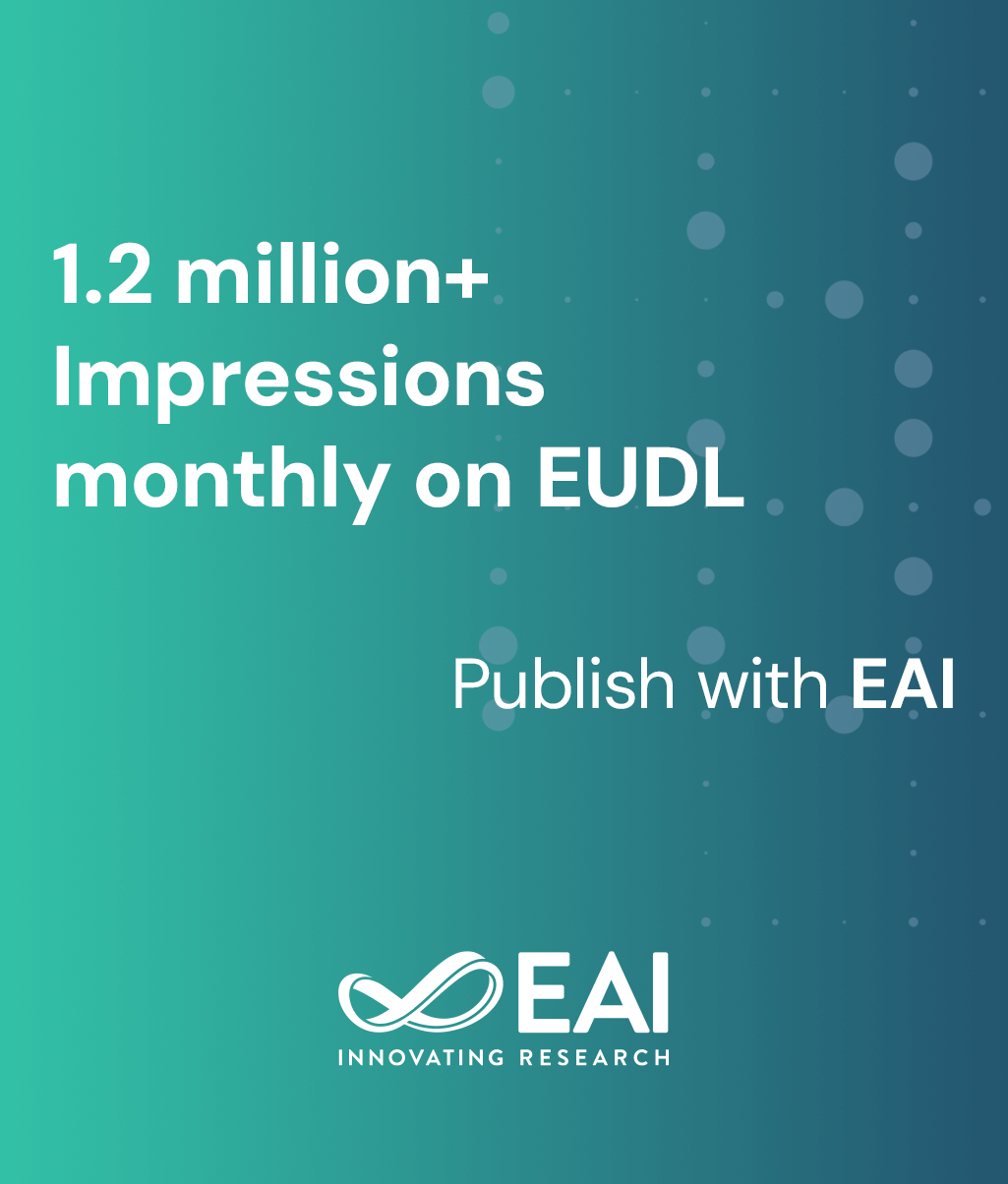
Research Article
Building High-quality Psychology Knowledge Graphs from Text using REBEL
@INPROCEEDINGS{10.4108/eai.29-3-2024.2347315, author={Zeli Chen and Yixuan Chen and Raymond Xu and Yao Zhao}, title={Building High-quality Psychology Knowledge Graphs from Text using REBEL}, proceedings={Proceedings of the 3rd International Conference on Bigdata Blockchain and Economy Management, ICBBEM 2024, March 29--31, 2024, Wuhan, China}, publisher={EAI}, proceedings_a={ICBBEM}, year={2024}, month={6}, keywords={nlp (natural language processing) text-to-knowledge graph rebel relation extraction psychology}, doi={10.4108/eai.29-3-2024.2347315} }
- Zeli Chen
Yixuan Chen
Raymond Xu
Yao Zhao
Year: 2024
Building High-quality Psychology Knowledge Graphs from Text using REBEL
ICBBEM
EAI
DOI: 10.4108/eai.29-3-2024.2347315
Abstract
This paper delves into the progression of Knowledge Graphs since their inception in 1972, highlighting their applications in sectors such as healthcare and finance. We present a novel model that automates knowledge graph creation using NLP technologies like BERT, spaCy, and NLTK. The study also explores three key tool categories in knowledge graph construction: Linguistic, Autoregressive Encoder-Decoder, and BERT-based REBEL, assessing their effectiveness and adaptability. Utilizing advanced algorithms like REBEL and KG-BERT, our system expedites the training process and enhances the quality of Knowledge Graphs. Testing on 20 'Psychology'-focused Wikipedia articles, we achieve near-optimal results in just 8 epochs. The study concludes that pre-training and predictive language models hold significant promise for improving knowledge graph utility and construction.