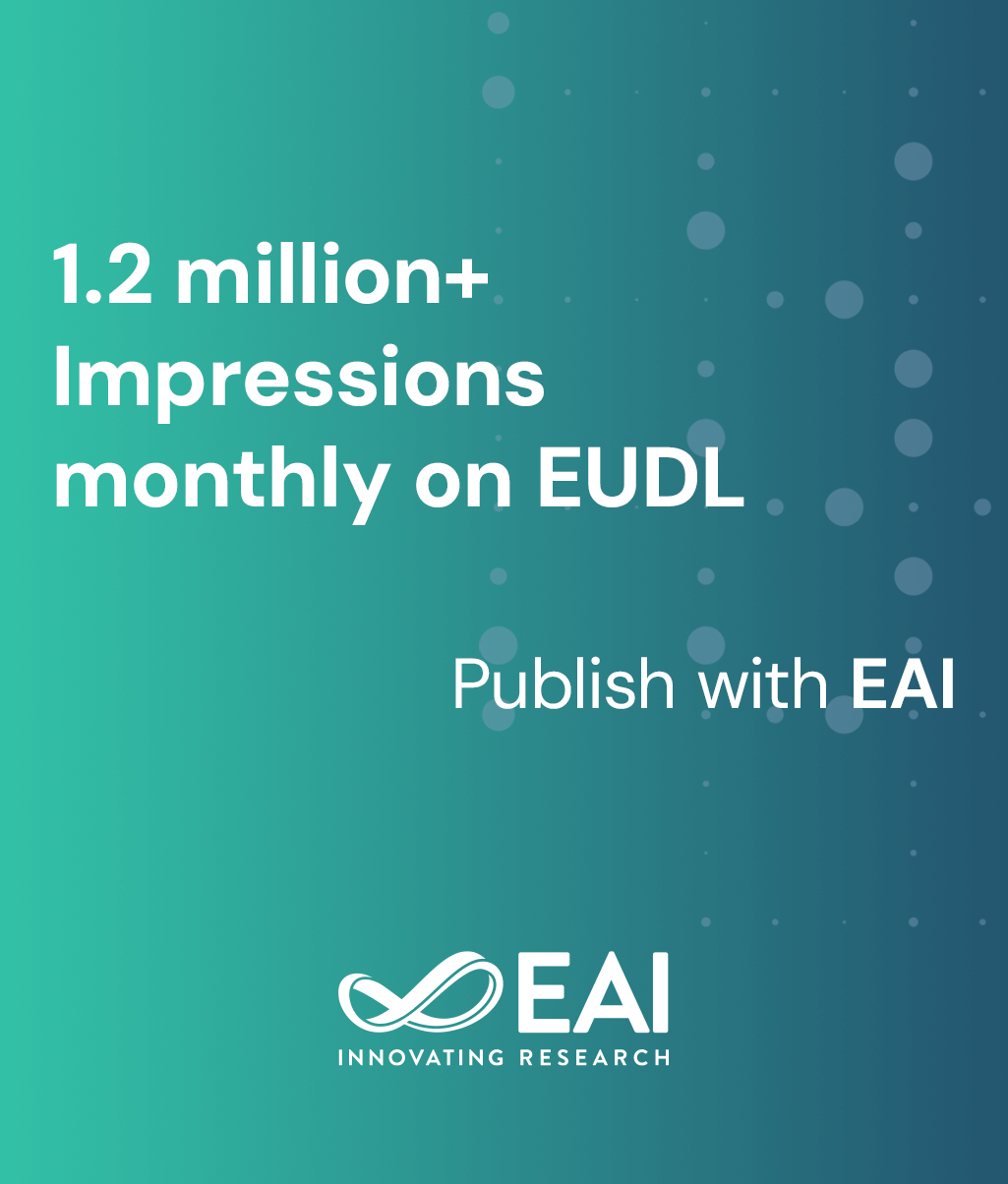
Research Article
It Can be Cheaper: Using Price Prediction to Obtain Better Prices from Dynamic Pricing in Ride-on-demand Services
@INPROCEEDINGS{10.4108/eai.7-11-2017.2273476, author={Suiming Guo and Chao Chen and Yaxiao Liu and Ke Xu and Dah Ming Chiu}, title={It Can be Cheaper: Using Price Prediction to Obtain Better Prices from Dynamic Pricing in Ride-on-demand Services}, proceedings={14th EAI International Conference on Mobile and Ubiquitous Systems: Computing, Networking and Services}, publisher={ACM}, proceedings_a={MOBIQUITOUS}, year={2018}, month={4}, keywords={price prediction ride-on-demand service dynamic pricing limit of predictability}, doi={10.4108/eai.7-11-2017.2273476} }
- Suiming Guo
Chao Chen
Yaxiao Liu
Ke Xu
Dah Ming Chiu
Year: 2018
It Can be Cheaper: Using Price Prediction to Obtain Better Prices from Dynamic Pricing in Ride-on-demand Services
MOBIQUITOUS
ACM
DOI: 10.4108/eai.7-11-2017.2273476
Abstract
In emerging ride-on-demand (RoD) services such as Uber or Didi (in China), dynamic pricing plays an important role in regulating supply and demand, trying to make such service, to some extent, more convenient for passengers. Despite the convenience, dynamic pricing also exerts mental burden on passengers: they wonder whether the current price is low enough to accept, or if it is not, what they could do to get a lower price. Without extra information, passengers sometimes feel anxious and lose satisfaction. It is thus necessary to provide more information to relieve the anxiety, and price prediction is one of the solutions.
In this paper we predict the dynamic prices to help passengers understand whether they could get a lower price in neighboring locations or within a short time. We first divide a city into rectangular cells, and use entropy and the temporal correlation of prices to characterize the predictability of prices of each cell. Based on the predictability of prices, we claim that different prediction algorithms should be used in different city areas, to balance between efficiency and accuracy. We design and implement two predictors, namely a Markov predictor and a neural network predictor, and evaluate their performance based on the real data from a major RoD service provider in China. The results verify that the Markov predictor works well enough in highly-predictable areas, and the neural network predictor works better in areas with lower predictabilities.