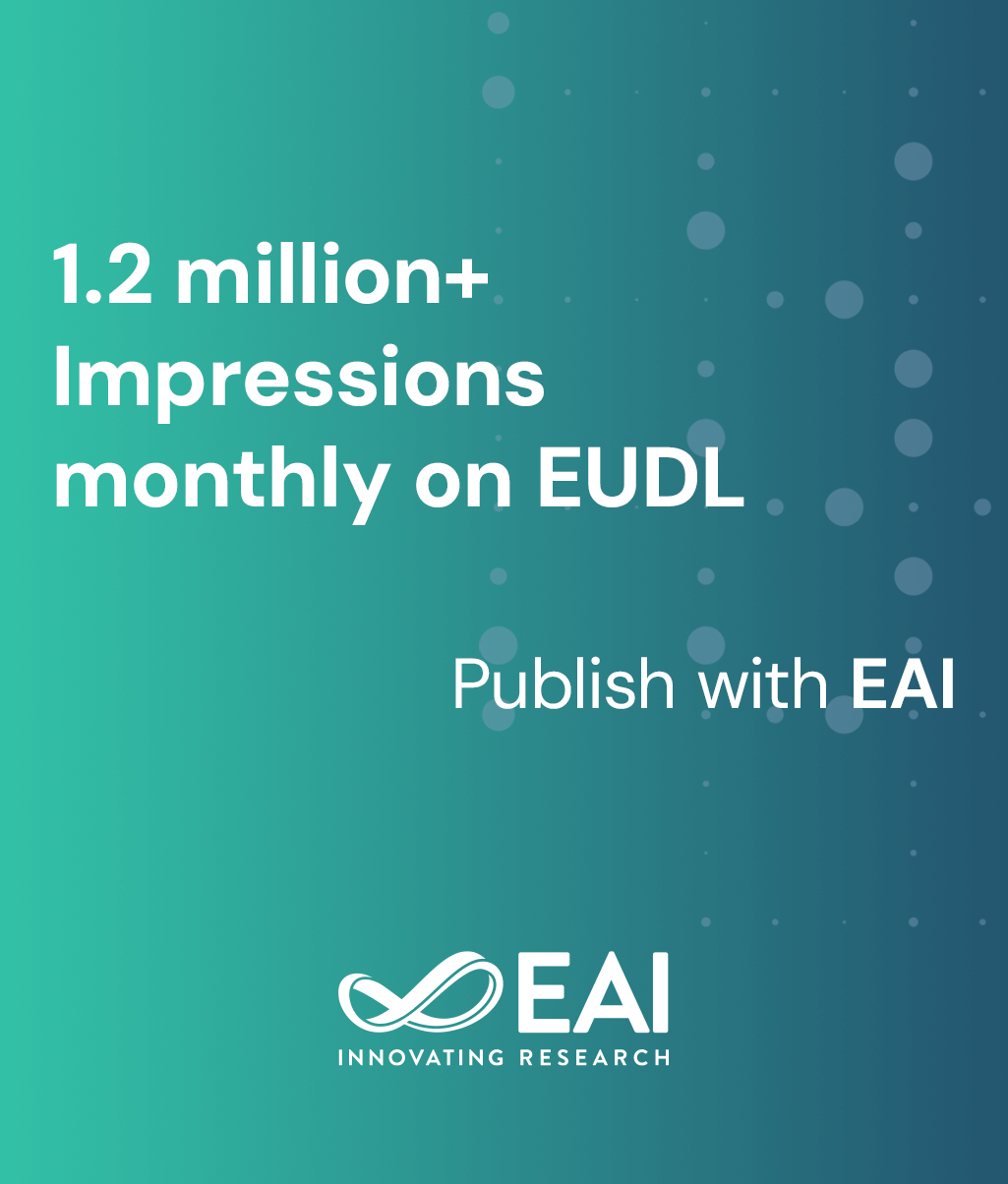
Research Article
Logistic Regression Based Classification of Spam and Non-Spam Emails
@INPROCEEDINGS{10.4108/eai.27-2-2020.2303291, author={Shahbaz Ahmad Khanday and Suraiya Parveen}, title={Logistic Regression Based Classification of Spam and Non-Spam Emails}, proceedings={Proceedings of the 2nd International Conference on ICT for Digital, Smart, and Sustainable Development, ICIDSSD 2020, 27-28 February 2020, Jamia Hamdard, New Delhi, India}, publisher={EAI}, proceedings_a={ICIDSSD}, year={2021}, month={3}, keywords={machine learning decision tree support vector machine (svm) logistic regression artificial neural networks naive bayesian classifier and spam-bots}, doi={10.4108/eai.27-2-2020.2303291} }
- Shahbaz Ahmad Khanday
Suraiya Parveen
Year: 2021
Logistic Regression Based Classification of Spam and Non-Spam Emails
ICIDSSD
EAI
DOI: 10.4108/eai.27-2-2020.2303291
Abstract
An email client receives emails from different websites, portals and domains, which can be an advertisement. Receiving a bulk amount of emails can cause serious damages like suspension of a particular email id. Mostly an email client gets exposed to the number of malicious receipts by registering an email account to a web portal, which in turn sends a bulk amount of emails. One of the solutions to escape from spam emails is to develop a decision based system which can classify the spam and non-spam emails. This can be achieved using different machine learning and deep learning and deep learning algorithms to classify the spam and non-spam emails by accessing the received emails of an email client. The machine learning approaches and mechanisms like SVM, naive Bayesian classifier, artificial neural networks and random forests can be of important help to determine spam emails. After classifying a spam email source a user can navigate, block and report the source of the spam email generator like spam-bots