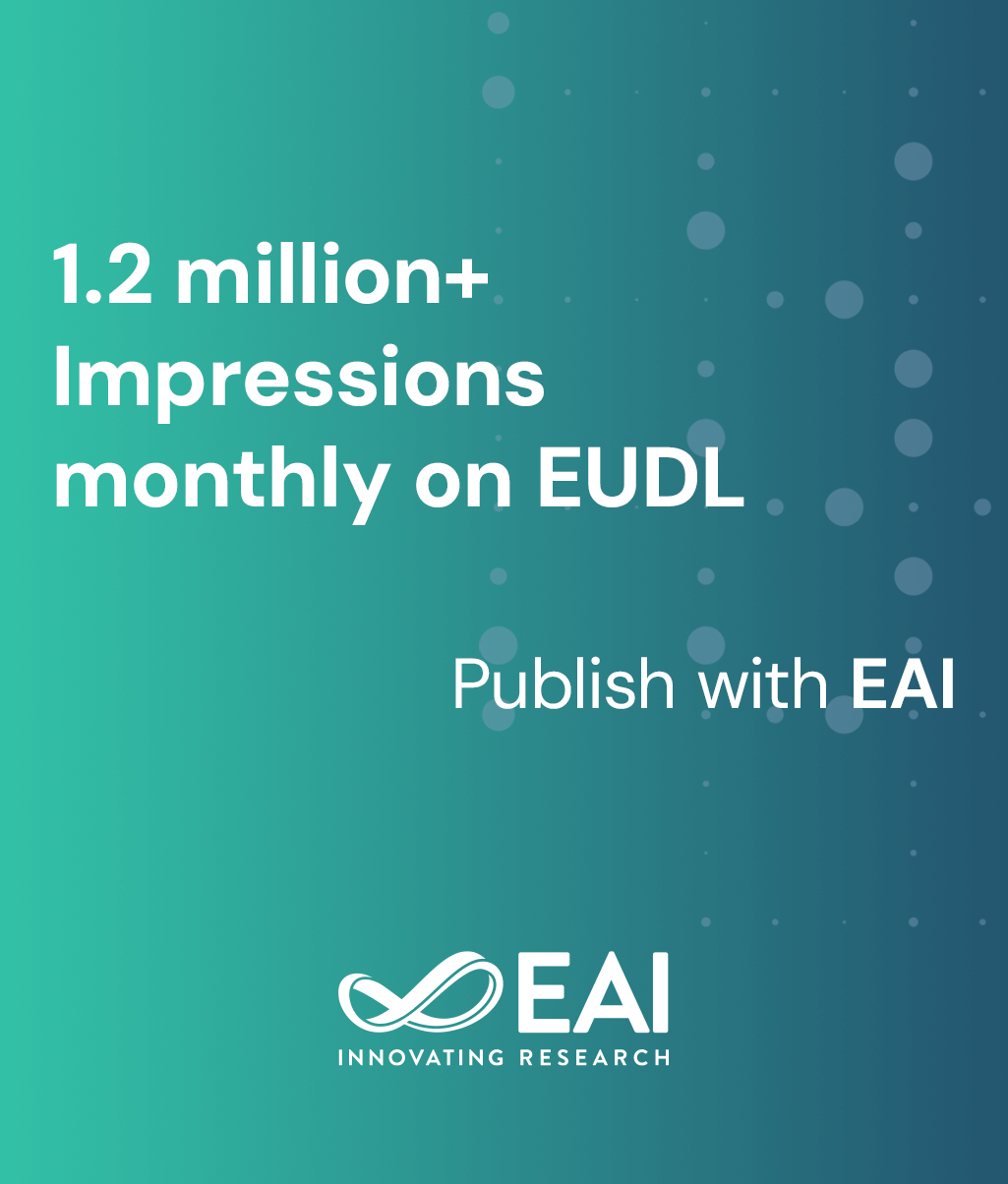
Research Article
Optimization of employee turnover through predictive analysis
@INPROCEEDINGS{10.4108/eai.4-12-2020.2303446, author={Katarina Stachova and Alexandra Barokova and Zdenko Stacho}, title={Optimization of employee turnover through predictive analysis}, proceedings={Management Trends in the Context of Industry 4.0}, publisher={EAI}, proceedings_a={MANAGEMENTTRENDS}, year={2021}, month={11}, keywords={predictive analysis human resources management fluctuation data analysis employee turnover}, doi={10.4108/eai.4-12-2020.2303446} }
- Katarina Stachova
Alexandra Barokova
Zdenko Stacho
Year: 2021
Optimization of employee turnover through predictive analysis
MANAGEMENTTRENDS
EAI
DOI: 10.4108/eai.4-12-2020.2303446
Abstract
In today's technologically driven world, data is essential for decision making process. A comprehensive data analysis is needed to review past practices to improve business performance in the future. The use of data science is found in every prominent domain today and human resources management is one of them. The departure of qualified employees is costly for compa-nies, so it is necessary for them to be able to estimate in advance the proba-bility of their departure and take preventive measures (Jaffara et al., 2019). Once companies have this information, they know how to change their strat-egy to retain key employees or start hiring new employees in time. For com-pany executives, it is important to link such an analysis of employee depar-tures with the creation of a strategy, and thus obtain cost-effective human re-sources management. We focused on predicting employee turnover through the Python programming language. Using a correlation matrix that shows the relationships between the various reasons for leaving and decision trees, we provide a management tool to determine the probability of employee turno-ver. One of the strongest reasons for employee turnover was exhaustion at work, where more than 54% of retired workers worked overtime. Another was income discrepancies between departments. The data analysis points to several differences in the treatment of employees.