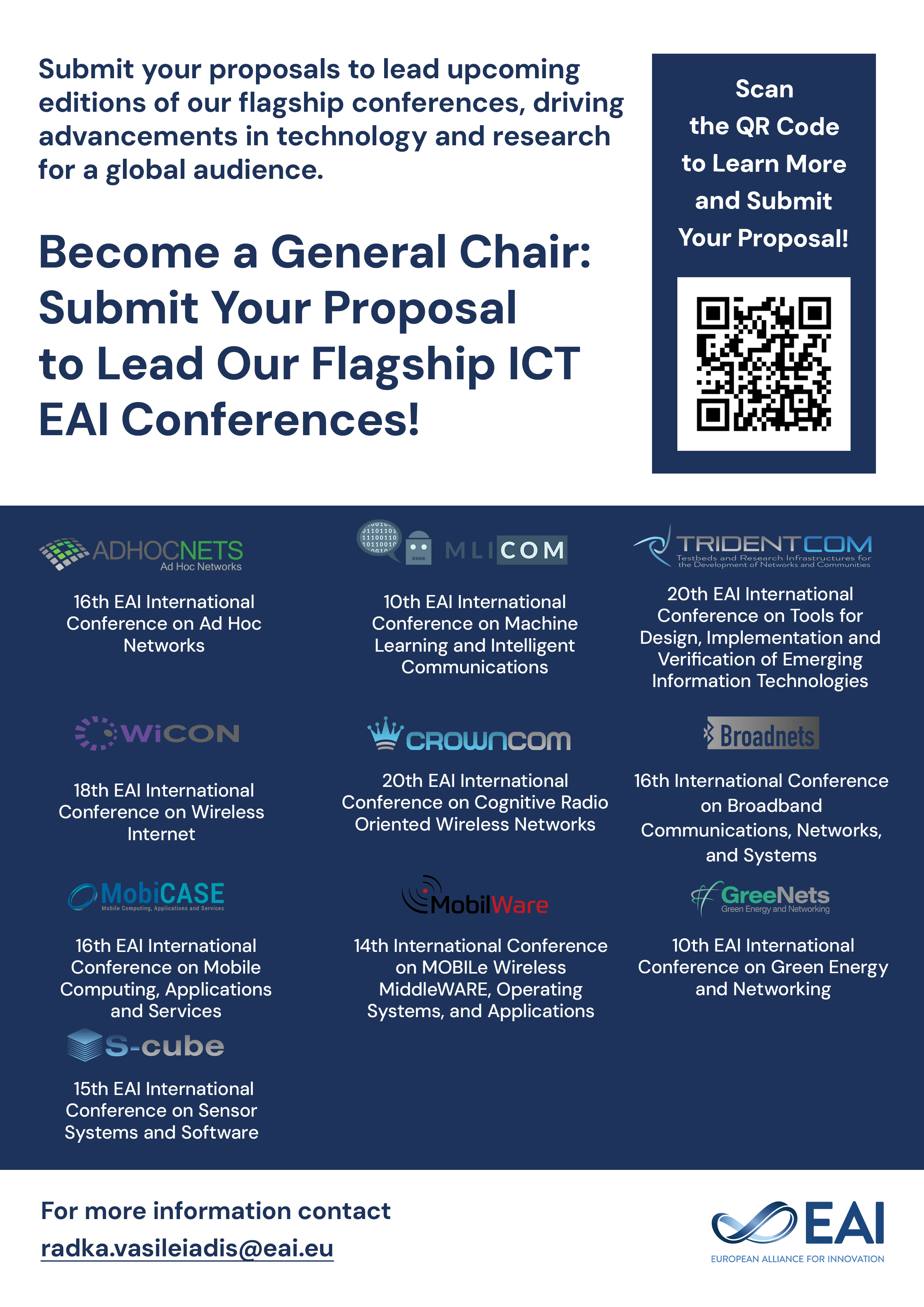
Research Article
Feature Fusion Convolutional Network Based Automatic Modulation Classification
@INPROCEEDINGS{10.4108/eai.27-8-2020.2294995, author={Jiajie Mai and Shanchuan Ying and Nanxin Wang and Sai Huang}, title={Feature Fusion Convolutional Network Based Automatic Modulation Classification}, proceedings={Proceedings of the 13th EAI International Conference on Mobile Multimedia Communications, Mobimedia 2020, 27-28 August 2020, Cyberspace}, publisher={EAI}, proceedings_a={MOBIMEDIA}, year={2020}, month={11}, keywords={automatic modulation classification residual neural network constellation cyclic spectrum density graph}, doi={10.4108/eai.27-8-2020.2294995} }
- Jiajie Mai
Shanchuan Ying
Nanxin Wang
Sai Huang
Year: 2020
Feature Fusion Convolutional Network Based Automatic Modulation Classification
MOBIMEDIA
EAI
DOI: 10.4108/eai.27-8-2020.2294995
Abstract
Automatic modulation classification (AMC) technology, which utilizes to classify different kinds of signals using various expert features, typically the constellation map and the cyclic spectrum density graph, plays a significant role in spectrum monitoring and radio communication. However, due to their own disadvantages of constellation and cyclic spectrum density when recognizing some certain signals, it is necessary to combine them together in AMC. In this paper aiming at the commonly used features-based (FB) approach in practice, we propose a novel AMC model which jointly utilizes constellation map and cyclic spectrum density graph as the signal features. In order to provide a solution for the model, we propose a feature-based and supervised network in Single in Single out (SISO) system to identify seven kinds of signals, which is called Feature Fusion Convolutional Network (FFCN). By employing modified residual neural network (ResNet), the network can accomplish the prediction and classification according to the normalized and combined input from both two approaches. Simulation results are provided to show that FFCN can classify different types of signals with high probability as well as maintain the splendid efficiency compared to the benchmarks. Typically when signal to noise ratio (SNR) is low, the success rate of the proposed network is 2% higher at average, which proves that our network has better performance.