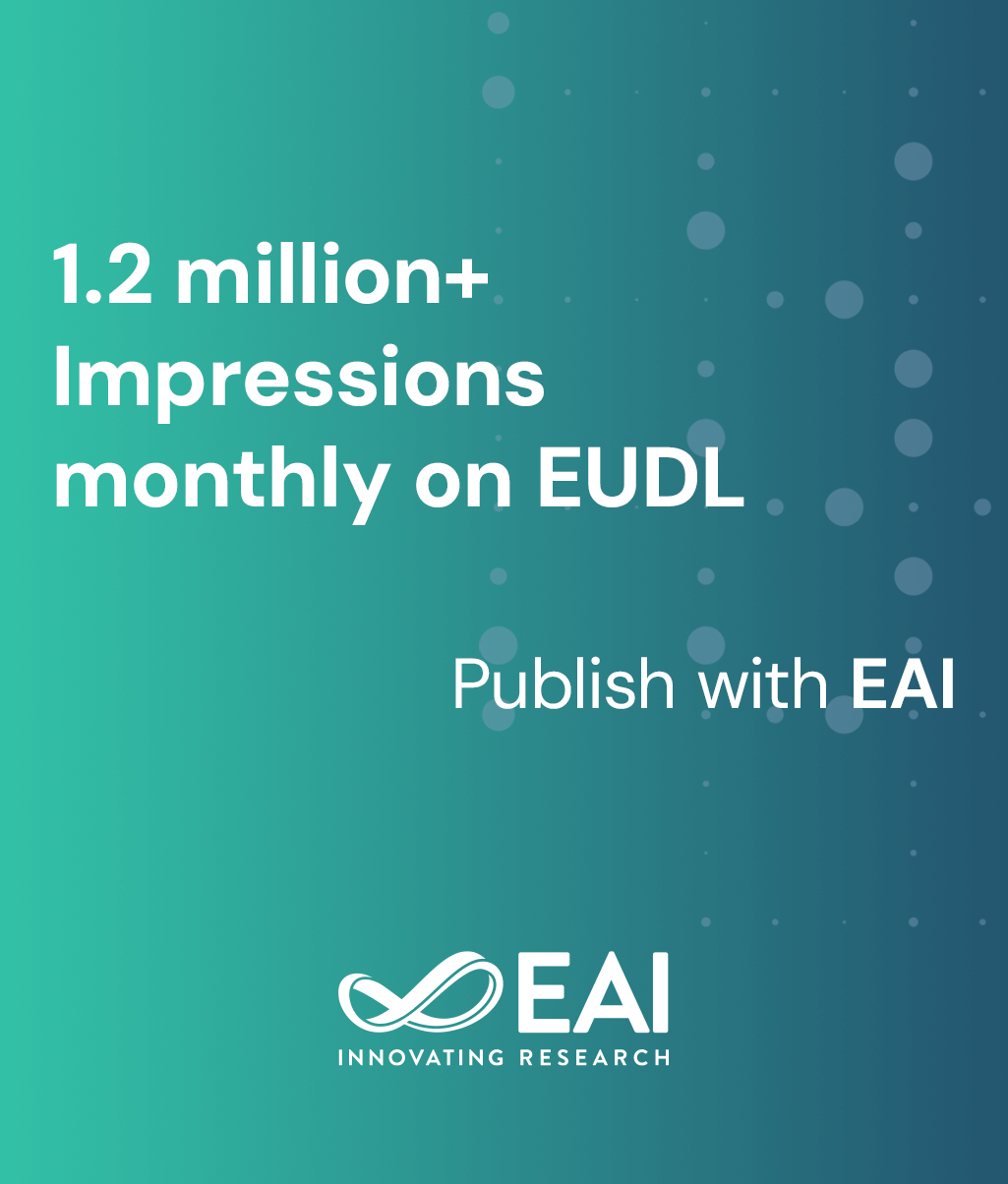
Research Article
Gist+RatSLAM: An Incremental Bio-inspired Place Recognition Front-End for RatSLAM
@ARTICLE{10.4108/eai.3-12-2015.2262532, author={S. M. Ali Musa Kazmi and B\aa{}rbel Mertsching}, title={Gist+RatSLAM: An Incremental Bio-inspired Place Recognition Front-End for RatSLAM}, journal={EAI Endorsed Transactions on Creative Technologies}, volume={3}, number={8}, publisher={ACM}, journal_a={CT}, year={2016}, month={5}, keywords={gist, bio-inspired mapping, scene recognition, global image feature, self-organizing neural network, competitive learning}, doi={10.4108/eai.3-12-2015.2262532} }
- S. M. Ali Musa Kazmi
Bärbel Mertsching
Year: 2016
Gist+RatSLAM: An Incremental Bio-inspired Place Recognition Front-End for RatSLAM
CT
EAI
DOI: 10.4108/eai.3-12-2015.2262532
Abstract
There exists ample research exploiting cognitive processes for robot localization and mapping, for instance RatSLAM [10]. In this regard, tasks such as visual perception and recognition, which are primarily governed by visual and perirhinal cortices, receive a little attention. To bridge this gap, we present a novel bio-inspired place recognition front-end for the RatSLAM system. Our algorithm uses Gist features to obtain the perceptual structure of the scenes and employs a modified growing self-organizing map (GSOM) to model the behavior of the cells found in perirhinal cortex, called recency and familiarity neurons [6]. This enables an online learning and recognition of the places without acquiring apriori knowledge of the environment. The experiments carried out on the standard St. Lucia dataset demonstrate that on average our approach achieves almost 10% improvement (in F1-Score); it is able to correctly flag the visited and unvisited places even for noisy and blurred visual inputs. The results show that the proposed method reaches fast convergence and utilizes a smaller number of cells (consumes less physical memory) to represent the traversed path compared to the RatSLAM approach.
Copyright © 2015 S. M. A. M. Kazmi et al., licensed to EAI. This is an open access article distributed under the terms of the Creative Commons Attribution licence (http://creativecommons.org/licenses/by/3.0/), which permits unlimited use, distribution and reproduction in any medium so long as the original work is properly cited.