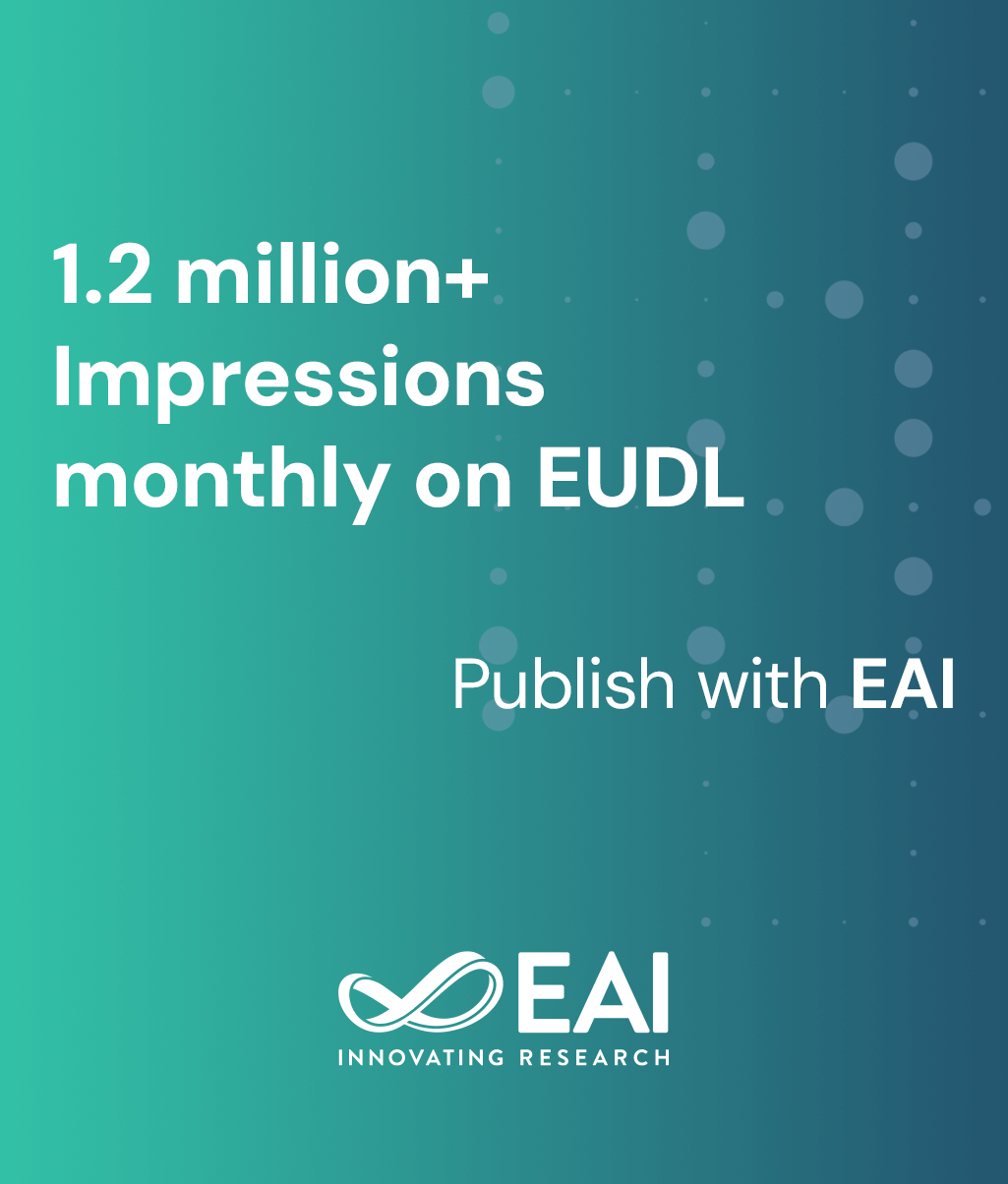
Research Article
Diagnosing Bipolar Disorders in a Wearable Device
@ARTICLE{10.4108/eai.28-9-2015.2261428, author={Chao Gui and Jie Zhu}, title={Diagnosing Bipolar Disorders in a Wearable Device}, journal={EAI Endorsed Transactions on Ambient Systems}, volume={3}, number={11}, publisher={ACM}, journal_a={AMSYS}, year={2015}, month={12}, keywords={bipolar disorder, support vector machine (svm), gaussian mixture model (gmm), wearable device}, doi={10.4108/eai.28-9-2015.2261428} }
- Chao Gui
Jie Zhu
Year: 2015
Diagnosing Bipolar Disorders in a Wearable Device
AMSYS
EAI
DOI: 10.4108/eai.28-9-2015.2261428
Abstract
Bipolar disorder is a common chronic recurrent psychosis and it mainly relies on doctors’ experience to determine the patient’s condition currently. We aimed to find a useful methodology to diagnose the mental state and guide medical treatment by using speech signal processing. Methods: Firstly, the feature classes were extracted (e.g., Pitch, Formant, MFCC, GT). Secondly, class separability criterion based on distance (the Between-class Variance and Within-class Variance) was adopted as an evaluation criteria to get the features assessment, and then, we found LPC played a core role on the all features. According to the experiment, the SVM have a good performance for the single patient up to 90%, and the GMM classifier yields the best performance with a classification rate of 72% for multi patients. The newly proposed methodology provide a good method for helping diagnose bipolar disorder.
Copyright © 2015 J. Zhu and C. Gui, licensed to EAI. This is an open access article distributed under the terms of the Creative Commons Attribution licence (http://creativecommons.org/licenses/by/3.0/), which permits unlimited use, distribution and reproduction in any medium so long as the original work is properly cited.