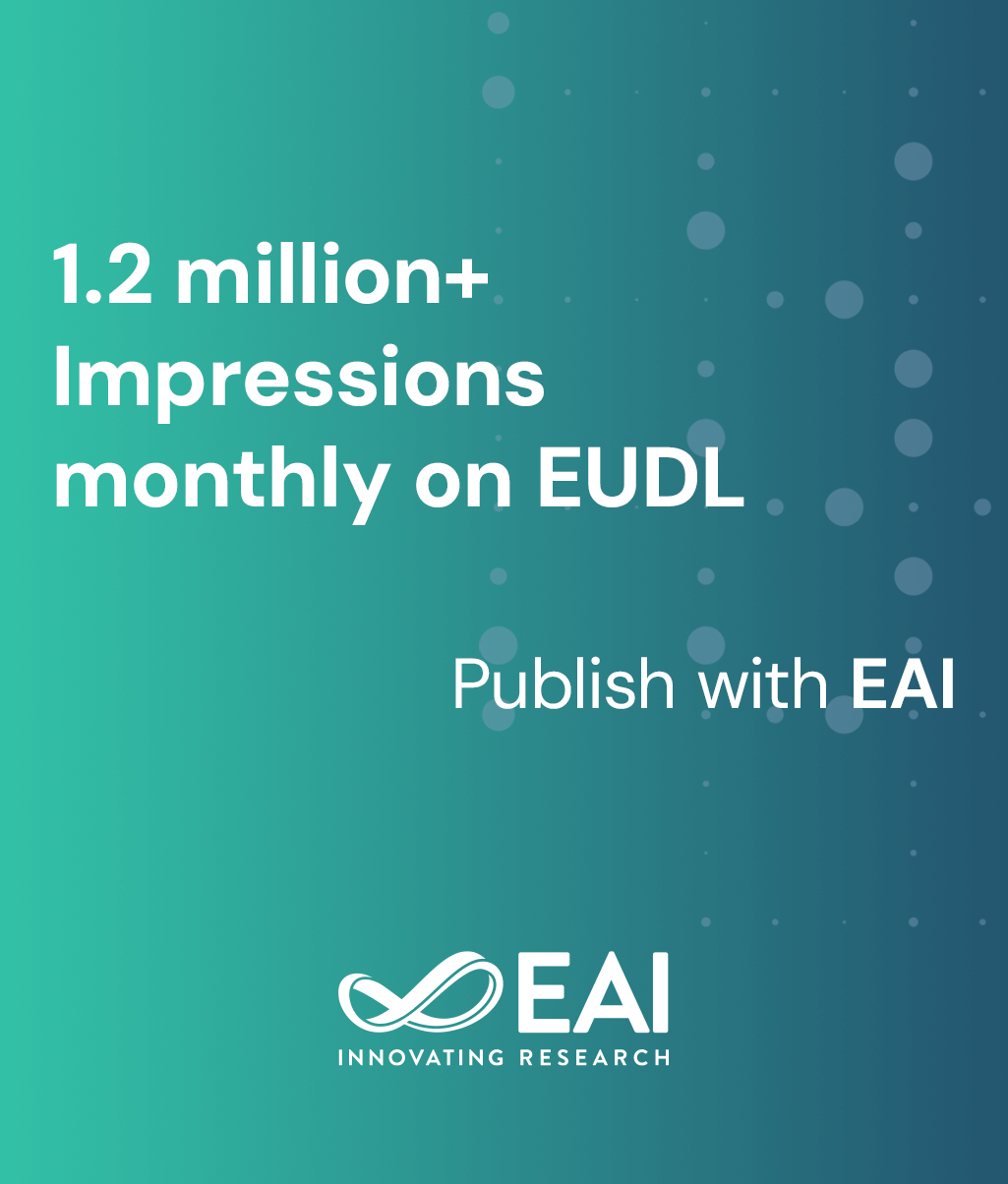
Research Article
Bio-inspired Computation Approach for Tumor Growth with Spatial Randomness Analysis of Kidney Cancer Xenograft Pathology Slides
@INPROCEEDINGS{10.4108/eai.3-12-2015.2262350, author={Aydin Saribudak and Yiyu Dong and James Hsieh and M. Umit Uyar}, title={Bio-inspired Computation Approach for Tumor Growth with Spatial Randomness Analysis of Kidney Cancer Xenograft Pathology Slides}, proceedings={9th EAI International Conference on Bio-inspired Information and Communications Technologies (formerly BIONETICS)}, publisher={ACM}, proceedings_a={BICT}, year={2016}, month={5}, keywords={artificial intelligence bio-inspired computation differential evolution kidney cancer h\&e slide pathology exponential linear tumor growth model spatial pattern analysis}, doi={10.4108/eai.3-12-2015.2262350} }
- Aydin Saribudak
Yiyu Dong
James Hsieh
M. Umit Uyar
Year: 2016
Bio-inspired Computation Approach for Tumor Growth with Spatial Randomness Analysis of Kidney Cancer Xenograft Pathology Slides
BICT
EAI
DOI: 10.4108/eai.3-12-2015.2262350
Abstract
In this paper, we analyze digitized images of Hematoxylin-Eosin (H&E) slides equipped with tumorous tissues from patient derived xenograft models to build our bio-inspired computation method, namely Personalized Relevance Parameterization of Spatial Randomness (PReP-SR). Applying spatial pattern analysis techniques of quadrat counts, kernel estimation and nearest neighbor functions to the images of the H&E samples, slide-specific features are extracted to examine the hypothesis that existence of dependency of nuclei positions possesses information of individual tumor characteristics. These features are then used as inputs to PReP-SR to compute tumor growth parameters for exponential-linear model. Differential evolution algorithms are developed for tumor growth parameter computations, where a candidate vector in a population consists of size selection indices for spatial evaluation and weight coefficients for spatial features and their correlations. Using leave-one-out-cross-validation method, we showed that, for a set of H&E slides from kidney cancer patient derived xenograft models, PReP-SR generates personalized model parameters with an average error rate of 13.58%. The promising results indicate that bio-inspired computation techniques may be useful to construct mathematical models with patient specific growth parameters in clinical systems.