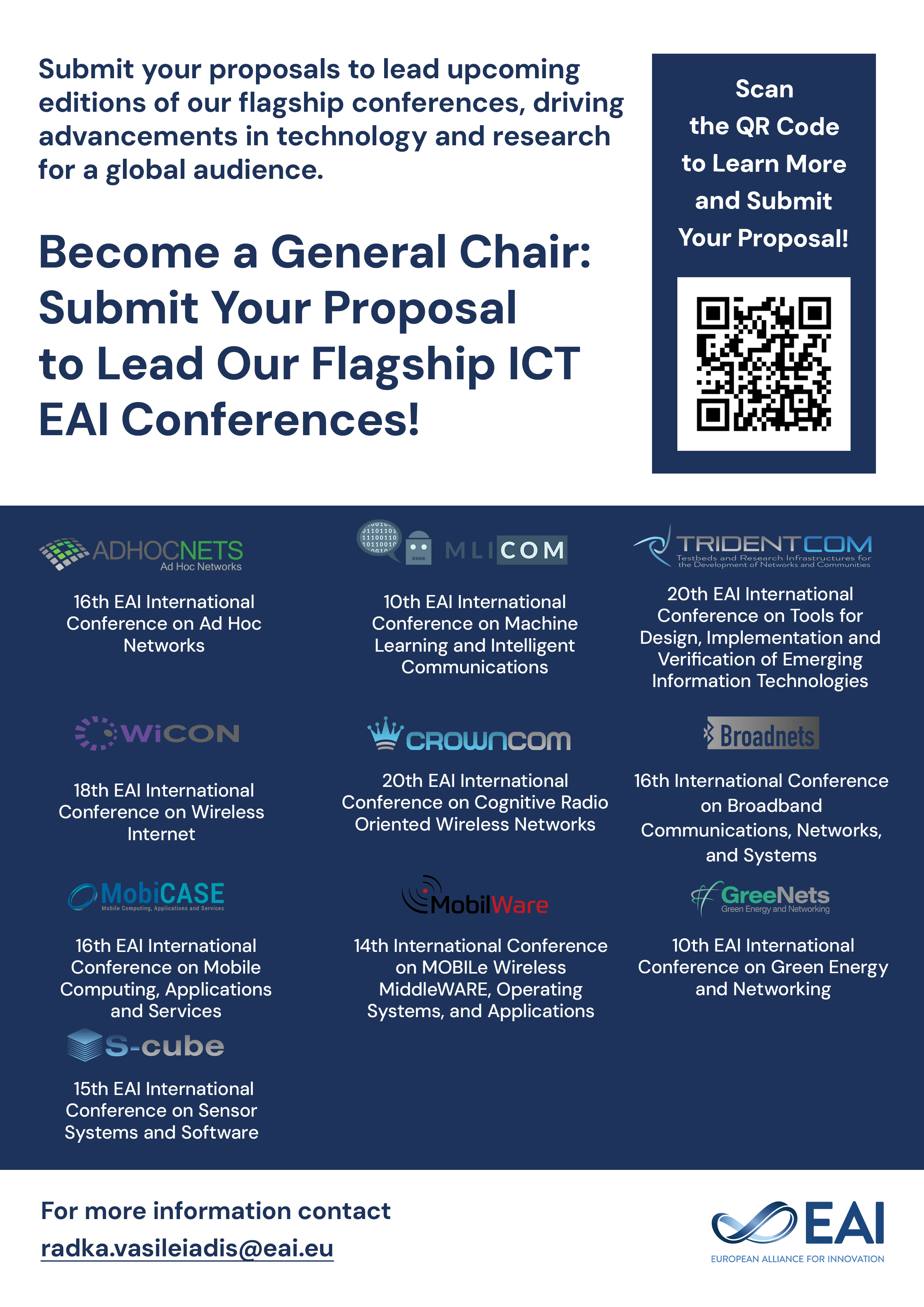
Research Article
Evaluation of Customer Behaviour with Machine Learning for Churn Prediction: The Case of Bank Customer Churn in Europe
@INPROCEEDINGS{10.4108/eai.28-10-2022.2328450, author={Pei Chen and Nian Liu and Binrui Wang}, title={Evaluation of Customer Behaviour with Machine Learning for Churn Prediction: The Case of Bank Customer Churn in Europe}, proceedings={Proceedings of the International Conference on Financial Innovation, FinTech and Information Technology, FFIT 2022, October 28-30, 2022, Shenzhen, China}, publisher={EAI}, proceedings_a={FFIT}, year={2023}, month={4}, keywords={machine learning; customer churn prediction; logistic regression; adaboost}, doi={10.4108/eai.28-10-2022.2328450} }
- Pei Chen
Nian Liu
Binrui Wang
Year: 2023
Evaluation of Customer Behaviour with Machine Learning for Churn Prediction: The Case of Bank Customer Churn in Europe
FFIT
EAI
DOI: 10.4108/eai.28-10-2022.2328450
Abstract
The way to extract valuable information from users' information data as well as provide correct and effective financial services is a topic worthy of attention. Machine learning scenarios are widely adopted to predict user churn, implanting corresponding marketing methods, and formulating appropriate retention measures is being applied by more and more companies to reduce customer churn. In this paper, the logistic regression classifier is chosen as the baseline model to predict customer churn based on 10,000 customer information of a European bank. Based on the SMOTE oversampling method to balance the data set and other processes, five models of logistic regression, SVC, GBDT, random forest and AdaBoost are finally trained. Thereinto, this paper only performs grid search on the four models except the baseline model to optimize the model parameters. After the evaluation through recall, precision and AUC scores, AdaBoost classifier is finally selected as the prediction model for this case, with recall and AUC score being 0.718 and 0.776, respectively. These results shed light on guiding further exploration of the prediction of bank customer churn.