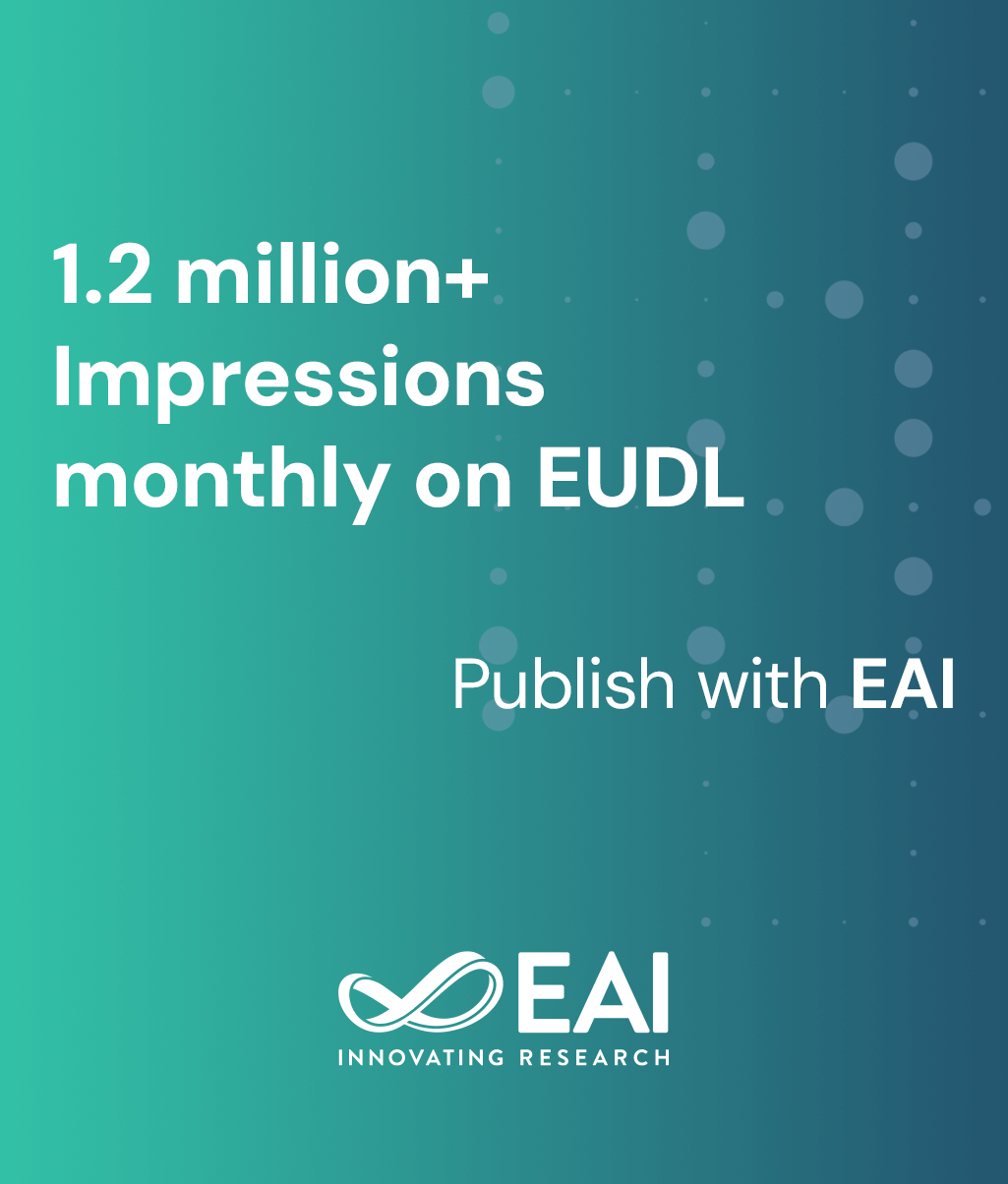
Research Article
Towards a mesoscopic-level canonical circuit definition for visual cortical processing
@INPROCEEDINGS{10.4108/eai.3-12-2015.2262448, author={Georg Layher and Tobias Brosch and Heiko Neumann}, title={Towards a mesoscopic-level canonical circuit definition for visual cortical processing}, proceedings={The First International Workshop on Computational Models of the Visual Cortex: Hierarchies, Layers, Sparsity, Saliency and Attention}, publisher={ACM}, proceedings_a={CMVC}, year={2016}, month={5}, keywords={visual cortex canonical circuit model cortical dynamics cortical column unsupervised learning}, doi={10.4108/eai.3-12-2015.2262448} }
- Georg Layher
Tobias Brosch
Heiko Neumann
Year: 2016
Towards a mesoscopic-level canonical circuit definition for visual cortical processing
CMVC
ACM
DOI: 10.4108/eai.3-12-2015.2262448
Abstract
The mammalian cortex is organized into different layers which are characterized by different cell clusterings and prominent lateral fiber connections. As a primary organizational principle driving signal inputs enter mainly at layer IV, feedforward output activations leave at superficial, while modulating feedback signals leave at deep layers. Likewise, modulatory input signals from other areas mainly enter in superficial layers. Based on such compartmental structure we suggest an abstract formulation of composite structural elements which form building blocks to define canonical elements for columnar computation in cortex. There is evidence that the brain makes use of an operational set of canonical computations, like, e.g., sensory signal filtering, reentrant response gain enhancement, noise suppression, and normalization. As a further abstraction, we define a dynamical three-stage model of processing in a cortical column for processing that allows to investigate their dynamic response properties. Some examples of the analysis of the dynamics are presented together with some simulation results that highlight properties of visual processing in model cortices. Finally, we demonstrate how learning mechanisms that adapt the connection weights can be incorporated in such a scheme capable to form feature representations and functional sub-networks in an adaptive fashion.