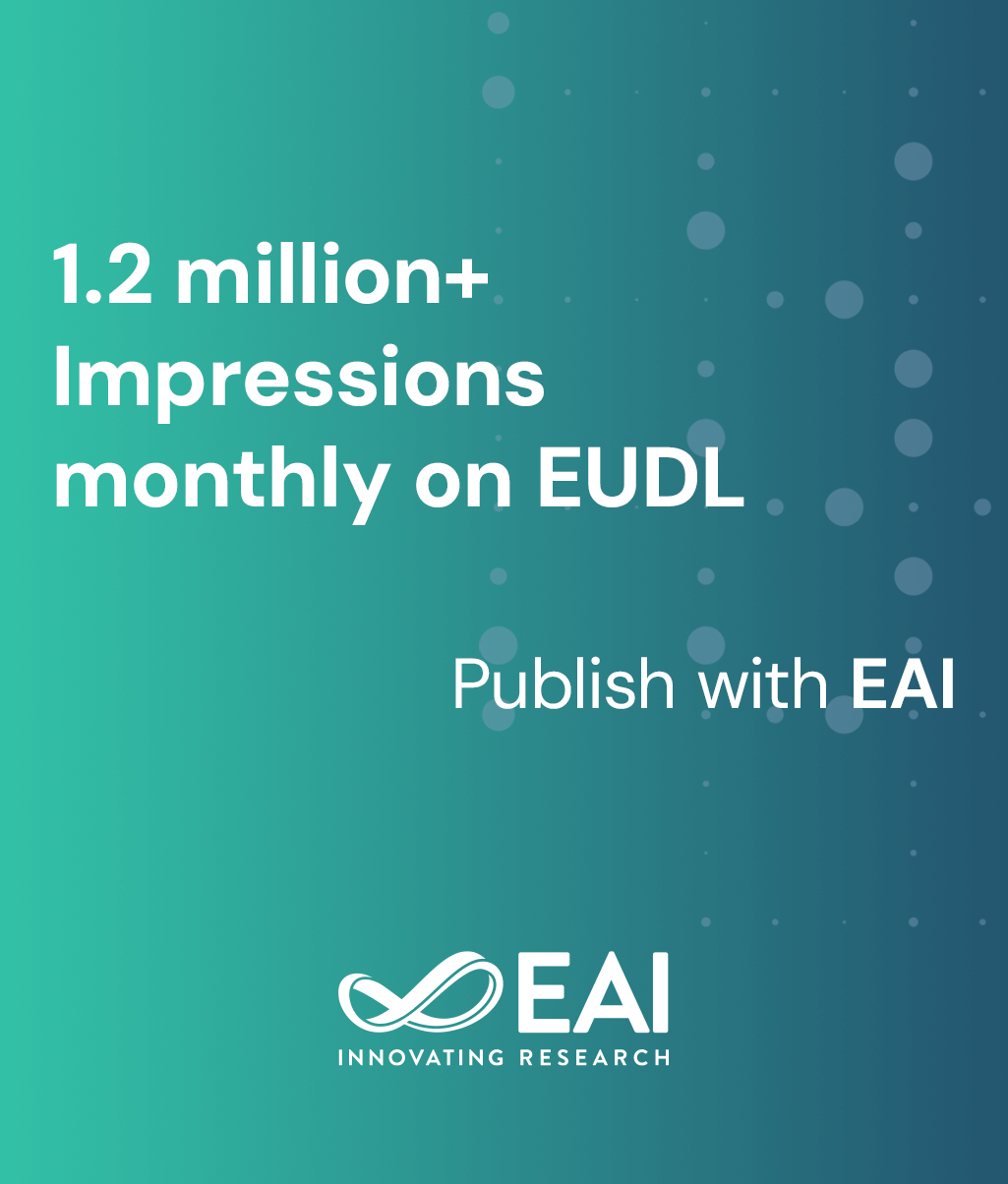
Research Article
IoT Based Detection of Molded Bread and Expiry Prediction using Machine Learning Techniques
@ARTICLE{10.4108/eai.27-4-2022.173972, author={Muhammad Shoaib Akhtar and Tao Feng}, title={IoT Based Detection of Molded Bread and Expiry Prediction using Machine Learning Techniques}, journal={EAI Endorsed Transactions on Creative Technologies}, volume={9}, number={31}, publisher={EAI}, journal_a={CT}, year={2022}, month={4}, keywords={IoT, ML, SVM}, doi={10.4108/eai.27-4-2022.173972} }
- Muhammad Shoaib Akhtar
Tao Feng
Year: 2022
IoT Based Detection of Molded Bread and Expiry Prediction using Machine Learning Techniques
CT
EAI
DOI: 10.4108/eai.27-4-2022.173972
Abstract
Expiration of a bread is a very popular issue in food logistics. Due to various conditions fungal bread can cause food poisoning for consumers. As a result, nausea, diarrhea and different medical issues appear in people. For this purpose, an intelligent system required for the detection of present condition of bread is required which will help the stores and consumers. In this study, we have developed a prototype made up of Arduino Nano as a microcontroller, MQ series sensors for CO and CO2 detection in shopper bags of bread in order to collect data. This data is further processed in different machine learning algorithms for the detection of current condition of bread in these stores. The data collected from these sensors was imbalanced. Data collected from sensors is then balanced by using SMOTE and TOMEC Links (data balancing techniques). Furthermore, data preprocessing and feature engineering has been applied on IoT Based dataset to improve its efficiency. We have applied linear learning models for the prediction of current condition of bread. Within linear models, Gaussian Naïve Bayes has scored highest accuracy of 81.54%.
Copyright © 2022 Muhammad Shoaib Akhtar et al., licensed to EAI. This is an open access article distributed under the terms of the Creative Commons Attribution license, which permits unlimited use, distribution and reproduction in any medium so long as the original work is properly cited.