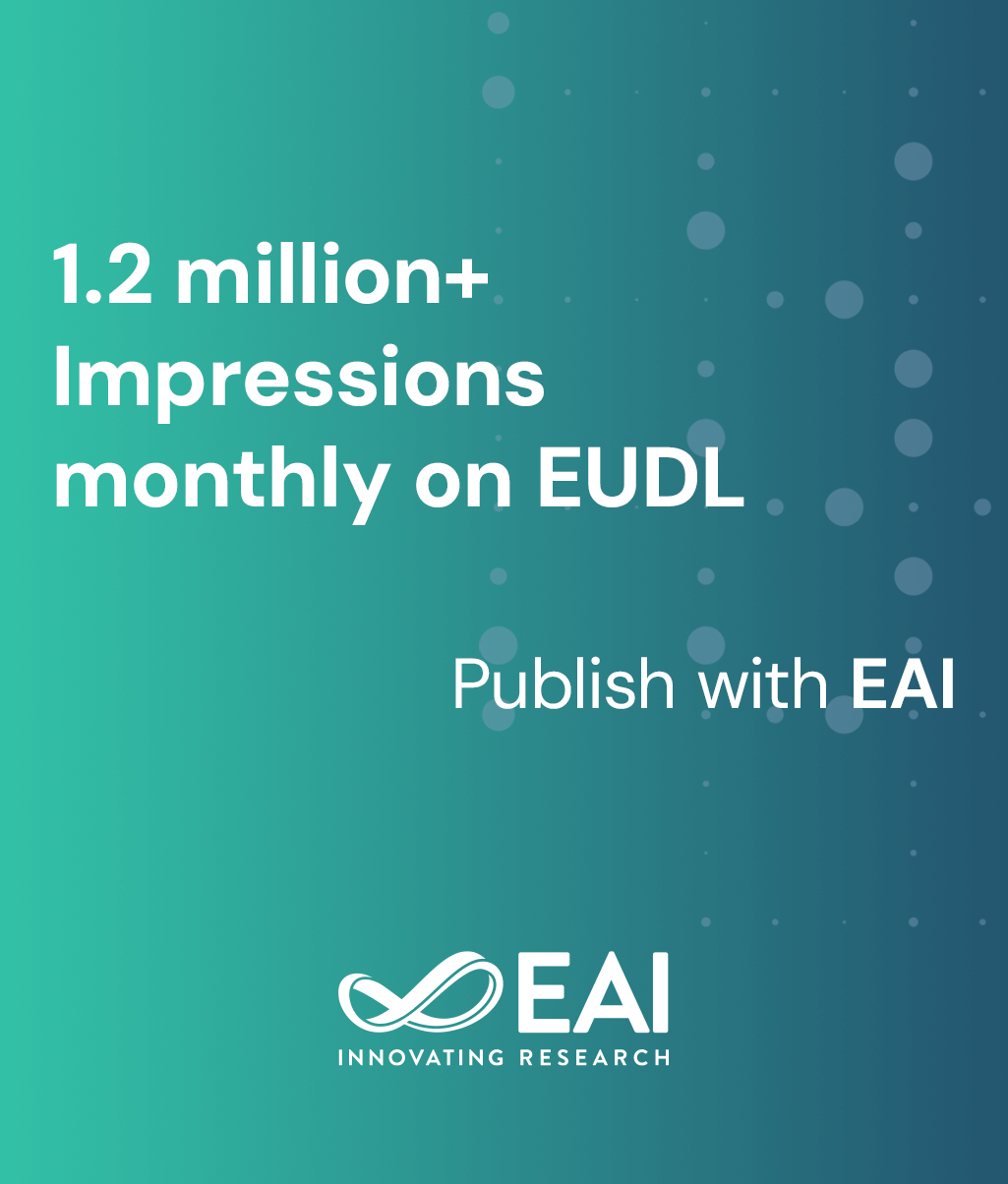
Research Article
A Large-Scale Study in Predictability of Daily Activities and Places
@INPROCEEDINGS{10.4108/eai.30-11-2016.2267041, author={Gordon Moon and Jihun Hamm}, title={A Large-Scale Study in Predictability of Daily Activities and Places}, proceedings={The 8th EAI International Conference on Mobile Computing, Applications and Services}, publisher={ACM}, proceedings_a={MOBICASE}, year={2016}, month={12}, keywords={daily activity prediction daily place prediction sequential model recurrent neural network lstm}, doi={10.4108/eai.30-11-2016.2267041} }
- Gordon Moon
Jihun Hamm
Year: 2016
A Large-Scale Study in Predictability of Daily Activities and Places
MOBICASE
ACM
DOI: 10.4108/eai.30-11-2016.2267041
Abstract
Modeling human activity has a wide range of applications, including customized service for mobile users and resource management for mobile communications. Predicting future activities and places is a challenging task, as the activity pattern shows both regularity and randomness simultaneously. Time information such as time-of-day or day-of-week can partially explain this regularity. Furthermore, current activity and place may depend strongly on the activity and place history far back into the past. To capture this long-range temporal dependency, we used Long Short-Term Memory (LSTM) network, a state-of-the-art sequential model. Instead of predicting a future state of the immediate next step, we proposed multi-step look-ahead predictions to evaluate how far we could accurately predict future states up to 6 hours. Experiments on large-scale American Time Use Survey dataset showed that our model outperforms previous approaches, including Markov-based models, consistently achieving 0.3-7% relative improvement. We divided subjects into four groups by employment status, and we showed qualitatively that daily activity patterns are different among groups, and quantitatively that group-specific training is essential to accurately predict the future activity of individuals.