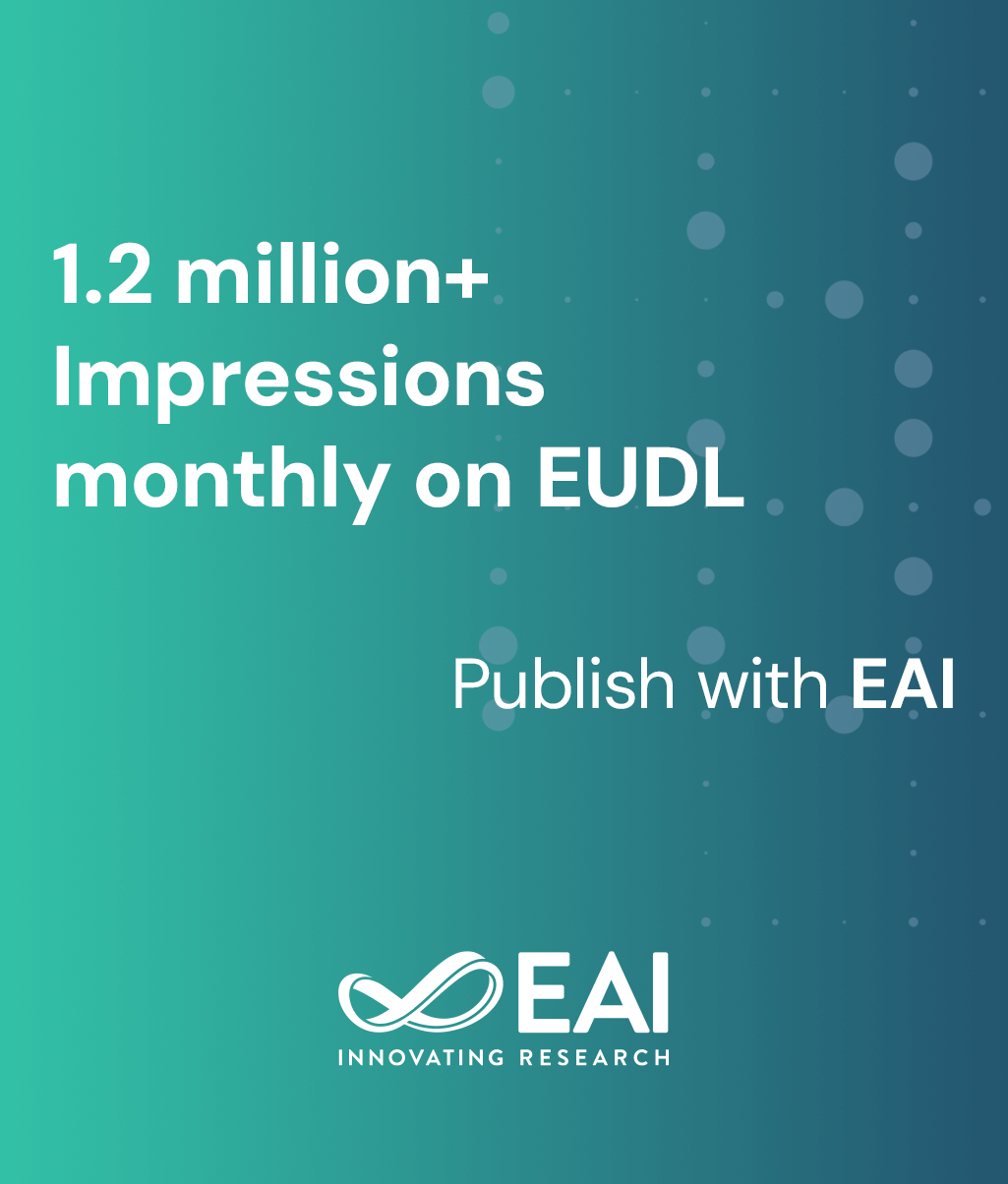
Research Article
Parking Space Recognition Based on Deep Convolutional Neural Network
@INPROCEEDINGS{10.4108/eai.6-6-2021.2307757, author={Zhuowen Chen and Zijun Gao and Jiaqi Li and Junjie Zhang and Yanan Dai and Wenbo Hu and Changmao Li}, title={Parking Space Recognition Based on Deep Convolutional Neural Network}, proceedings={Proceedings of the 8th EAI International Conference on Green Energy and Networking, GreeNets 2021, June 6-7, 2021, Dalian, People’s Republic of China}, publisher={EAI}, proceedings_a={GREENETS}, year={2021}, month={8}, keywords={pretreatment deep learning point transformation senet network feature enhancement}, doi={10.4108/eai.6-6-2021.2307757} }
- Zhuowen Chen
Zijun Gao
Jiaqi Li
Junjie Zhang
Yanan Dai
Wenbo Hu
Changmao Li
Year: 2021
Parking Space Recognition Based on Deep Convolutional Neural Network
GREENETS
EAI
DOI: 10.4108/eai.6-6-2021.2307757
Abstract
Automatic real-time recognition for lots of spots is a crucial and challenging task, which can significantly improve the intelligence level of a city and make it that much more convenient for people to travel. Deep learning models have been applied in various fields, and significant development has also been achieved. However, taking into account interference in recognition images and occlusions of lane lines, deep learning still lacks accuracy and real-time.Therefore, the paper argues that combining OpenCV processing and deep learning should be utilized. This method can improve recognition for identifying parking spaces and increase the intellectualization of a city. In this paper, a network model based on VGG19 has been proposed, and the SENet network module has been added to its output along with a performing secondary enhancement on training images. Advanced pre-processing to obtain accurate point coordinates has finally obtained a satisfactory effect. It also predicts images with severe interference and images with line occlusion. These two experimental results show that the network can effectively identify even with severe interference. At the same time, a large number of experimental evaluations also show that this method can also be applied to small target recognitions in many fields and can also be used as a basic product for small target detections and recognitions in the future.