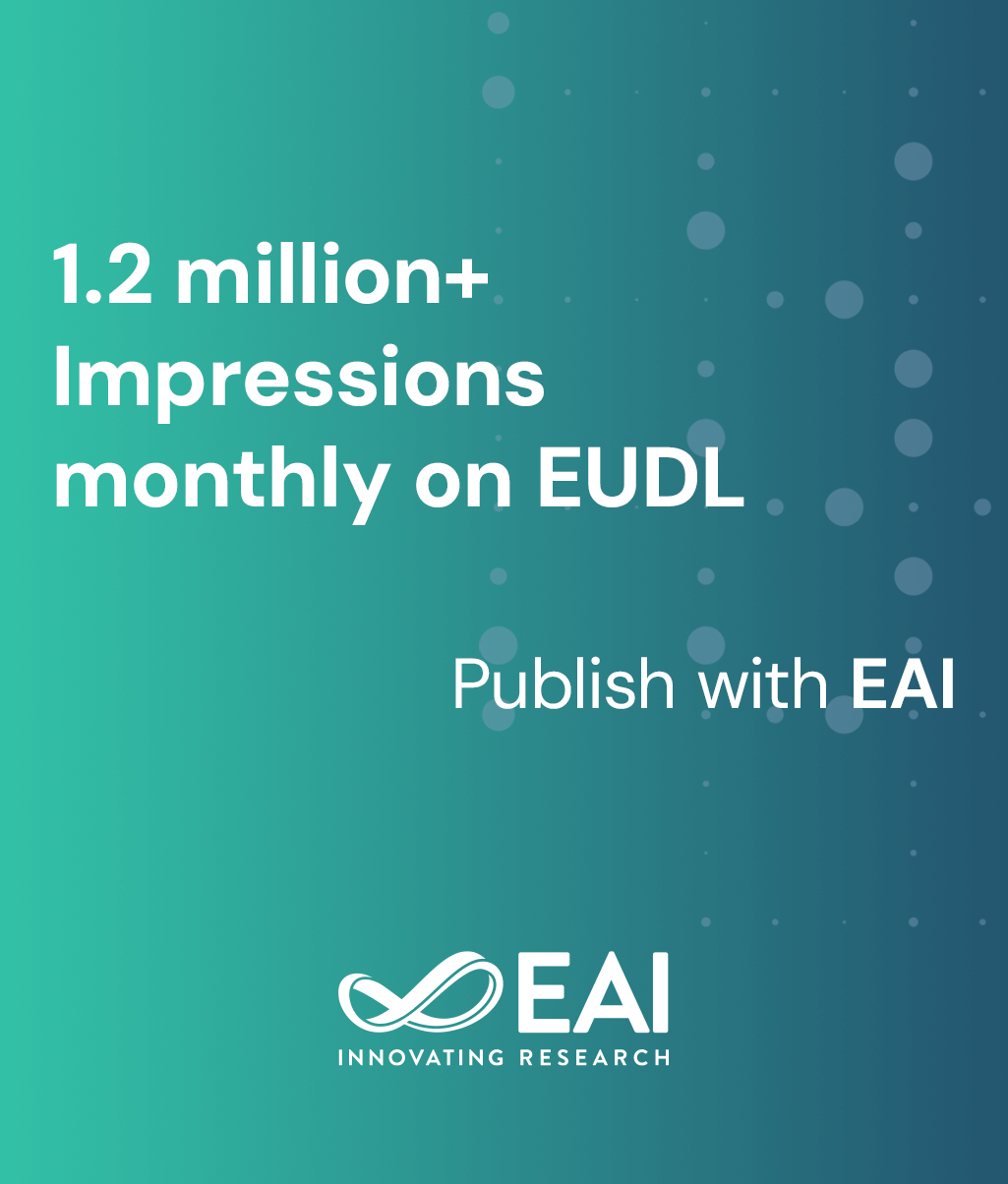
Research Article
Dirichlet Process Gaussian Mixture Model for Activity Discovery in Smart Homes with Ambient Sensors
@INPROCEEDINGS{10.4108/eai.7-11-2017.2275003, author={Thuong Nguyen and Qing Zhang and Duc Le and Mohan Karunanithi}, title={Dirichlet Process Gaussian Mixture Model for Activity Discovery in Smart Homes with Ambient Sensors}, proceedings={14th EAI International Conference on Mobile and Ubiquitous Systems: Computing, Networking and Services}, publisher={ACM}, proceedings_a={MOBIQUITOUS}, year={2018}, month={4}, keywords={activity discovery smart home ambient sensing unsupervised learning bayesian nonparametric dirichlet process}, doi={10.4108/eai.7-11-2017.2275003} }
- Thuong Nguyen
Qing Zhang
Duc Le
Mohan Karunanithi
Year: 2018
Dirichlet Process Gaussian Mixture Model for Activity Discovery in Smart Homes with Ambient Sensors
MOBIQUITOUS
ACM
DOI: 10.4108/eai.7-11-2017.2275003
Abstract
Existing approaches to activity recognition in smart homes mostly rely on supervised learning from well-annotated sensor data, acquired in a controled lab environment. However obtaining such labeled data in real home scenarios could be prohibitive due to either the privacy concerns of using cameras, or the low adherence of self reports done by home residents. Unsupervised learning, on the other hand, aims at discovering activities through applying fixed complexity models, yet assuming apriori knowledge of the number of activities. Again this is also a non-practical approach because the number of activities could vary drastically, even within a home. In this paper, we propose a novel practical unsupervised Bayesian nonparametric model to discover activities in smart homes, without prior assumption on the number of activities. Instead, our model can automatically infer such number only from sensor readings, thus it can be easily applied to any new home. We test our method on a public dataset and a dataset collected in our project. On the CASAS dataset, which has activity labels, our approach can achieve the performance close to the best of GMM and outperforms Kmeans. On our smart home dataset, the discovered activities are highly correlated with the typical daily routine of the resident. Such experimental results demonstrate the eciency of our method for activity discovery in smart homes.