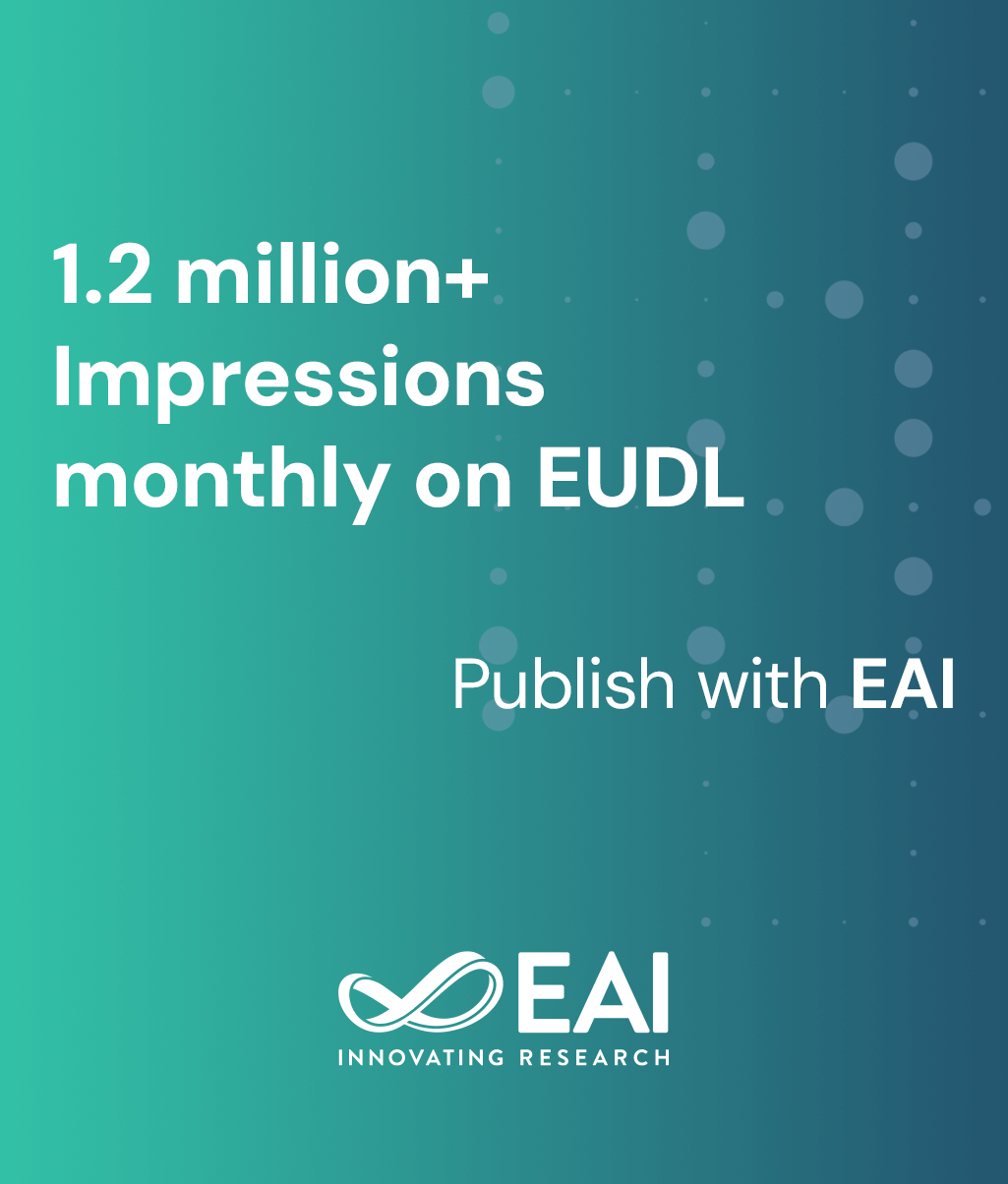
Research Article
Multifactorial Linear Model for Crptocurrency Prediction: OLS and Lasso
@INPROCEEDINGS{10.4108/eai.28-10-2022.2328414, author={Haoyuan Ma}, title={Multifactorial Linear Model for Crptocurrency Prediction: OLS and Lasso}, proceedings={Proceedings of the International Conference on Financial Innovation, FinTech and Information Technology, FFIT 2022, October 28-30, 2022, Shenzhen, China}, publisher={EAI}, proceedings_a={FFIT}, year={2023}, month={4}, keywords={cryptocurrency; machine learning; price forcasting; ols; lasso}, doi={10.4108/eai.28-10-2022.2328414} }
- Haoyuan Ma
Year: 2023
Multifactorial Linear Model for Crptocurrency Prediction: OLS and Lasso
FFIT
EAI
DOI: 10.4108/eai.28-10-2022.2328414
Abstract
Price forecasting is pretty crucial in the asset management and allocation and quantitative trading industries. With the development of the global economic situation, decentralized finance has gradually entered people's field of vision, and cryptocurrency and cryptocurrency finance have become the research fields of a large number of scientists and practitioners. This article will use two traditional and basic machine learning models to predict the price of cryptocurrencies. Primarily, the tick data and order book information including the price and trading volume of cryptocurrencies will be obtained through OKEX. Subsequently, 16 common technical indicators will be used for stock trading to build OLS and Lasso models. The model is trained on the first part of the data, and finally the model is made to make predictions about future prices and compare it with the second half of the data, which is the true price. According to the evaluations, when the price is in the middle range, the accuracy of the model is relatively high, and it can also follow the market trend well. However, when the price fluctuates, the model predicts the result to be extreme than the reality, i.e., higher than the price above the median price, lower than the price below the median price, and the overall volatility is greater. These results shed light on guiding further exploration of cryptocurrency pricing forecasting.