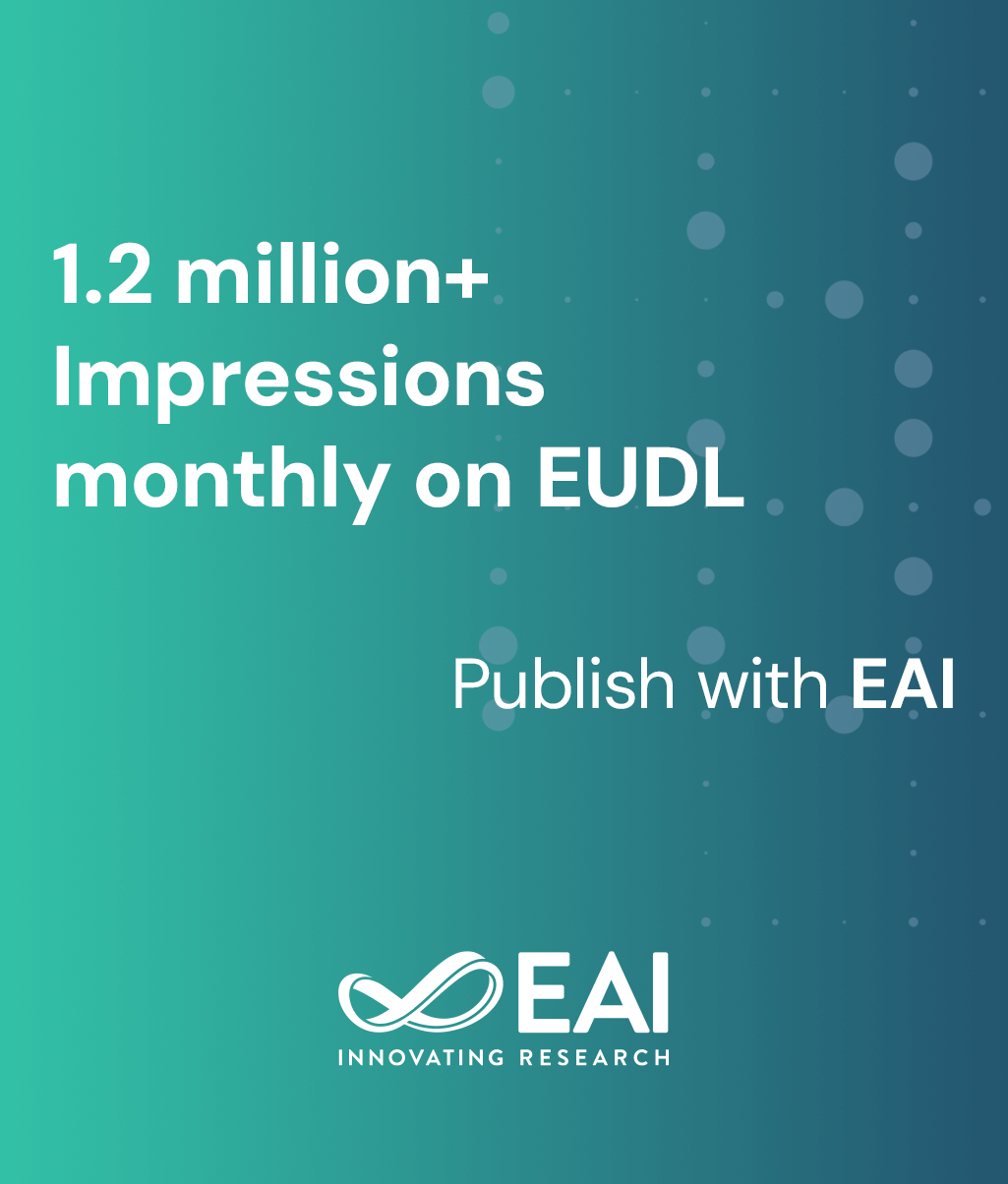
Research Article
Fall-MobileGuard: a Smart Real-Time Fall Detection System
@INPROCEEDINGS{10.4108/eai.28-9-2015.2261462, author={Giancarlo Fortino and Raffaele Gravina}, title={Fall-MobileGuard: a Smart Real-Time Fall Detection System}, proceedings={10th EAI International Conference on Body Area Networks}, publisher={ACM}, proceedings_a={BODYNETS}, year={2015}, month={12}, keywords={fall detection wearable sensors clound computing bodycloud}, doi={10.4108/eai.28-9-2015.2261462} }
- Giancarlo Fortino
Raffaele Gravina
Year: 2015
Fall-MobileGuard: a Smart Real-Time Fall Detection System
BODYNETS
ICST
DOI: 10.4108/eai.28-9-2015.2261462
Abstract
This paper proposes Fall-MobileGuard, a novel real-time non-invasive fall detection and alarm notification system. The proposed system, in particular, is able to recognize different types of falls and is based on a wearable inertial sensor node, equipped with a tri-axial accelerometer, worn at the waist and a personal mobile device. The detection method consists of two main processing blocks; the first is threshold-based trigger and is executed on the wearable sensor while the second includes posture classification and operates on the mobile device. Conversely to previous literature, we introduced multiple severity levels of detected fall events. The system is primarily intended for elderly people living alone or in retirements homes and supports alarm notifications of fall events over diversified channels, including social networks and automatic voice calls, according to the severity of the event. A comprehensive and well defined experimentation has been conducted, collecting in semi-controlled environment acceleration data from twenty subjects emulating falls and performing everyday life activities. Performance evaluation of our system has been carried out, resulting in 97% specificity, 83% sensitivity and 90% precision.