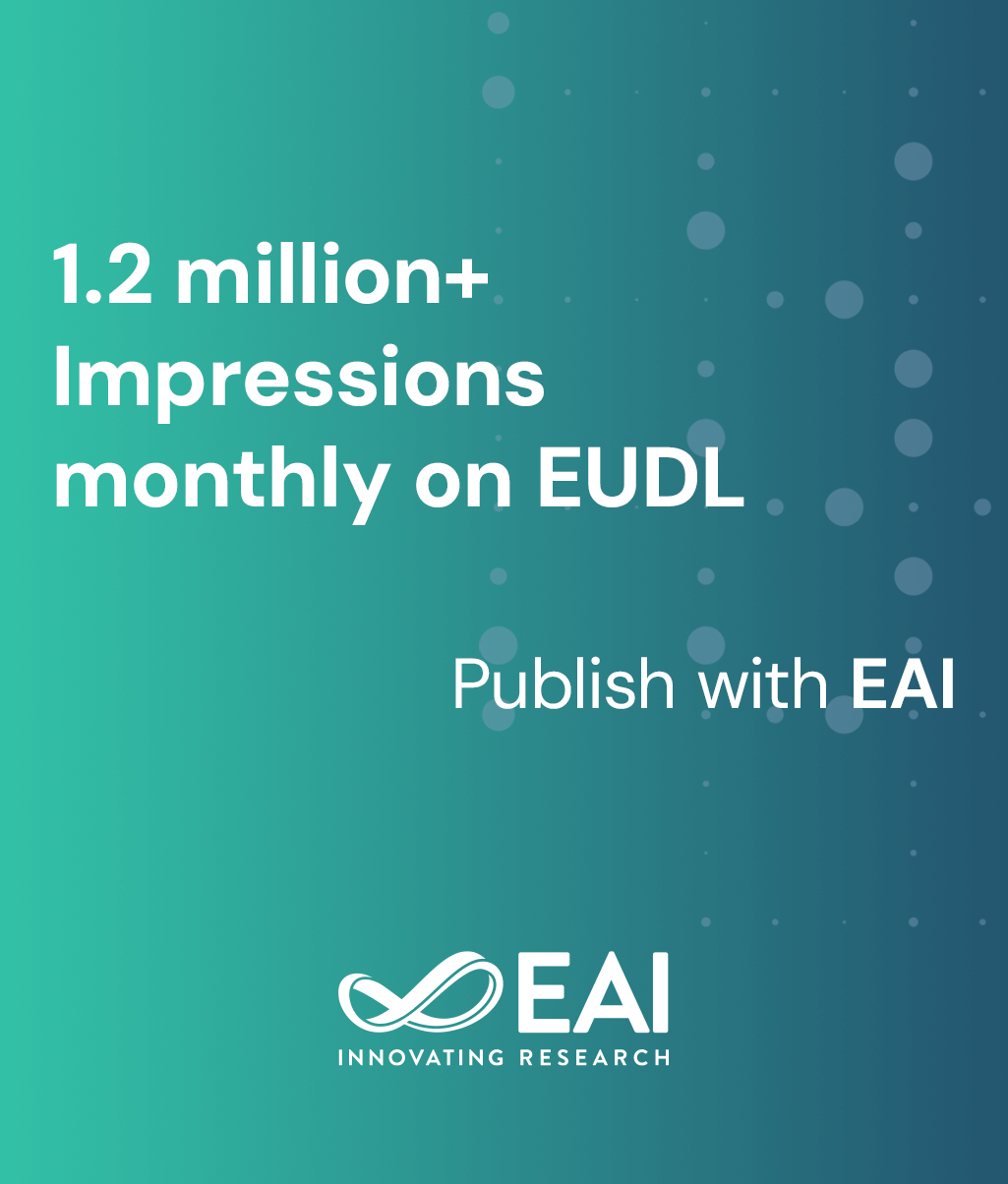
Research Article
Quantifying the Uncertainty of Next-Place Predictions
@INPROCEEDINGS{10.4108/eai.30-11-2016.2267147, author={Paul Baumann and Marc Langheinrich and Anind Dey and Silvia Santini}, title={Quantifying the Uncertainty of Next-Place Predictions}, proceedings={The 8th EAI International Conference on Mobile Computing, Applications and Services}, publisher={ACM}, proceedings_a={MOBICASE}, year={2016}, month={12}, keywords={human mobility prediction uncertainty quantification analysis of mobile phone data sets}, doi={10.4108/eai.30-11-2016.2267147} }
- Paul Baumann
Marc Langheinrich
Anind Dey
Silvia Santini
Year: 2016
Quantifying the Uncertainty of Next-Place Predictions
MOBICASE
ACM
DOI: 10.4108/eai.30-11-2016.2267147
Abstract
Context-aware systems use predictions about a user's future state (e.g., next movements, actions, or needs) in order to seamlessly trigger the display of information or the execution of services. Predictions, however, always have an associated uncertainty that, when above a certain threshold, should prevent a system from taking action due to the risk of getting it wrong''. In this work, we present a context-dependent
level of trust'' estimator that is able to determine whether a prediction should be trusted -- and thus used to trigger an action -- or not. Our estimator relies on ensemble learning to adapt across different users and application scenarios. We demonstrate its performance in the context of a popular problem -- next-place prediction -- and show how it outperforms existing approaches. We also report on the results of a survey that investigated user attitudes towards mobile-phone-based personal assistants and their ability to trigger actions in response to predictions. While users appreciated such assistants, they had substantially different tolerance thresholds with respect to prediction errors depending on the use case. This further motivates the need for a context-dependent level of trust estimator.