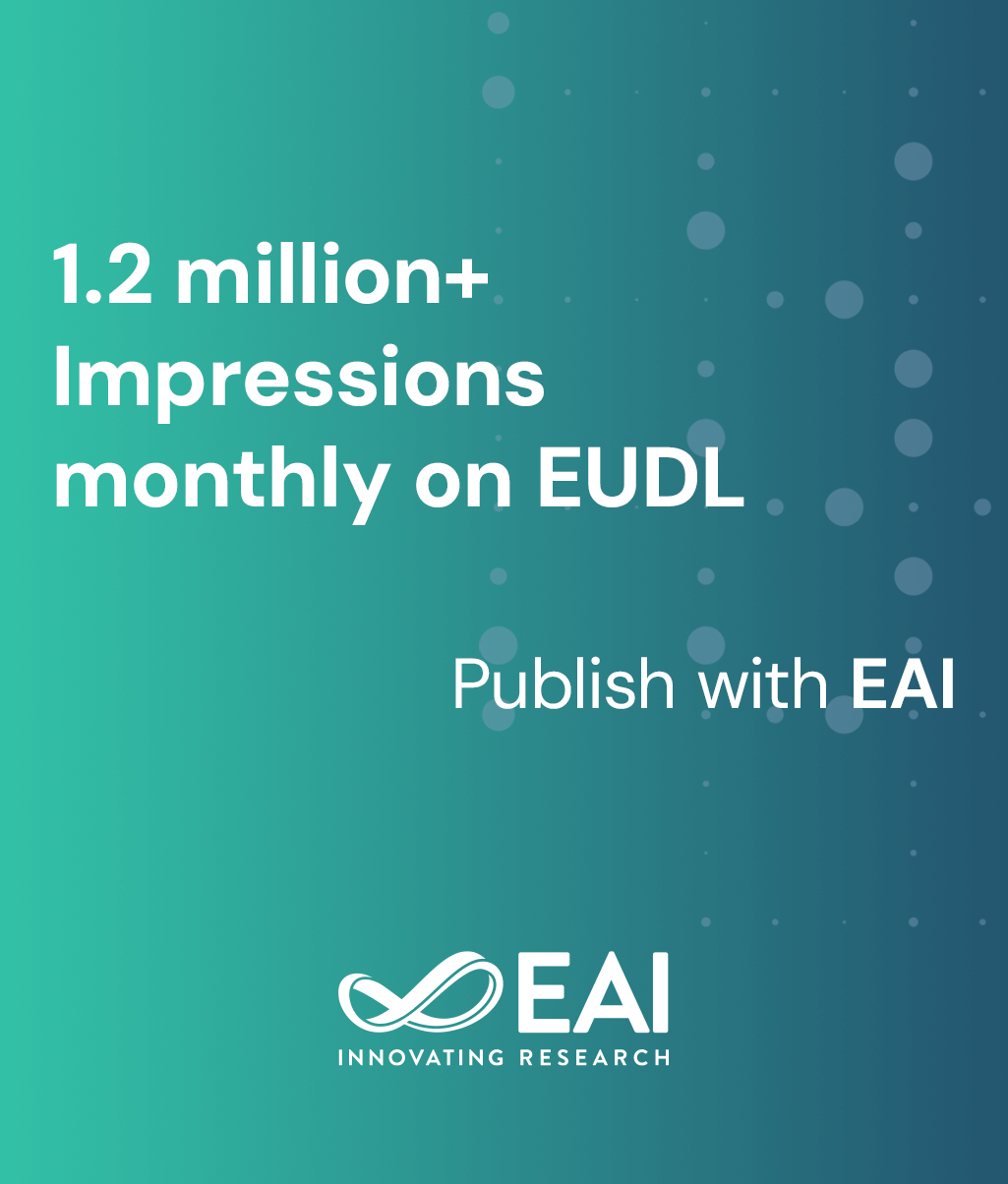
Research Article
A smart phone based gait monitor system
@ARTICLE{10.4108/eai.28-9-2015.2261519, author={Dong Qin and Ming-Chun Huang}, title={A smart phone based gait monitor system}, journal={EAI Endorsed Transactions on Pervasive Health and Technology}, volume={2}, number={7}, publisher={ACM}, journal_a={PHAT}, year={2015}, month={12}, keywords={gait analysis, wearable computing, fall detection, gait model}, doi={10.4108/eai.28-9-2015.2261519} }
- Dong Qin
Ming-Chun Huang
Year: 2015
A smart phone based gait monitor system
PHAT
EAI
DOI: 10.4108/eai.28-9-2015.2261519
Abstract
Gait is a person’s manner of walking. Analysis of gait can be used in many areas like healthcare, therapy, sports training and characteristic recognition. The goal of this paper is to present a smart phone based system to collect and calculate gait parameters. These parameters which consists of steps, step length, velocity, cadence, motion intensity and walking regularity were collected by the inertial sensor in the smartphone. A prototype of gait parameter collection and visualization system has been built to collect accelerometer data from the smartphone, providing a reliable algorithm to calculate several gait parameters closely related to walking activity. The system also contains a fall detection function. Once the user suffers from fall, an alarm message will be send to another. Experiment has been done on 4 subjects for testing the stability and accuracy of the system. The experiment result has been compared with the real data. It shows a high accuracy and reliability for counting steps (error<5.47%) and walking duration (error<4.55%). Based on the gait monitor system, an anomaly data detection method is presented. Four independent gait parameters (cadence and motion intensity in three axis) are chosen from previous results during normal activity and their mean and standard deviation are calculated individually. If the latest data deviate from the normal activity model too far, this data is defined as an abnormal event.
Copyright © 2015 M.-C. Huang and D. Qin, licensed to EAI. This is an open access article distributed under the terms of the Creative Commons Attribution licence (http://creativecommons.org/licenses/by/3.0/), which permits unlimited use, distribution and reproduction in any medium so long as the original work is properly cited.