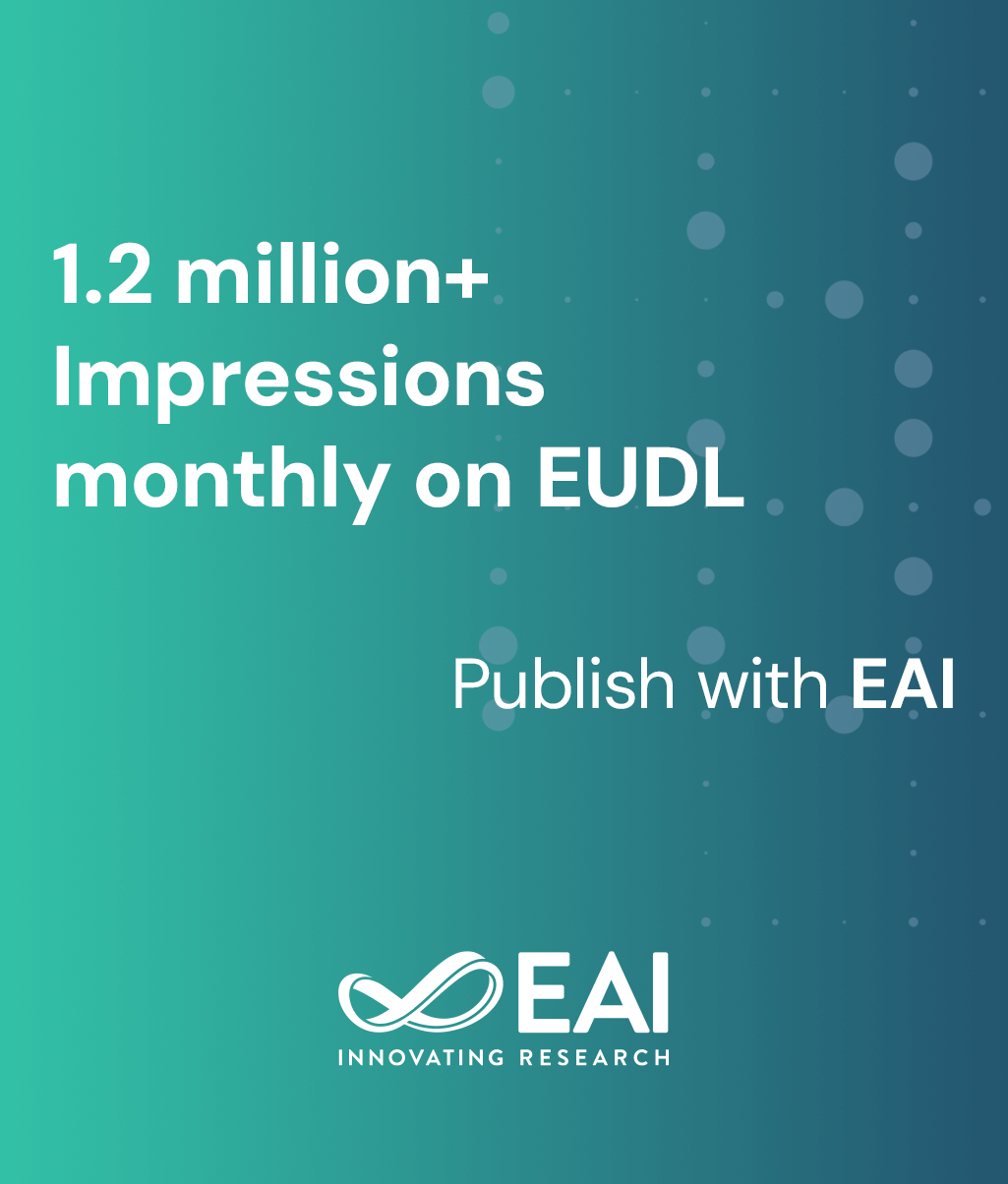
Research Article
Classify Online Customer Reviews from Different Restaurants Based on Sentiment Analysis
@INPROCEEDINGS{10.4108/eai.29-3-2024.2347309, author={Brian Zhou and Qingyang Yang and Lingye Kong}, title={Classify Online Customer Reviews from Different Restaurants Based on Sentiment Analysis}, proceedings={Proceedings of the 3rd International Conference on Bigdata Blockchain and Economy Management, ICBBEM 2024, March 29--31, 2024, Wuhan, China}, publisher={EAI}, proceedings_a={ICBBEM}, year={2024}, month={6}, keywords={sentiment analysis social media restaurant reviews data processing different classifiers}, doi={10.4108/eai.29-3-2024.2347309} }
- Brian Zhou
Qingyang Yang
Lingye Kong
Year: 2024
Classify Online Customer Reviews from Different Restaurants Based on Sentiment Analysis
ICBBEM
EAI
DOI: 10.4108/eai.29-3-2024.2347309
Abstract
It is nearly difficult to manually analyze the data in sentiment analysis since the volume is growing quickly every day. People frequently express their opinions through social media platforms like Tripadvisor and restaurant review sites. Several machine-learning approaches might be used to automate the process of text analysis. Enormous amounts of textual data are produced, yet they are worthless without processing. This paper leverages sentiment analysis to classify customer reviews into three categories: positive, neutral and negative. We used many different classifiers, including Logistic Regression SVM, Random Forest, and Gaussian Naive Bayes, to sort the feedback into appropriate categories. The Random Forest and SVM turned out to have the highest and similar accuracy for the prediction of text data. Logistic regression has a very close but relatively lower accuracy in comparison, and Gaussian Naive Bayes yielded the lowest accuracy. In the end, we use a majority voting ensemble classifier which combines three classifier predictions with the most accuracy. We assessed their performance using a cross-validation technique, comparing their accuracy, precision, recall, and F1-score on the test set. The Majority voting turned out not to have a significant increase in accuracy.