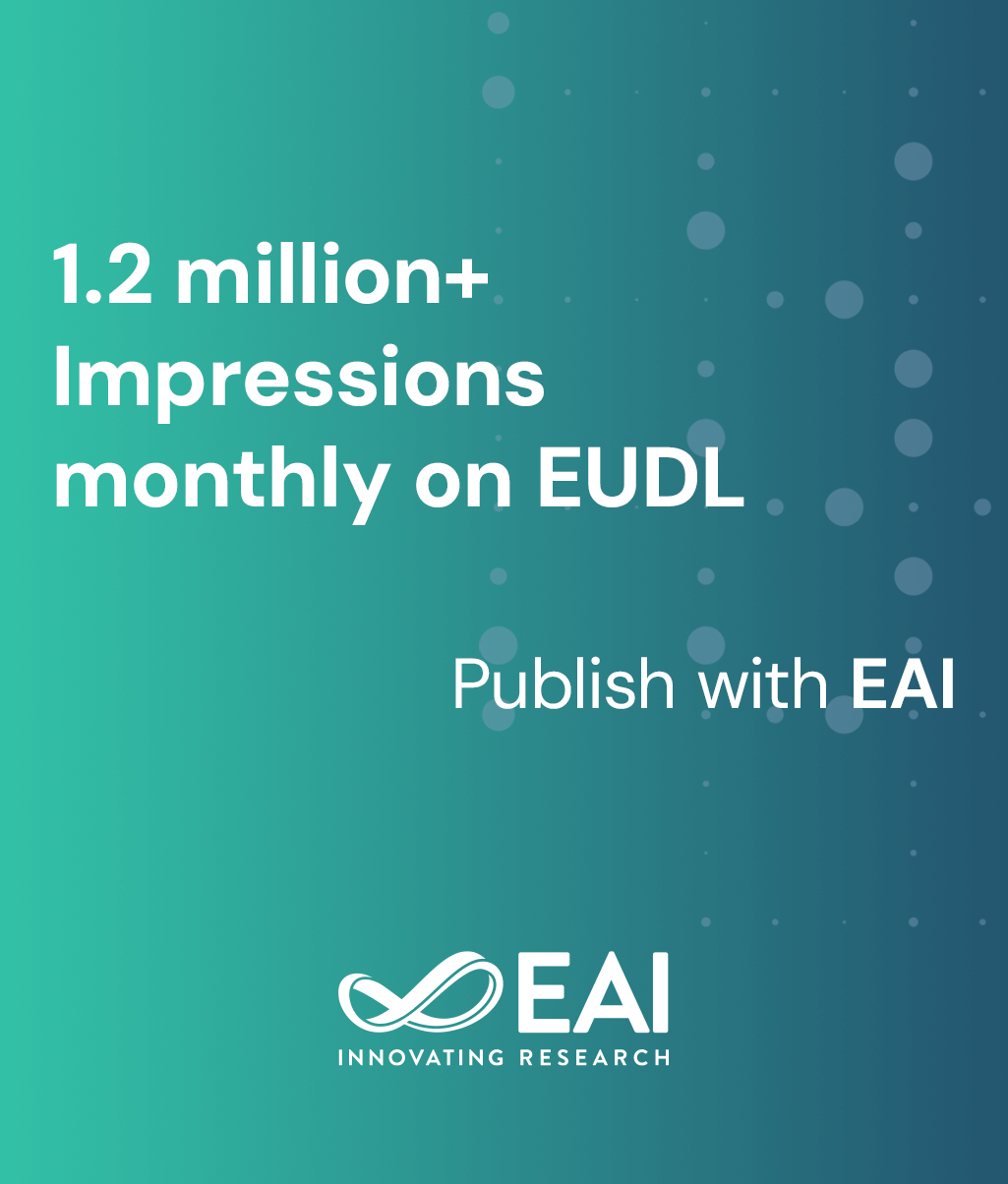
Research Article
Follow The Best: Crowdsourced Automated Travel Advice
@INPROCEEDINGS{10.4108/eai.7-11-2017.2273723, author={Abdullah Saleh Aldwyish and Egemen Tanin and Shanika Karunasekera}, title={Follow The Best: Crowdsourced Automated Travel Advice}, proceedings={14th EAI International Conference on Mobile and Ubiquitous Systems: Computing, Networking and Services}, publisher={ACM}, proceedings_a={MOBIQUITOUS}, year={2018}, month={4}, keywords={spatial databases location-based social networks}, doi={10.4108/eai.7-11-2017.2273723} }
- Abdullah Saleh Aldwyish
Egemen Tanin
Shanika Karunasekera
Year: 2018
Follow The Best: Crowdsourced Automated Travel Advice
MOBIQUITOUS
ACM
DOI: 10.4108/eai.7-11-2017.2273723
Abstract
Recently many navigation services have started to collect traffic data from drivers to enhance their services. The focus of their efforts are on real-time estimation of traffic conditions to offer better navigation services and help people avoid traffic incidents. However, using drivers as mere traffic sensors and aggregating their data disposes off any human knowledge and judgment that exists in their choices. Commuters in a city have a valuable knowledge about their city traffic patterns as a result of their daily commute over many years. In our work we take a different approach to navigation advice, we use individual driver trajectories to deduce travel choices. Our goal is to help drivers avoid traffic events by providing routes that bypassed congested routes during similar events in the past. We present a solution that combines graph search and trajectory search to find the fastest path taken in the past using historical trajectories under similar conditions in a city. We evaluate our approach through extensive experiments in realistic settings and experimental results show that our solution was able to find faster paths, avoiding the congested areas in cases where state-of-the-art methods resulted in drivers leading to routes through congested areas.