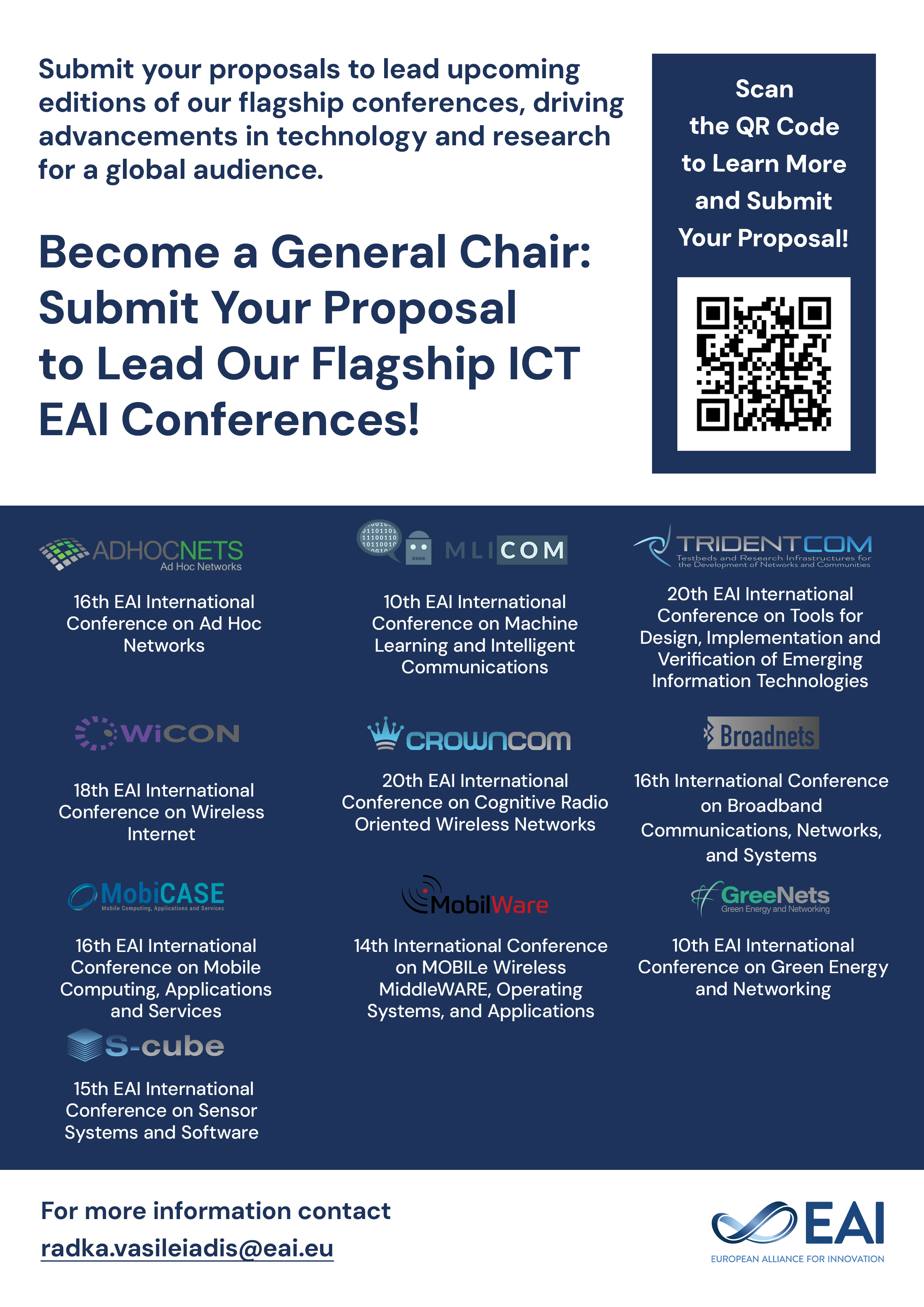
Research Article
Long Short-term Memory Neural Network Model for Stock Prediction under COVID-19 Pandemic
@INPROCEEDINGS{10.4108/eai.28-10-2022.2328444, author={Cheng Gao}, title={Long Short-term Memory Neural Network Model for Stock Prediction under COVID-19 Pandemic}, proceedings={Proceedings of the International Conference on Financial Innovation, FinTech and Information Technology, FFIT 2022, October 28-30, 2022, Shenzhen, China}, publisher={EAI}, proceedings_a={FFIT}, year={2023}, month={4}, keywords={stock price prediction; lstm model; pandemic}, doi={10.4108/eai.28-10-2022.2328444} }
- Cheng Gao
Year: 2023
Long Short-term Memory Neural Network Model for Stock Prediction under COVID-19 Pandemic
FFIT
EAI
DOI: 10.4108/eai.28-10-2022.2328444
Abstract
Due to disasters like the COVID-19 pandemic, the stock markets became more volatile than before. To avoid significant losses, it is important for investors to accurately predict big variations in stock prices under the impact of the pandemic through financial modeling. In this paper, an advanced model, the Long Short-term Memory Model (LSTM) was introduced, which makes a more accurate prediction than the previous technical-based models (e.g. ARIMA) under the current circumstance. The study implemented the daily stock price from three technological firms. The dataset was divided into two parts. One was used for training and optimizing the model, while the other was for testing the accuracy of the model. With the training dataset, the model began memorizing the important features within the sequential data, which are shown as the updating cell state and hidden state within the model. After the optimization, it generated the predicted value using the testing dataset and compared it with the real value to present its accuracy. Experimental results indicated that the LSTM model achieved the purpose of forecasting every significant variation in stock price trends, although the trends are unstable under the influence of the pandemic.