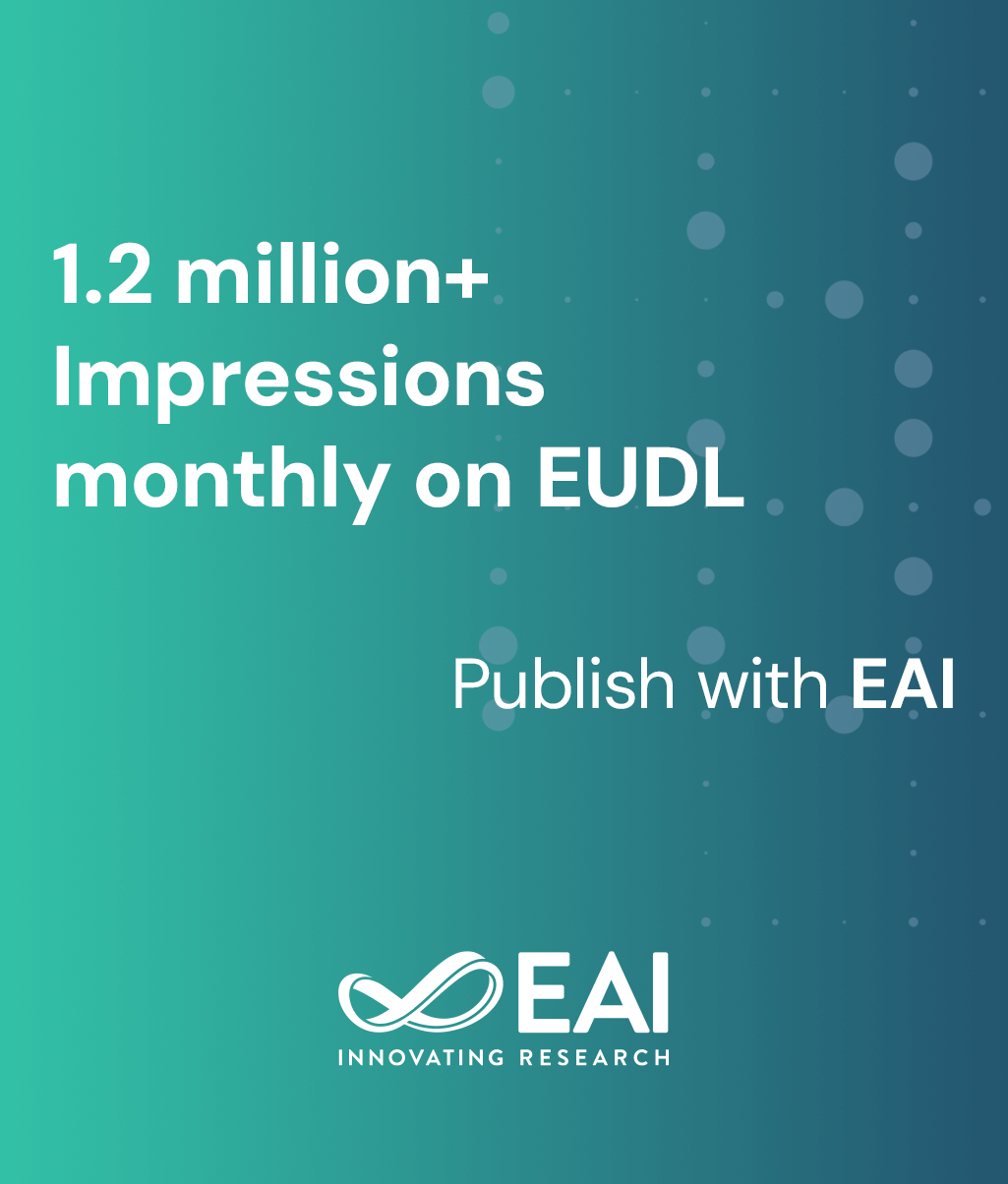
Research Article
Transfer Learning Based Screen Defect Classification
@INPROCEEDINGS{10.4108/eai.27-8-2020.2294670, author={Li Yilei and Li Chengyuan and Zhang Yifan and Chang Shuo and Zhang Fan and wang zixuan}, title={Transfer Learning Based Screen Defect Classification}, proceedings={Proceedings of the 13th EAI International Conference on Mobile Multimedia Communications, Mobimedia 2020, 27-28 August 2020, Cyberspace}, publisher={EAI}, proceedings_a={MOBIMEDIA}, year={2020}, month={11}, keywords={defect classification transfer learning cnn generation module}, doi={10.4108/eai.27-8-2020.2294670} }
- Li Yilei
Li Chengyuan
Zhang Yifan
Chang Shuo
Zhang Fan
wang zixuan
Year: 2020
Transfer Learning Based Screen Defect Classification
MOBIMEDIA
EAI
DOI: 10.4108/eai.27-8-2020.2294670
Abstract
For the screen defect classification, human inspectors and traditional machine learning algorithms are inefficient and inaccurate. Convolutional neural network (CNN) driven by data are feasible solutions. However, real training images are limited in the industrial scenario, which causes overfitting. Hence, in this paper, a novel learning based method is proposed for defect classification, which is based on the CNN. To alleviate the problem of limited images, two strategies are introduced into model learning. For the training data, a data generation module is implemented to enlarge the training dataset. For the CNN model learning, transfer learning is applied in the whole training process. To verify the proposed method, various experiments are carried out. The results indicate that our screen defect classification model achieves superior performance.