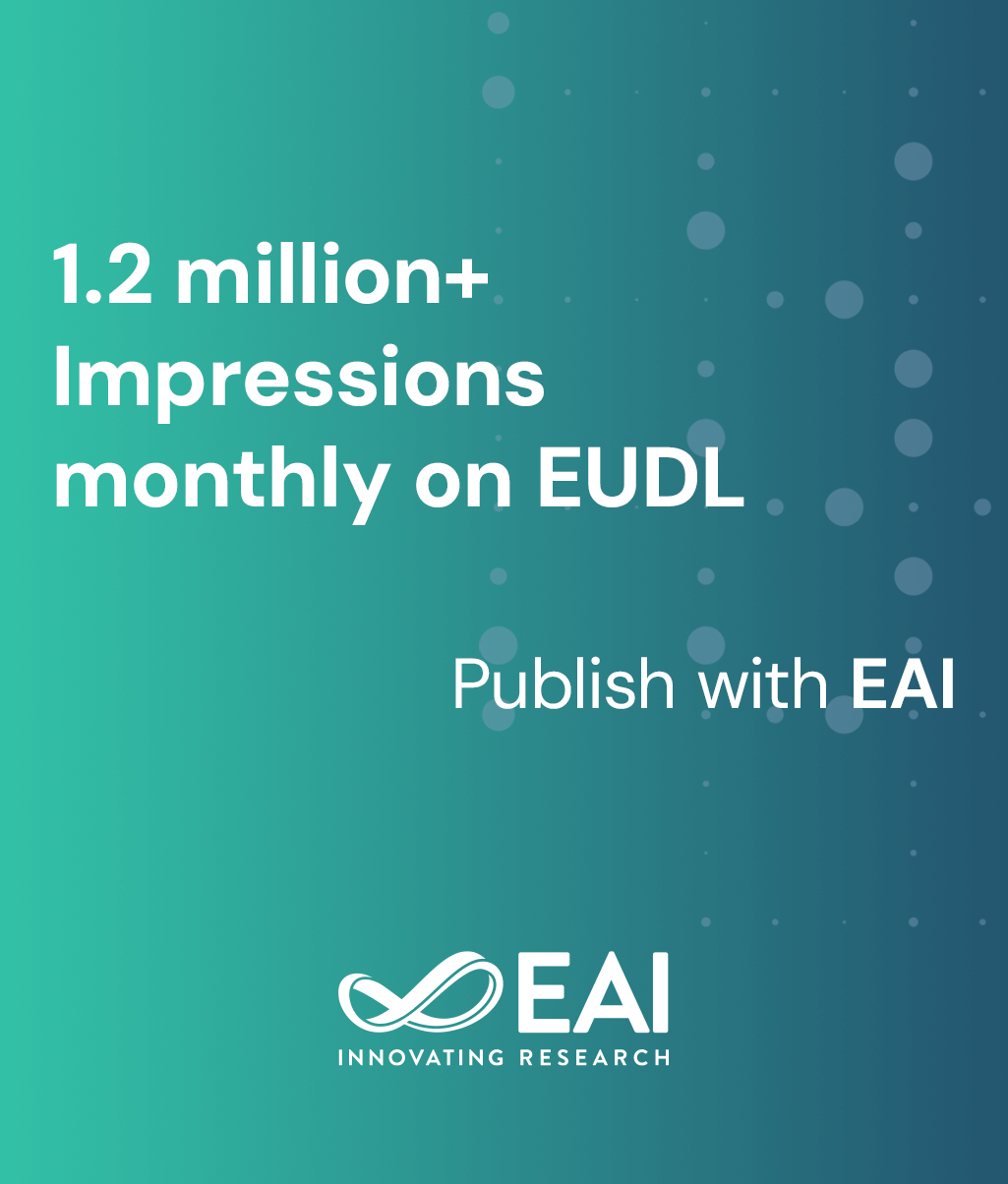
Research Article
ParkUs 2.0: Automated Cruise Detection for Parking Availability Inference
@INPROCEEDINGS{10.4108/eai.7-11-2017.2273778, author={Michael Jones and Aftab Khan and Parag Kulkarni and Pietro Carnelli and Mahesh Sooriyabandara}, title={ParkUs 2.0: Automated Cruise Detection for Parking Availability Inference}, proceedings={14th EAI International Conference on Mobile and Ubiquitous Systems: Computing, Networking and Services}, publisher={ACM}, proceedings_a={MOBIQUITOUS}, year={2018}, month={4}, keywords={activity recognition smart parking cruise detection mobile sensing automated annotation}, doi={10.4108/eai.7-11-2017.2273778} }
- Michael Jones
Aftab Khan
Parag Kulkarni
Pietro Carnelli
Mahesh Sooriyabandara
Year: 2018
ParkUs 2.0: Automated Cruise Detection for Parking Availability Inference
MOBIQUITOUS
ACM
DOI: 10.4108/eai.7-11-2017.2273778
Abstract
Recent studies show that a key contributor to congestion and increased CO2 emissions within cities are drivers searching (or cruising) to find a vacant on-street parking space. It has been shown that approximately (depending on the city) 20-30% of vehicles in congested urban areas were cruising to find a parking space with a parking search time varying in the order of several minutes. In the city of Bristol alone, we have shown, using our collected trip and publicly available census data that over 790 metric tons of CO2 is generated every year due to cruising. At a total cost of £368,000 (US$467,000) in terms of fuel wasted. The solution, described in this paper, aims to reduce parking search times using our automated real-time parking system called ParkUs 2.0. Our proposed method leverages sensor and location data collected from smartphones (carried by drivers), uses machine learning (classification) to detect cruising behaviour, automatically annotates parking availability on road segments based on the classified data and displays this information as a heatmap of parking availability information on the user’s smartphone. This is the first such attempt to automatically detect cruising to the best of our knowledge. Evaluation through controlled trials with volunteer participants highlights the potential of our novel approach as we are able to detect cruising with an accuracy of 81%.