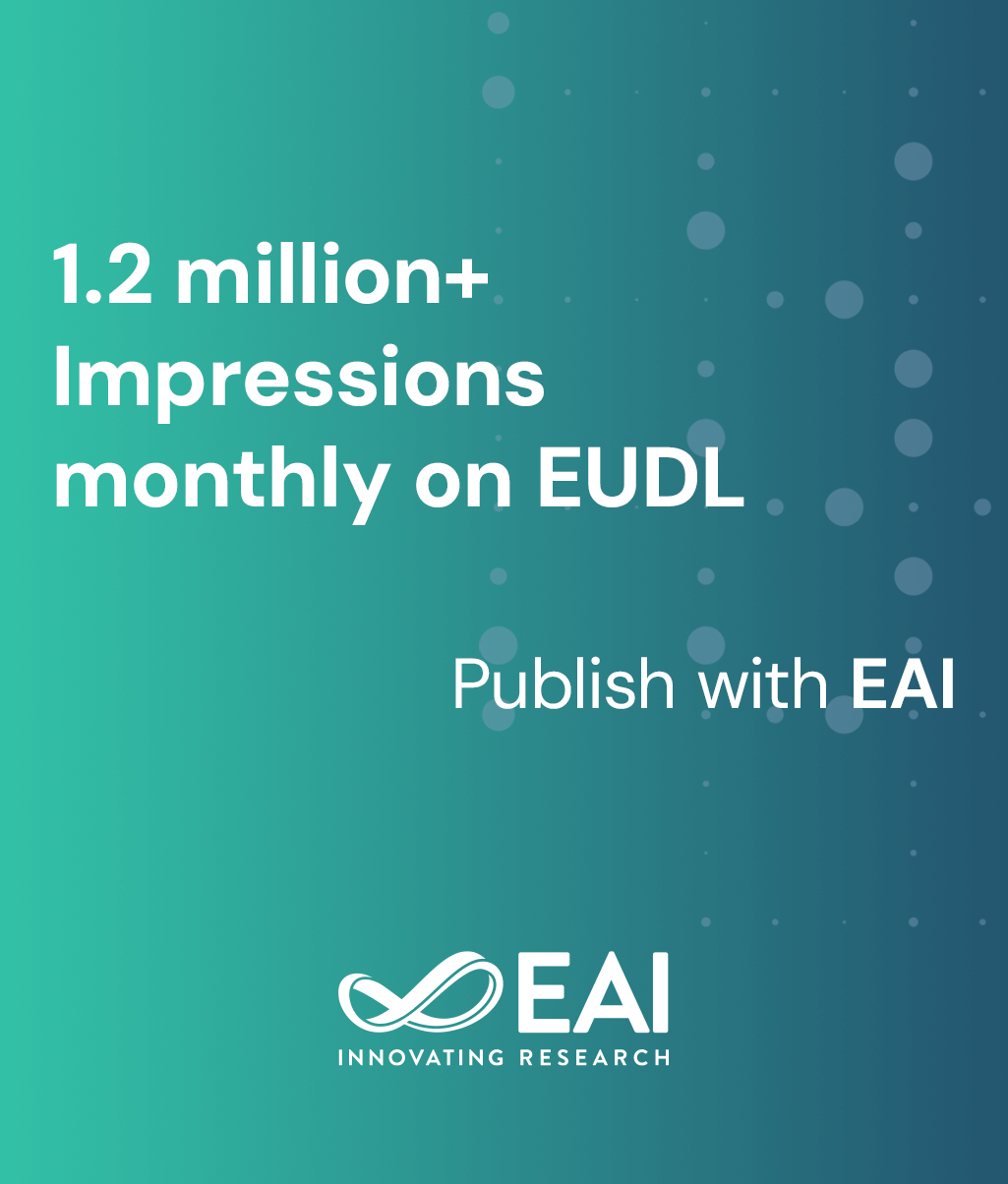
Research Article
Reinforcement Learning with Internal Reward for Multi-Agent Cooperation: A Theoretical Approach
@ARTICLE{10.4108/eai.3-12-2015.2262878, author={Fumito Uwano and Naoki Tatebe and Masaya Nakata and Keiki Takadama and Tim Kovacs}, title={Reinforcement Learning with Internal Reward for Multi-Agent Cooperation: A Theoretical Approach}, journal={EAI Endorsed Transactions on Collaborative Computing}, volume={2}, number={8}, publisher={ACM}, journal_a={CC}, year={2016}, month={5}, keywords={multi-agent system, analysis, q-learning, internal reward}, doi={10.4108/eai.3-12-2015.2262878} }
- Fumito Uwano
Naoki Tatebe
Masaya Nakata
Keiki Takadama
Tim Kovacs
Year: 2016
Reinforcement Learning with Internal Reward for Multi-Agent Cooperation: A Theoretical Approach
CC
EAI
DOI: 10.4108/eai.3-12-2015.2262878
Abstract
This paper focuses on a multi-agent cooperation which is generally difficult to be achieved without sufficient information of other agents, and proposes the reinforcement learning method that introduces an internal reward for a multi-agent cooperation without sufficient information. To guarantee to achieve such a cooperation, this paper theoretically derives the condition of selecting appropriate actions by changing internal rewards given to the agents, and extends the reinforcement learning methods (Q-learning and Profit Sharing) to enable the agents to acquire the appropriate Q-values updated according to the derived condition. Concretely, the internal rewards change when the agents can only find better solution than the current one. The intensive simulations on the maze problems as one of testbeds have revealed the following implications:(1) our proposed method successfully enables the agents to select their own appropriate cooperating actions which contribute to acquiring the minimum steps towards to their goals, while the conventional methods (i.e., Q-learning and Profit Sharing) cannot always acquire the minimum steps; and (2) the proposed method based on Profit Sharing provides the same good performance as the proposed method based on Q-learning.
Copyright © 2015 F. Uwano et al., licensed to EAI. This is an open access article distributed under the terms of the Creative Commons Attribution licence (http://creativecommons.org/licenses/by/3.0/), which permits unlimited use, distribution and reproduction in any medium so long as the original work is properly cited.