for a precise and dependable seizure detector is critical, not only for a better understanding of seizures, but also as a benefit for medics who are fatigued by the prospect of picturing a continuous and massive long-term recording of seizures. The lack of an acceptable mix of methodologies, despite significant advancements in automated epilepsy diagnosis over the last decade, highlights the need for suitable seizure detection systems, which are not yet widely used. A wide variety of methods is being extensively explored, and every attempt is being made with the greatest amount of involvement in order to provide a strategy for seizure detection. For the delta, alpha, and beta bands, the power spectrum estimator (Welsh power spectrum estimator) was used to compute the frequency spectrum and the power. These data were utilised to create a feature vector, which was then used as an input to the next step of the process. The suggested classification algorithms are described in detail below. In order to identify the existence of a seizure in an EEG signal, a hybrid extreme machine learning is employed. It was decided to integrate the Modified Levenberg Marquardt algorithm (MLA) with the classifiers to decrease the time complexity.