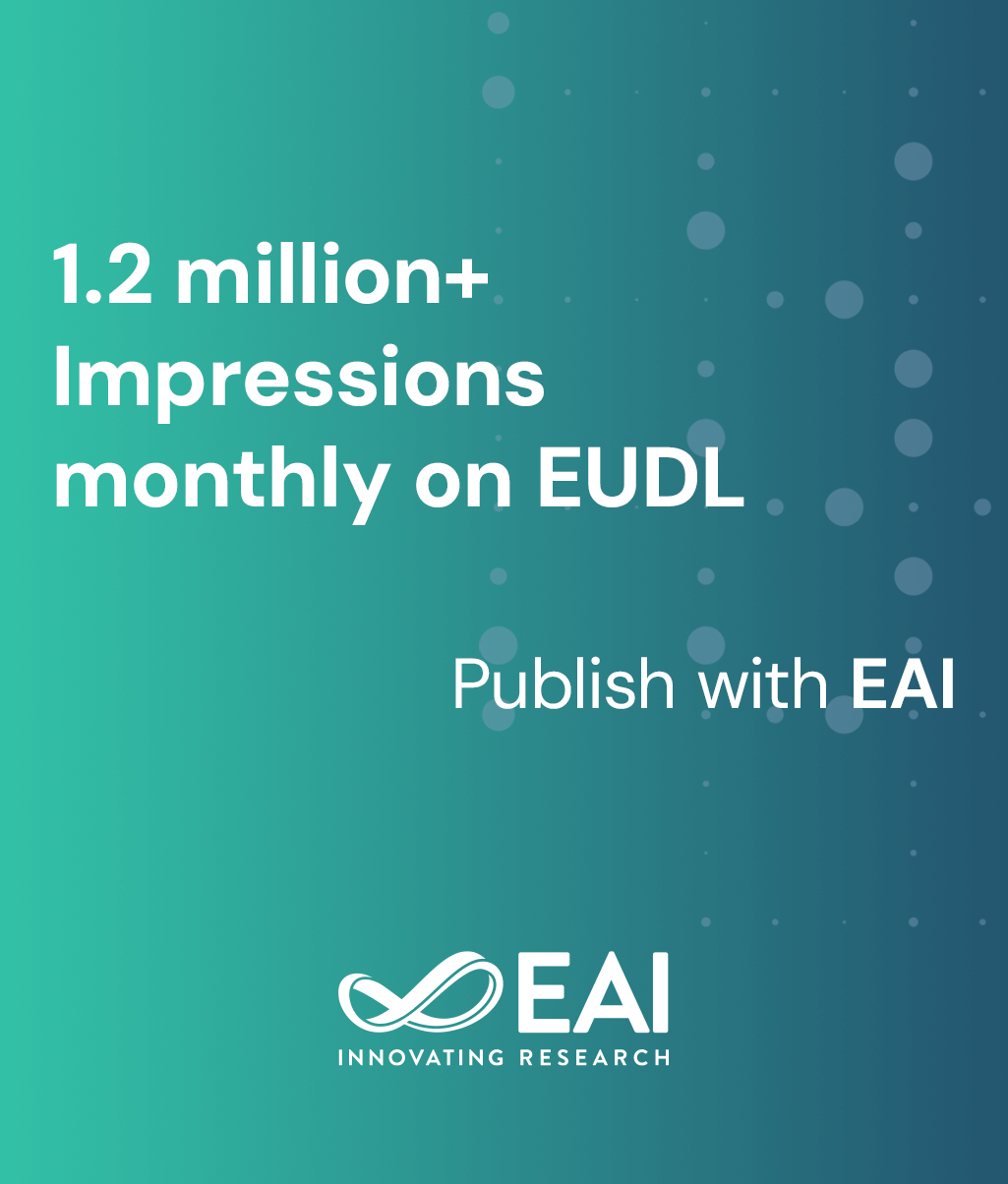
Research Article
Capturing Daily Student Life by Recognizing Complex Activities Using Smartphones
@INPROCEEDINGS{10.4108/eai.7-11-2017.2273984, author={Christian Meurisch and Artur Gogel and Benedikt Schmidt and Timo Nolle and Frederik Janssen and Immanuel Schweizer and Max M\'{y}hlh\aa{}user}, title={Capturing Daily Student Life by Recognizing Complex Activities Using Smartphones}, proceedings={14th EAI International Conference on Mobile and Ubiquitous Systems: Computing, Networking and Services}, publisher={ACM}, proceedings_a={MOBIQUITOUS}, year={2018}, month={4}, keywords={student life user behavior analysis mobile sensing activity recognition heterogeneous data sources smartphone}, doi={10.4108/eai.7-11-2017.2273984} }
- Christian Meurisch
Artur Gogel
Benedikt Schmidt
Timo Nolle
Frederik Janssen
Immanuel Schweizer
Max Mühlhäuser
Year: 2018
Capturing Daily Student Life by Recognizing Complex Activities Using Smartphones
MOBIQUITOUS
ACM
DOI: 10.4108/eai.7-11-2017.2273984
Abstract
In-depth understanding of student life is essential to proactively support students in their academic educations. However, there is no work that identifies and recognizes a sufficient set of activities to capture a daily student life since complex activity recognition is still challenging. In this paper, we address this issue by recognizing 10 complex student activities such as learning, attending a lecture, or sleeping. We first identify these relevant student activities by conducting a pre-study with 21 students aiming to get an insight into their daily lives. Based on this outcome, we design our sensing applications to collect an appropriate dataset including user-annotated ground-truth data from 163 students over 4 weeks. We investigate different multi-class hierarchical approaches, as well as compare general models against individual models. The results show that our approaches consistently outperform the baseline classifiers and achieve F1-scores of 82.3% for the 1st level, 83.4% and 72.7% for both 2nd levels (study-related and non-study-related activities) on a merged activity set using individual models. The findings offer a novel way to capture a daily student life and can be used to support or guide students in their study.