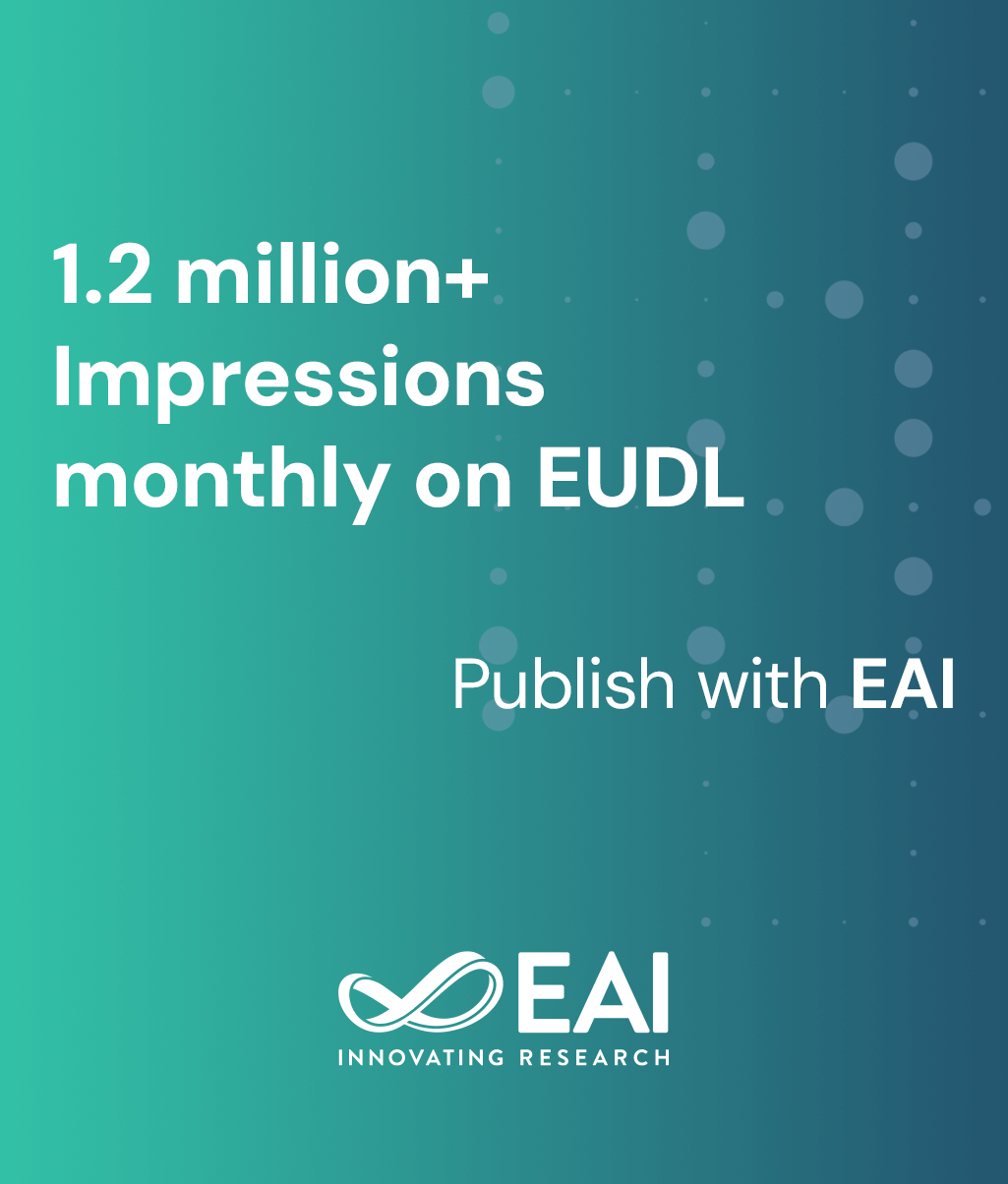
Research Article
GraMap: QoS-Aware Indoor Mapping Through Crowd-Sensing Point Clouds with Grammar Support
@INPROCEEDINGS{10.4108/eai.7-11-2017.2273627, author={Mohamed Abdelaal and Frank Duerr and Kurt Rothermel and Susanne Becker and Dieter Fritsch}, title={GraMap: QoS-Aware Indoor Mapping Through Crowd-Sensing Point Clouds with Grammar Support}, proceedings={14th EAI International Conference on Mobile and Ubiquitous Systems: Computing, Networking and Services}, publisher={ACM}, proceedings_a={MOBIQUITOUS}, year={2018}, month={4}, keywords={crowd-sensing indoor modeling energy efficiency formal grammars}, doi={10.4108/eai.7-11-2017.2273627} }
- Mohamed Abdelaal
Frank Duerr
Kurt Rothermel
Susanne Becker
Dieter Fritsch
Year: 2018
GraMap: QoS-Aware Indoor Mapping Through Crowd-Sensing Point Clouds with Grammar Support
MOBIQUITOUS
ACM
DOI: 10.4108/eai.7-11-2017.2273627
Abstract
Recently, several approaches have been proposed to automatically model indoor environments. Most of such efforts principally rely on the crowd to sense data such as motion traces, images, and WiFi footprints. In this paper, we propose GraMap, a QoS-aware automatic indoor modeling approach through crowd-sensing 3D point clouds. GraMap exploits a recently-developed sensors fusion mechanism, namely Tango technology, to cooperatively collect point clouds from the crowd. Afterward, a set of backend servers extracts the required geometrical information to derive indoor models. To improve the energy efficiency of the mobile devices, GraMap performs data quality assurance along with 3D data compression. Specifically, we propose a probabilistic quality model---implemented on the mobile devices---to ensure high-quality of the captured point clouds. In this manner, we conserve energy via sidestepping the repetition of sensing queries due to uploading low-quality point clouds. However, the resultant indoor models may still suffer from incompleteness and inaccuracies. Therefore, GraMap leverages formal grammars which encode design-time knowledge, i.e. structural information about the building, to enhance the quality of the derived models. To examine the effectiveness of GraMap, we implemented an Android App to collect point clouds from volunteers. We show that GraMap derives highly-accurate models while reducing the energy costs of pre-processing and reporting the point clouds.