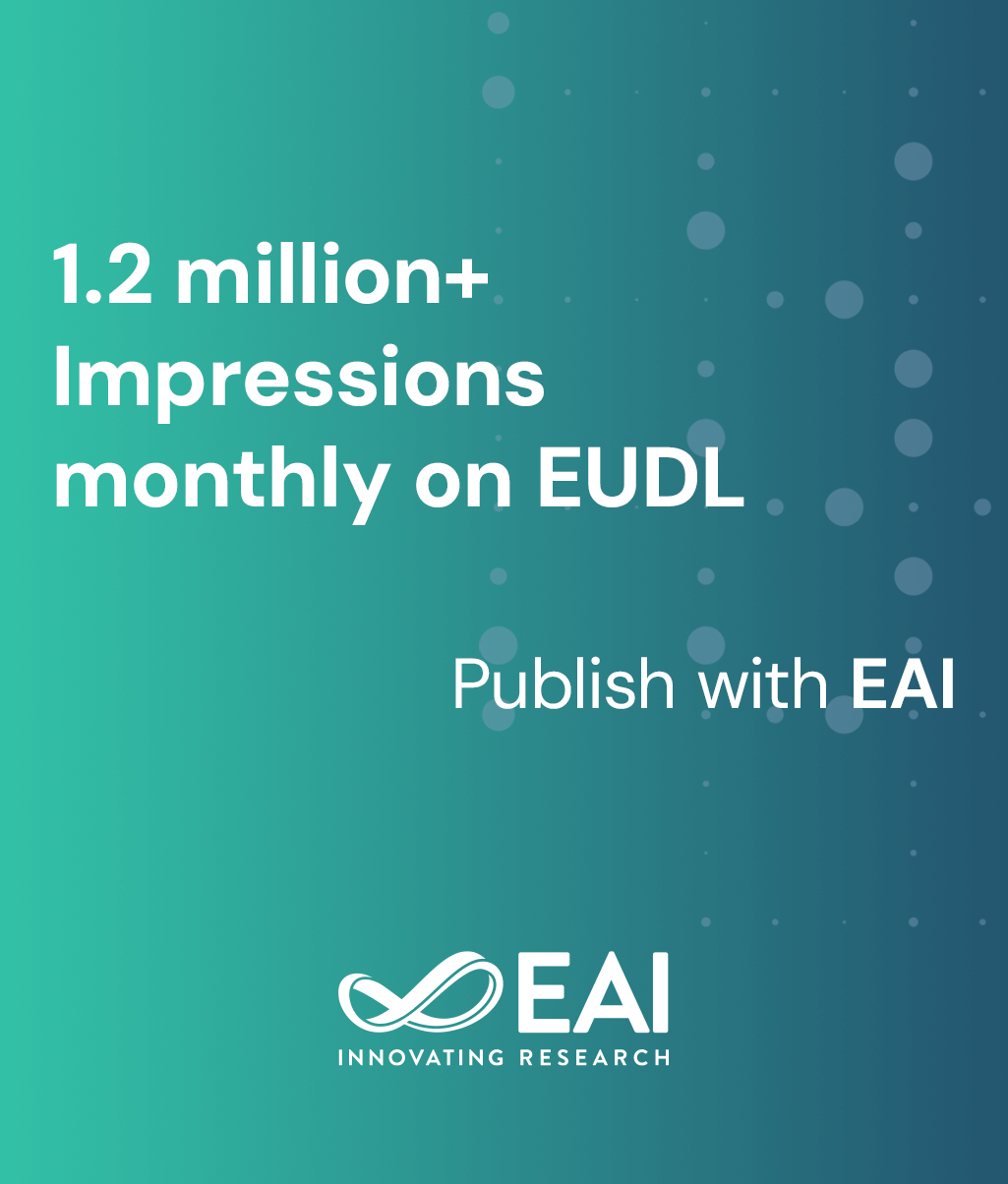
Research Article
Markov Dynamic Subsequence Ensemble for Energy-Efficient Activity Recognition
@INPROCEEDINGS{10.4108/eai.7-11-2017.2273710, author={Weihao Cheng and Sarah Erfani and Rui Zhang and Ramamohanarao Kotagiri}, title={Markov Dynamic Subsequence Ensemble for Energy-Efficient Activity Recognition}, proceedings={14th EAI International Conference on Mobile and Ubiquitous Systems: Computing, Networking and Services}, publisher={ACM}, proceedings_a={MOBIQUITOUS}, year={2018}, month={4}, keywords={activity recognition energy efficiency markov decision process}, doi={10.4108/eai.7-11-2017.2273710} }
- Weihao Cheng
Sarah Erfani
Rui Zhang
Ramamohanarao Kotagiri
Year: 2018
Markov Dynamic Subsequence Ensemble for Energy-Efficient Activity Recognition
MOBIQUITOUS
ACM
DOI: 10.4108/eai.7-11-2017.2273710
Abstract
Ubiquitous mobile computing technology provides opportunities for accurate Activity Recognition (AR). Recently, ensemble models using multiple feature representations based on time series subsequences have demonstrated excellent performance on recognition accuracy. However, these models can significantly increase the energy overhead and shorten battery lifespans of the mobile devices. We formalize a dynamic subsequence selection problem that minimizes the computational cost while persevering a high recognition accuracy. To solve the problem, we propose Markov Dynamic Subsequence Ensemble (MDSE), an algorithm for the selection of the subsequences via a Markov Decision Process (MDP), where a policy is learned for choosing the best subsequence given the state of prediction. Regarding MDSE, we derive an upper bound of the expected ensemble size, so that the energy consumption caused by the computations of the proposed method is guaranteed. Extensive experiments are conducted on 6 real AR datasets to evaluate the effectiveness of MDSE. Compared to the state-of-the-art methods, MDSE reduces 70.8% computational cost which is 3.42 times more energy efficient, and achieves a comparably high accuracy.