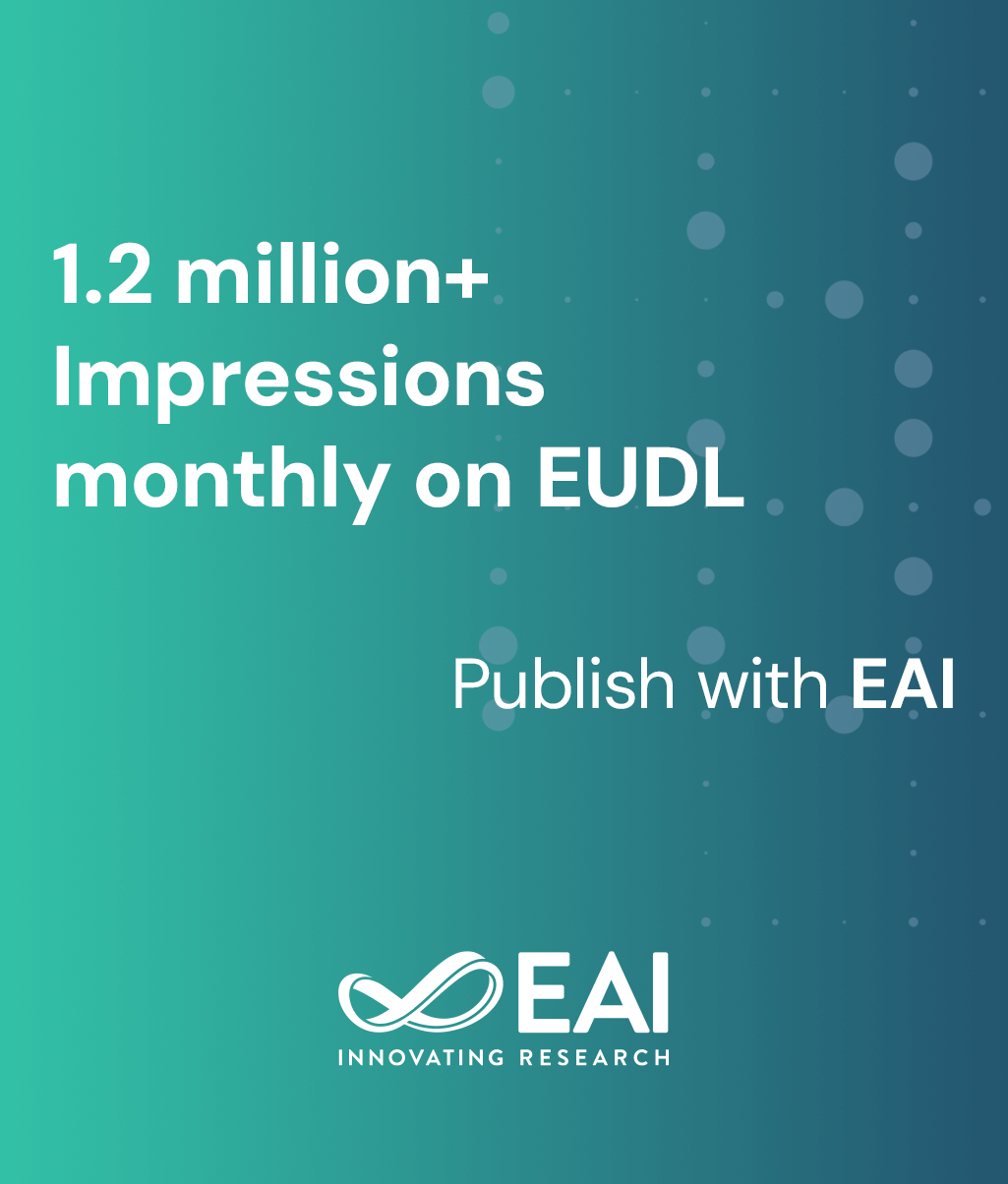
Research Article
Heart Condition Classification using Deep Learning as A Diagnosing Helper
@INPROCEEDINGS{10.4108/eai.5-10-2022.2329543, author={Churun In Layyinah and Mohamad Johan Arifin and Riyanto Sigit and Tita Karlita and Taufiq Hidayat}, title={Heart Condition Classification using Deep Learning as A Diagnosing Helper}, proceedings={Proceedings of the 5th International Conference on Applied Engineering, ICAE 2022, 5 October 2022, Batam, Indonesia}, publisher={EAI}, proceedings_a={ICAE}, year={2023}, month={6}, keywords={heart disease deep learning tracking optical flow classification}, doi={10.4108/eai.5-10-2022.2329543} }
- Churun In Layyinah
Mohamad Johan Arifin
Riyanto Sigit
Tita Karlita
Taufiq Hidayat
Year: 2023
Heart Condition Classification using Deep Learning as A Diagnosing Helper
ICAE
EAI
DOI: 10.4108/eai.5-10-2022.2329543
Abstract
Heart is one of the most vital organ. One of its roles is to pump blood so that the blood can circulate through the body and then receive it after the blood passed the lungs for cleaning. Unfortunately, heart disease is one of the most deadly disease in the world. One of many tools to support heart disease examination is echocardiography. Echocardiography shows the heart’s left ventricular movement so that doctors can see whether the patient is experiencing ischemia or infarction. Sadly, the examination results depend on the doctors’ experience and accuracy. Hence, in this study, a system with the ability to classify human heart conditions based on left ventricle movement are developed. The methods used in the system include optical flow Lucas-Kanade to track heart cavity movement. The features that will be extracted from the process are distance and direction. Distance feature will be calculated using Euclidean distance formula and direction feature will be calculated according to the points’ angle using cosine triangle formula. And at final, after all the feature obtained, the classification will be done using deep learning method. The tracking and feature extraction process is done succesfully. The classification process obtained 71,43% accuracy.