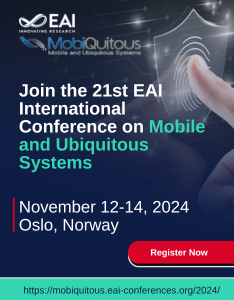
Research Article
A Deep Learning Approach for Network Intrusion Detection System
@ARTICLE{10.4108/eai.3-12-2015.2262516, author={Ahmad Javaid and Quamar Niyaz and Weiqing Sun and Mansoor Alam}, title={A Deep Learning Approach for Network Intrusion Detection System}, journal={EAI Endorsed Transactions on Security and Safety}, volume={3}, number={9}, publisher={ACM}, journal_a={SESA}, year={2016}, month={5}, keywords={network security, nids, deep learning, sparse autoencoder, nsl-kdd}, doi={10.4108/eai.3-12-2015.2262516} }
- Ahmad Javaid
Quamar Niyaz
Weiqing Sun
Mansoor Alam
Year: 2016
A Deep Learning Approach for Network Intrusion Detection System
SESA
EAI
DOI: 10.4108/eai.3-12-2015.2262516
Abstract
A Network Intrusion Detection System (NIDS) helps system administrators to detect network security breaches in their organizations. However, many challenges arise while developing a flexible and efficient NIDS for unforeseen and unpredictable attacks. We propose a deep learning based approach for developing such an efficient and flexible NIDS. We use Self-taught Learning (STL), a deep learning based technique, on NSL-KDD - a benchmark dataset for network intrusion. We present the performance of our approach and compare it with a few previous work. Compared metrics include accuracy, precision, recall, and f-measure values.
Copyright © 2015 A. Javaid et al., licensed to EAI. This is an open access article distributed under the terms of the Creative Commons Attribution licence (http://creativecommons.org/licenses/by/3.0/), which permits unlimited use, distribution and reproduction in any medium so long as the original work is properly cited.