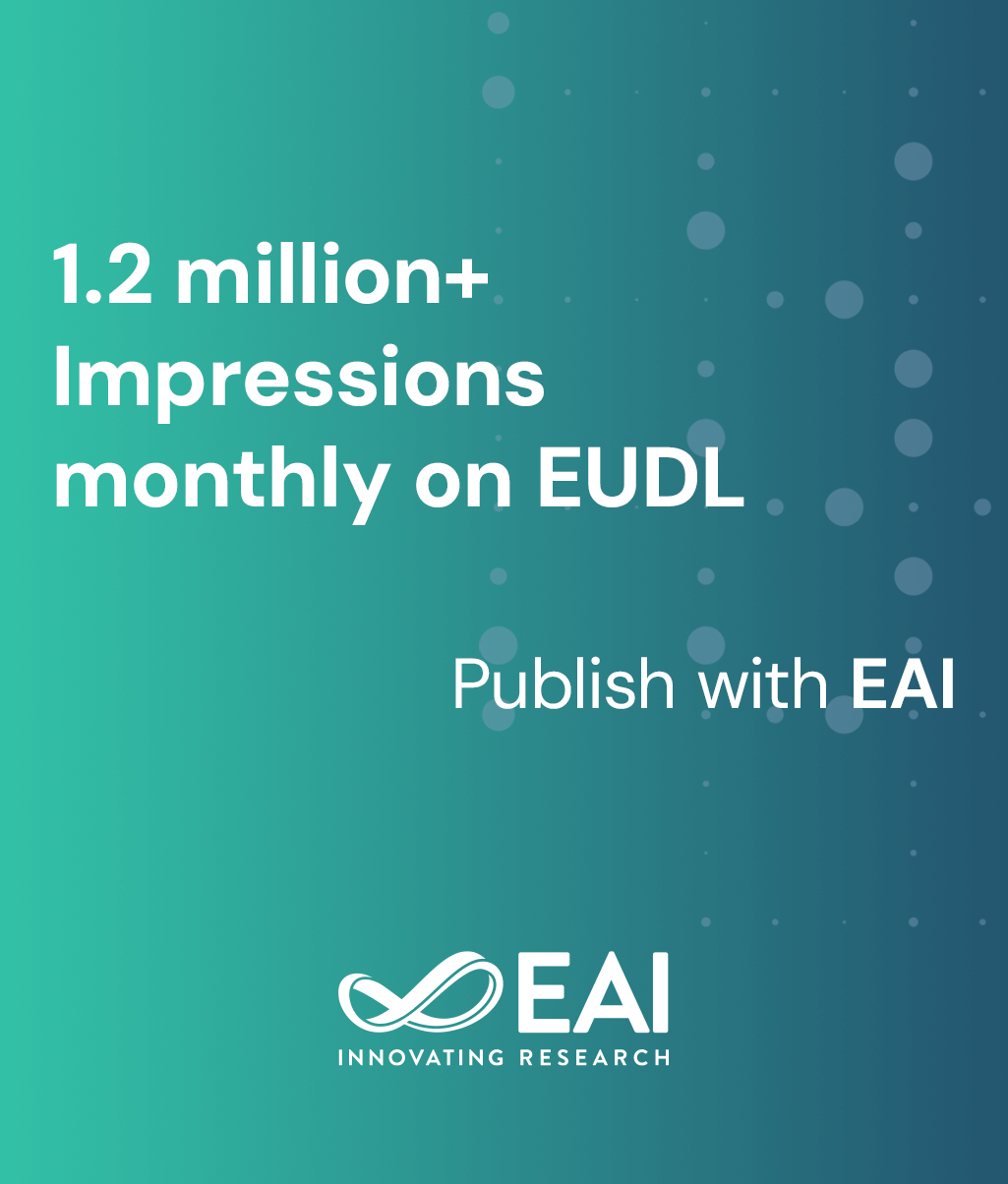
Research Article
Multi-Person Brain Activity Recognition via Comprehensive EEG Signal Analysis
@INPROCEEDINGS{10.4108/eai.7-11-2017.2273696, author={Xiang Zhang and Lina Yao and Dalin Zhang and Xianzhi Wang and Quan Z. Sheng and Tao Gu}, title={Multi-Person Brain Activity Recognition via Comprehensive EEG Signal Analysis}, proceedings={14th EAI International Conference on Mobile and Ubiquitous Systems: Computing, Networking and Services}, publisher={ACM}, proceedings_a={MOBIQUITOUS}, year={2018}, month={4}, keywords={brain computer interface eeg classification activity recognition}, doi={10.4108/eai.7-11-2017.2273696} }
- Xiang Zhang
Lina Yao
Dalin Zhang
Xianzhi Wang
Quan Z. Sheng
Tao Gu
Year: 2018
Multi-Person Brain Activity Recognition via Comprehensive EEG Signal Analysis
MOBIQUITOUS
ACM
DOI: 10.4108/eai.7-11-2017.2273696
Abstract
An electroencephalography (EEG) based brain activity recogni- tion is a fundamental field of study for a number of significant applications such as intention prediction, appliance control, and neurological disease diagnosis in smart home and smart healthcare domains. Existing techniques mostly focus on binary brain activity recognition for a single person, which limits their deployment in wider and complex practical scenarios. Therefore, multi-person and multi-class brain activity recognition has obtained popularity recently. Another challenge faced by brain activity recognition is the low recognition accuracy due to the massive noises and the low signal-to-noise ratio in EEG signals. Moreover, the feature engineering in EEG processing is time-consuming and highly re- lies on the expert experience. In this paper, we attempt to solve the above challenges by proposing an approach which has better EEG interpretation ability via raw Electroencephalography (EEG) signal analysis for multi-person and multi-class brain activity recog- nition. We evaluate our approach on both a public and a local EEG datasets and conduct extensive experiments to explore the effect of several factors on the recognition results. The experimental results show that our approach achieves a high accuracy comparing to competitive state-of-the-art methods, indi- cating its potential in promoting future research on multi-person EEG recognition.