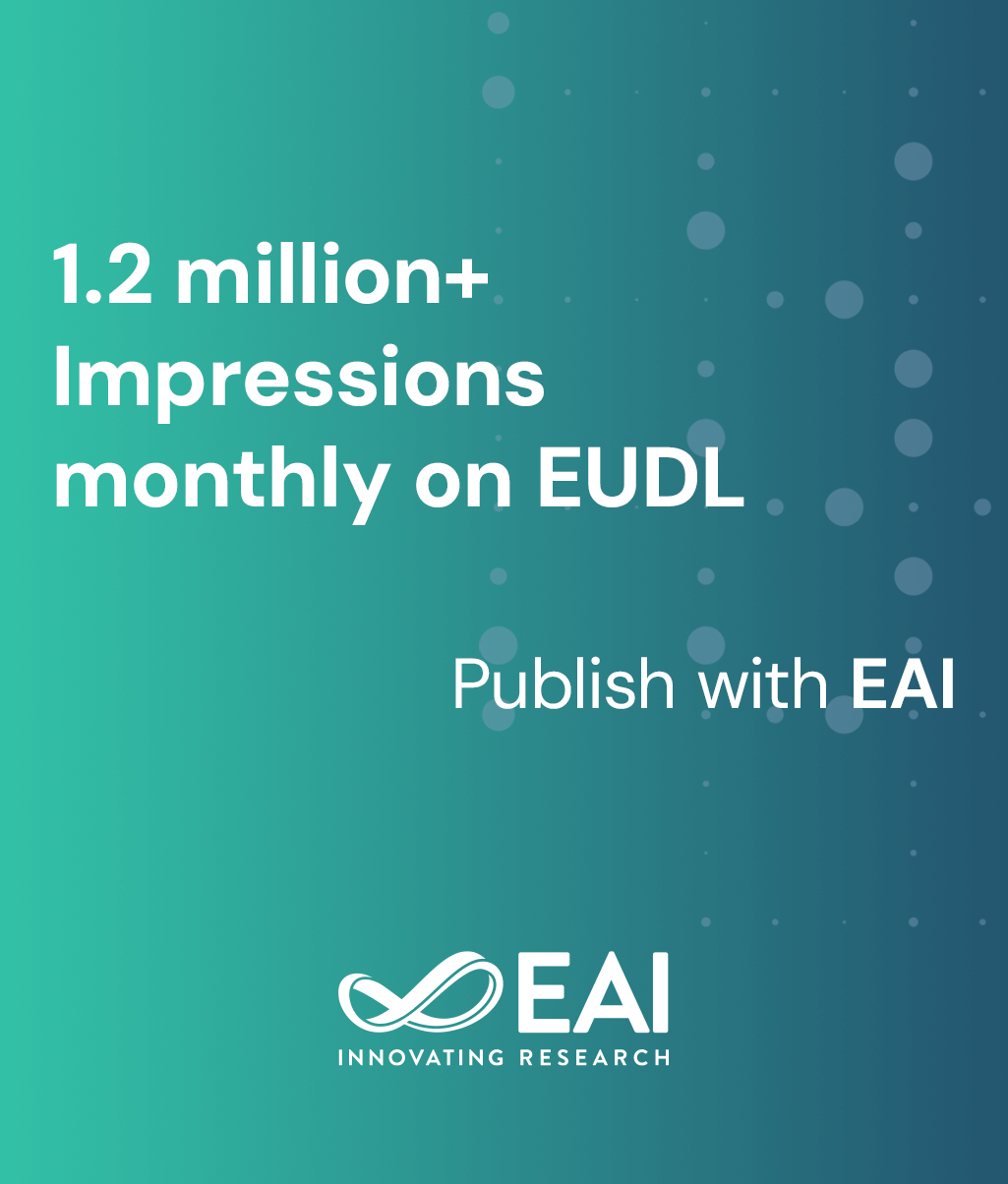
Research Article
Spectrum Sensing For Cognitive Radios Through Differential Entropy
@ARTICLE{10.4108/eai.5-4-2016.151147, author={Sanjeev Gurugopinath and R. Muralishankar and H. N. Shankar}, title={Spectrum Sensing For Cognitive Radios Through Differential Entropy}, journal={EAI Endorsed Transactions on Cognitive Communications}, volume={2}, number={6}, publisher={EAI}, journal_a={COGCOM}, year={2016}, month={4}, keywords={Spectrum sensing, goodness-of-fit, differential entropy, maximum entropy principle, non-Gaussian noise.}, doi={10.4108/eai.5-4-2016.151147} }
- Sanjeev Gurugopinath
R. Muralishankar
H. N. Shankar
Year: 2016
Spectrum Sensing For Cognitive Radios Through Differential Entropy
COGCOM
EAI
DOI: 10.4108/eai.5-4-2016.151147
Abstract
In this work, we present a novel Goodness-of-Fit Test driven by differential entropy for spectrum sensing in cognitive radios, under three different noise models – Gaussian, Laplacian and mixture of Gaussians. We analyze the proposed detector under Gaussian noise which models the worst-case. We then analyze by considering the Laplacian noise process which has tails heavier than that of the Gaussian. We generalize the analysis considering the noise to be a mixture of Gaussians, which is often the case with noise and interference in communication systems. We analyze the performance under each of these cases for a large class of practically relevant fading channel models and primary signal models, with emphasis on low Signal-to-Noise ratio regimes. Towards this end, we derive closed form expressions for the distribution of the test statistic under the null hypothesis and the detection threshold that satisfies a constraint on the probability of false-alarm. Through Monte Carlo simulations, we demonstrate that our detection strategy outperforms an existing spectrum sensing technique based on order statistics.
Copyright © 2016 S. Gurugopinath et al., licensed to EAI. This is an open access article distributed under the terms of the Creative Commons Attribution license (http://creativecommons.org/licenses/by/3.0/), which permits unlimited use, distribution and reproduction in any medium so long as the original work is properly cited.