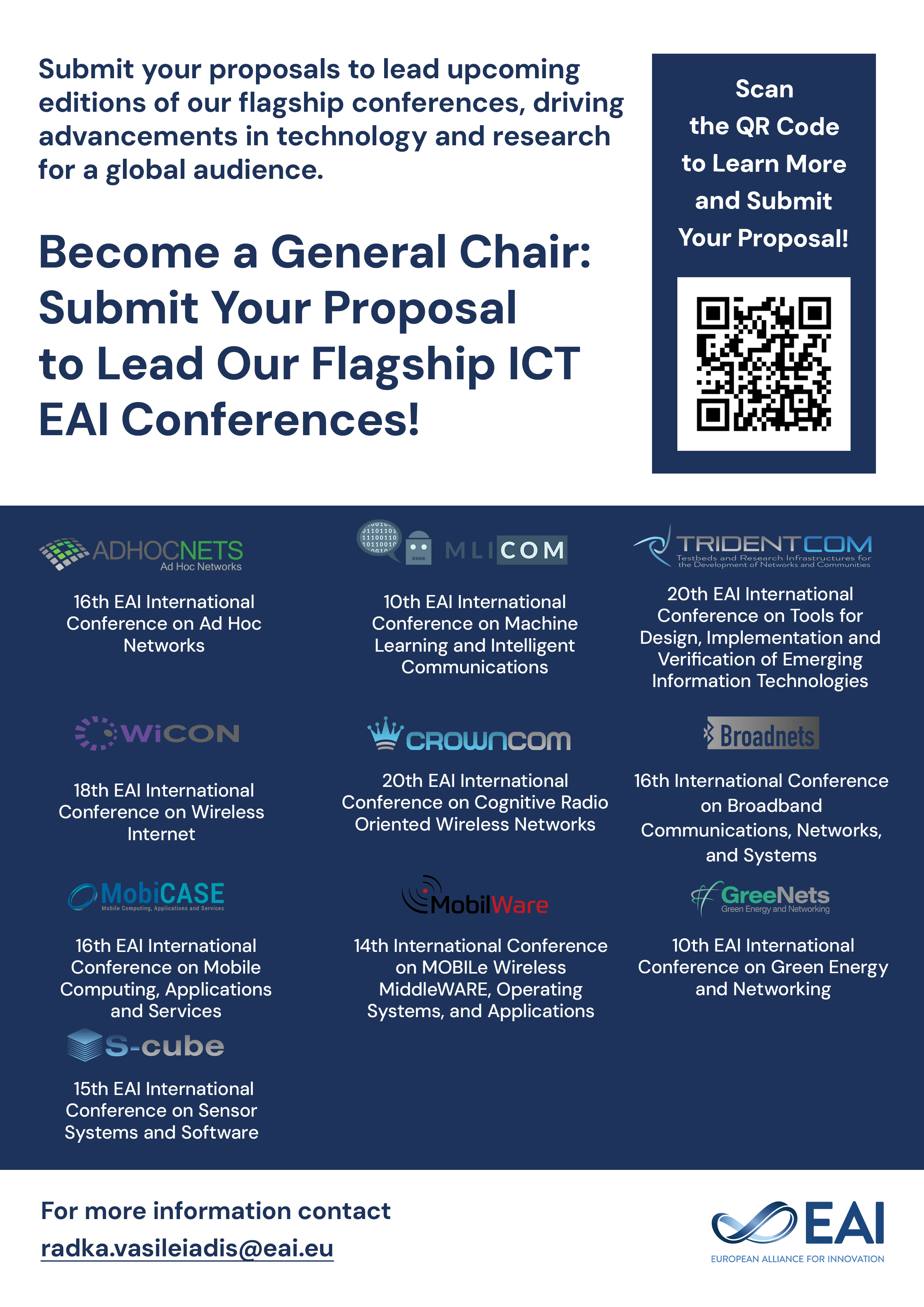
Research Article
Heterogeneous wireless sensor network routing protocol for an adaptive gray wolf optimizer
@INPROCEEDINGS{10.4108/eai.27-8-2020.2295028, author={Chao Cheng and QingShan Han and Guoli Cheng and Shuang Zhai}, title={Heterogeneous wireless sensor network routing protocol for an adaptive gray wolf optimizer}, proceedings={Proceedings of the 13th EAI International Conference on Mobile Multimedia Communications, Mobimedia 2020, 27-28 August 2020, Cyberspace}, publisher={EAI}, proceedings_a={MOBIMEDIA}, year={2020}, month={11}, keywords={energy efficient; gray wolf optimizer (gwo); balanced cluster structure; wireless sensor network}, doi={10.4108/eai.27-8-2020.2295028} }
- Chao Cheng
QingShan Han
Guoli Cheng
Shuang Zhai
Year: 2020
Heterogeneous wireless sensor network routing protocol for an adaptive gray wolf optimizer
MOBIMEDIA
EAI
DOI: 10.4108/eai.27-8-2020.2295028
Abstract
Wireless sensor network (WSN), plays an increasingly important role in information collection. In this paper, firstly, in order to adapt to the actual conditions, the communication process of the nodes energy is limited, and a three-stage energy heterogeneous network model is designed. Secondly, for the convergence node frequent task forwarding and complex cluster first-round energy consumption, by combining the optimal number of cluster heads with the gray wolf optimization algorithm, a new fitness function is designed that integrates the remaining energy of the nodes and the distance from the nodes to the base station. In addition, an improved iterative factor is introduced to enhance the ability of local search in cluster head selection, so as to improve the accuracy of cluster head search. Finally, the simulation results show that the proposed method extends the lifetime of the network 50%, reduces the process of energy consumption, and improves the throughput of network data 30%.