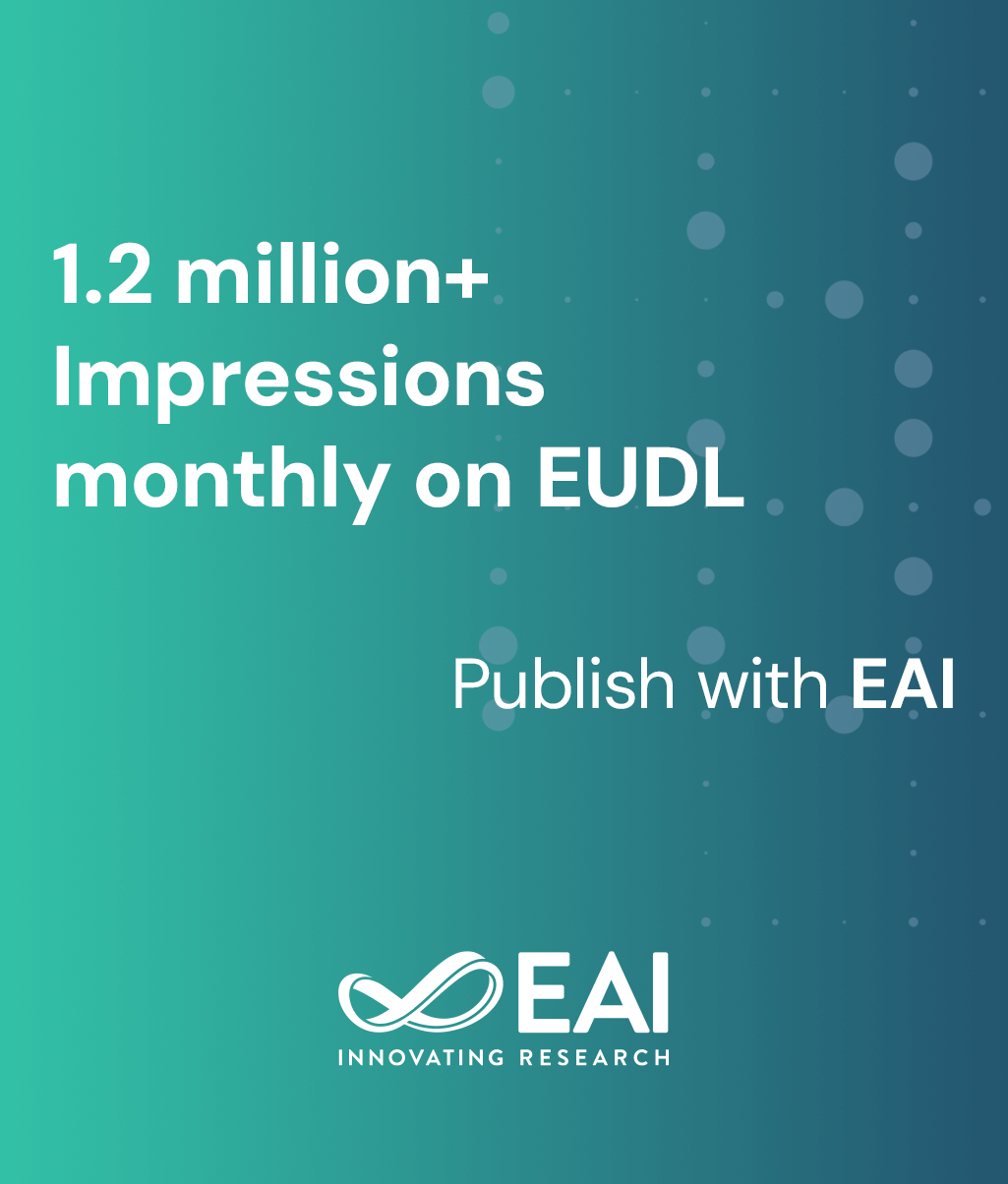
Research Article
Comparing the Efficiency of Stock and Index Price Prediction Between FNN and LSTM Scenarios
@INPROCEEDINGS{10.4108/eai.28-10-2022.2328401, author={Yigong Yuan}, title={Comparing the Efficiency of Stock and Index Price Prediction Between FNN and LSTM Scenarios}, proceedings={Proceedings of the International Conference on Financial Innovation, FinTech and Information Technology, FFIT 2022, October 28-30, 2022, Shenzhen, China}, publisher={EAI}, proceedings_a={FFIT}, year={2023}, month={4}, keywords={fnn; lstm; hongkong stock; internet industry}, doi={10.4108/eai.28-10-2022.2328401} }
- Yigong Yuan
Year: 2023
Comparing the Efficiency of Stock and Index Price Prediction Between FNN and LSTM Scenarios
FFIT
EAI
DOI: 10.4108/eai.28-10-2022.2328401
Abstract
Predicting the trend of changes in stock prices serves as a crucial role in the quantitative investment industry, and few previous empirical analysis studies choose the underlying assets from Hong Kong Stock Exchange. This study compares the performance of two machine learning approaches (i.e., FNN and LSTM) in the interpretation accuracy of two Hong Kong Internet technology industry companies and two related market indices, for the sake of exploring a better way to explain the Hong Kong stock price movement. Ten technical analysis factors were selected as inputs to the model. According to the analysis, after selecting the best model parameters and training times, both FNN and LSTM models have high accuracy in predicting Hong Kong stock data (82.76% and 90.85% respectively). In comparison, LSTM is more suitable for analyzing Hong Kong Internet technology Model selection for stock movement trends. These results shed light on guiding further exploration of stock price prediction in terms of the state-of-art neural network techniques.