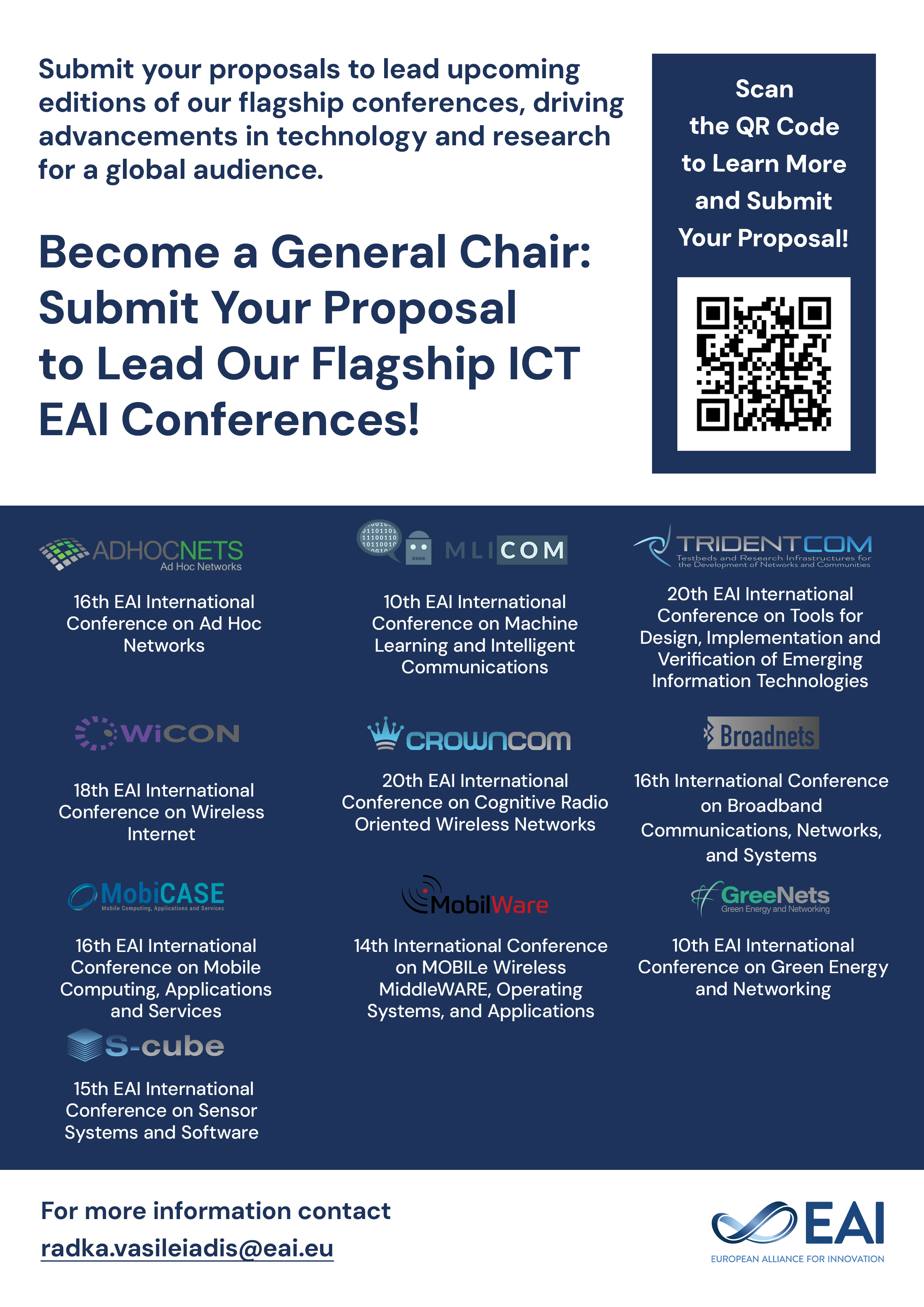
Research Article
Quantitative Research on Stock Multi-Factor Models in Empirical Investment
@INPROCEEDINGS{10.4108/eai.6-9-2024.2353667, author={Chengzhao Zhang and Xun Huang and Hairong Li and Jingxin Zhao}, title={Quantitative Research on Stock Multi-Factor Models in Empirical Investment}, proceedings={Proceedings of the 3rd International Conference on Public Management, Digital Economy and Internet Technology, ICPDI 2024, September 6--8, 2024, Jinan, China}, publisher={EAI}, proceedings_a={ICPDI}, year={2024}, month={12}, keywords={multi-factor; quantitative stock investment; volatility; portfolio; csi300}, doi={10.4108/eai.6-9-2024.2353667} }
- Chengzhao Zhang
Xun Huang
Hairong Li
Jingxin Zhao
Year: 2024
Quantitative Research on Stock Multi-Factor Models in Empirical Investment
ICPDI
EAI
DOI: 10.4108/eai.6-9-2024.2353667
Abstract
This study aims to empirically examine the efficacy of a multi-factor model in quantitative stock investment. The model integrates various factors such as value, size, momentum, and volatility, which are well-established in academic research and have been shown to significantly influence stock returns. Utilizing a comprehensive dataset of stock market information, we establish factor portfolios based on these factors and evaluate their performance over a specific time frame. The empirical findings indicate that the multi-factor model surpasses conventional single-factor models in terms of risk-adjusted returns. Specifically, the value and momentum factors demonstrate notable positive alphas, signifying their capacity to generate excess returns beyond market risk considerations. Additionally, the size and volatility factors also exhibit substantial impacts on stock returns, further underscoring the efficacy of the multi-factor model in capturing stock price movements. During a specific time period, this study focused on the constituents of the CSI300 Index (China Securities Index 300) and selected 12 factors, including industry, technical, and financial factors, to establish an initial factor pool. Subsequently, a multi-factor stock selection model based on neural networks was developed and backtested. The empirical results demonstrated that this investment strategy yielded higher excess returns compared to the benchmark index.