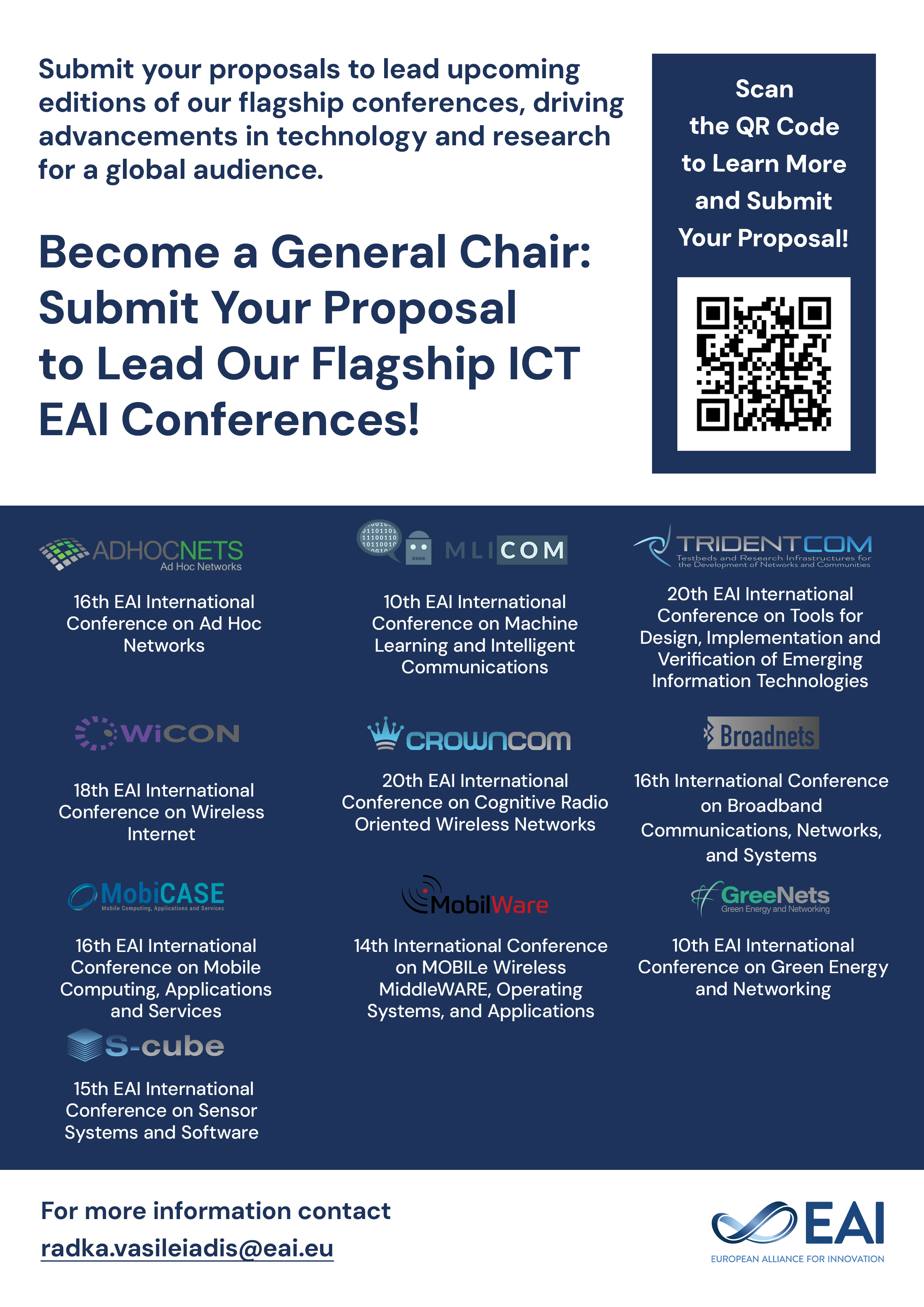
Research Article
A hybrid feature selection method for credit scoring
@ARTICLE{10.4108/eai.6-3-2017.152335, author={Sang Ha Van and Nam Nguyen Ha and Hien Nguyen Thi Bao}, title={A hybrid feature selection method for credit scoring}, journal={EAI Endorsed Transactions on Context-aware Systems and Applications}, volume={4}, number={11}, publisher={EAI}, journal_a={CASA}, year={2017}, month={3}, keywords={Credit risk, Credit scoring, Hybrid Feature selection, GBM, RFE, Information Values, and Machine learning.}, doi={10.4108/eai.6-3-2017.152335} }
- Sang Ha Van
Nam Nguyen Ha
Hien Nguyen Thi Bao
Year: 2017
A hybrid feature selection method for credit scoring
CASA
EAI
DOI: 10.4108/eai.6-3-2017.152335
Abstract
Reliable credit scoring models played a very important role of retail banks to evaluate credit applications and it has been widely studied. The main objective of this paper is to build a hybrid credit scoring model using feature selection approach. In this study, we constructed a credit scoring model based on parallel GBM (Gradient Boosted Model), filter and wrapper approaches to evaluate the applicant’s credit score from the input features. Feature scoring expression are combined by feature important (Gini index) and Information Value. Backward sequential scheme is used for selecting optimal subset of relevant features while the subset is evaluated by GBM classifier. To reduce the running time, we applied parallel GBM classifier to evaluate the proposed subset of features. The experimental results showed that the proposed method obtained a higher predictive accuracy than a baseline method for some certain datasets. It also showed faster speed and better generalization than traditional feature selection methods widely used in credit scoring.
Copyright © 2017 Sang Ha Van et al., licensed to EAI. This is an open access article distributed under the terms of the Creative Commons Attribution licence (http://creativecommons.org/licenses/by/3.0/), which permits unlimited use, distribution and reproduction in any medium so long as the original work is properly cited.