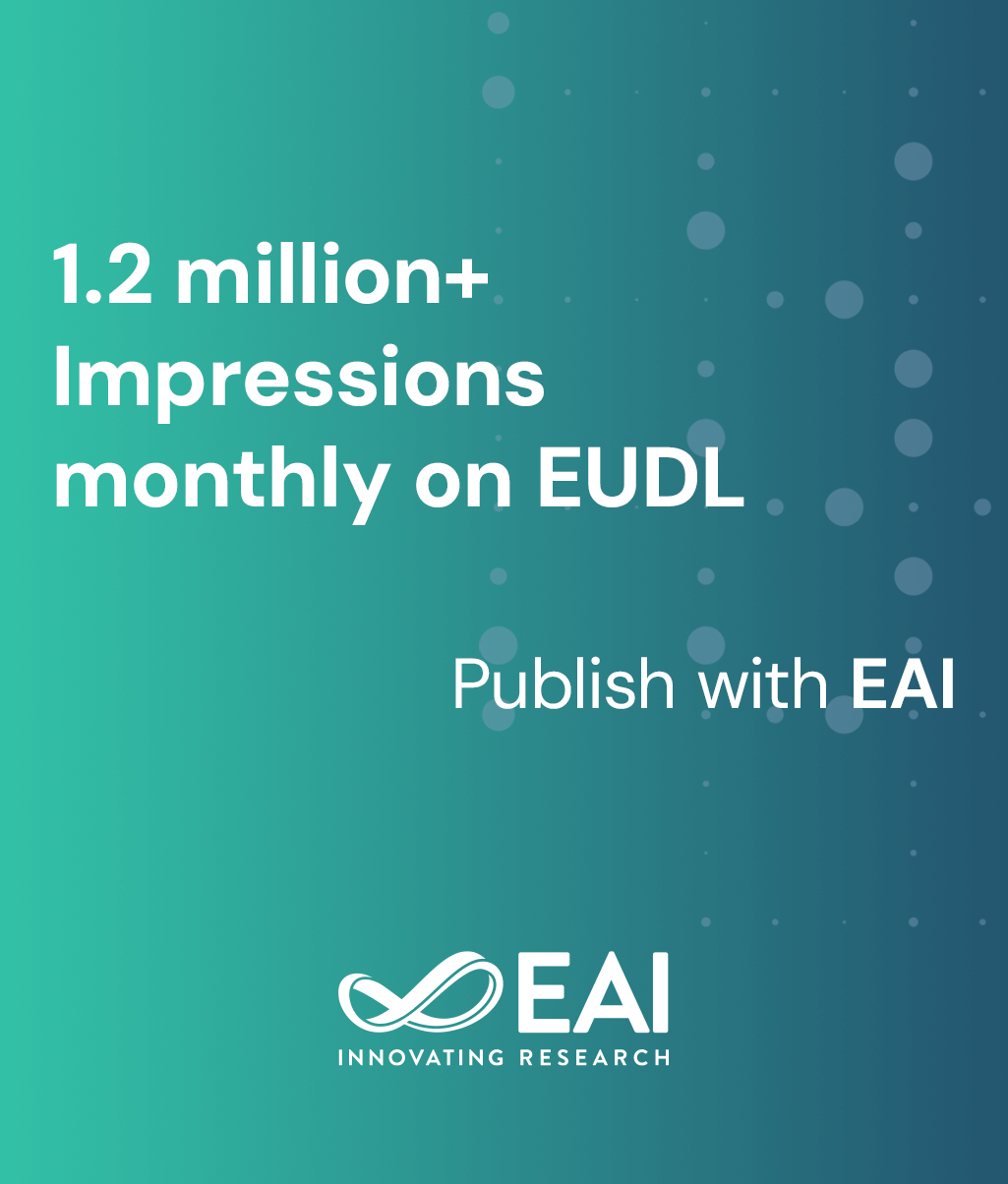
Research Article
Proposing Multimodal Integration Model Using LSTM and Autoencoder
@INPROCEEDINGS{10.4108/eai.3-12-2015.2262505, author={Wataru Noguchi and Hiroyuki Iizuka and Masahito Yamamoto}, title={Proposing Multimodal Integration Model Using LSTM and Autoencoder}, proceedings={9th EAI International Conference on Bio-inspired Information and Communications Technologies (formerly BIONETICS)}, publisher={ACM}, proceedings_a={BICT}, year={2016}, month={5}, keywords={multimodal integration deep learning autoencoder long short term memory}, doi={10.4108/eai.3-12-2015.2262505} }
- Wataru Noguchi
Hiroyuki Iizuka
Masahito Yamamoto
Year: 2016
Proposing Multimodal Integration Model Using LSTM and Autoencoder
BICT
EAI
DOI: 10.4108/eai.3-12-2015.2262505
Abstract
We propose an architecture of neural network that can learn and integrate sequential multimodal information using Long Short Term Memory. Our model consists of encoder and decoder LSTMs and multimodal autoencoder. For integrating sequential multimodal information, firstly, the encoder LSTM encodes a sequential input to a fixed range feature vector for each modality. Secondly, the multimodal autoencoder integrates the feature vectors from each modality and generate a fused feature vector which contains sequential multimodal information in a mixed form. The original feature vectors from each modality are re-generated from the fused feature vector in the multimodal autoencoder. The decoder LSTM decodes the sequential inputs from the regenerated feature vector. Our model is trained with the visual and motion sequences of humans and is tested by recall tasks. The experimental results show that our model can learn and remember the sequential multimodal inputs and decrease the ambiguity generated at the learning stage of LSTMs using integrated multimodal information. Our model can also recall the visual sequences from the only motion sequences and vice versa.