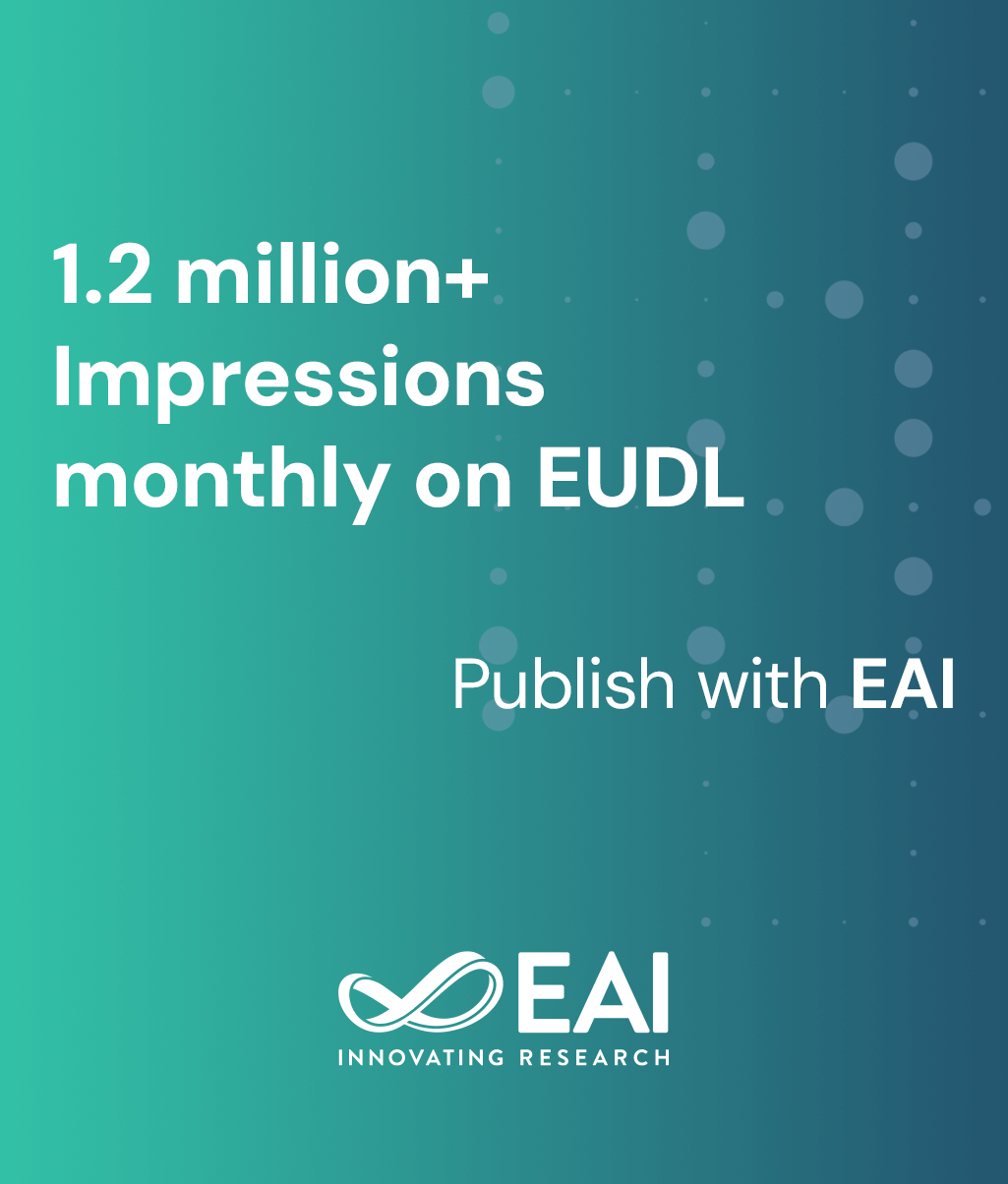
Research Article
Event-based optical flow on neuromorphic hardware
@INPROCEEDINGS{10.4108/eai.3-12-2015.2262447, author={Tobias Brosch and Heiko Neumann}, title={Event-based optical flow on neuromorphic hardware}, proceedings={The First International Workshop on Computational Models of the Visual Cortex: Hierarchies, Layers, Sparsity, Saliency and Attention}, publisher={ACM}, proceedings_a={CMVC}, year={2016}, month={5}, keywords={optical flow event-based sensing address-event representation real-time vision neuromorphic computing}, doi={10.4108/eai.3-12-2015.2262447} }
- Tobias Brosch
Heiko Neumann
Year: 2016
Event-based optical flow on neuromorphic hardware
CMVC
ACM
DOI: 10.4108/eai.3-12-2015.2262447
Abstract
Event-based sensing, i.e. the asynchronous detection of luminance changes, promises low-energy, high dynamic range, and sparse sensing. This stands in contrast to whole image frame-wise acquisition using standard cameras. Recently, we proposed a novel biologically inspired efficient motion detector for such event-based input streams and demonstrated how a canonical neural circuit can improve such representations using normalization and feedback. In this contribution, we suggest how such a motion detection scheme is defined by utilizing a canonical neural circuit corresponding to the resolution of cortical columns. In addition, we develop a mapping of key computational elements of this circuit model onto neuromorphic hardware. In particular, we focus on the recently developed TrueNorth chip architecture by IBM to realize a real-time, energy-efficient and adjustable neuromorphic optical flow detector. We demonstrate the function of the computations of the canonical model and its approximate neuromorphic realization.